pyRBDome: A comprehensive computational platform for enhancing and interpreting RNA-binding proteome data
biorxiv(2023)
摘要
High-throughput proteomics approaches have revolutionised the identification of RNA-binding proteins (RBPome) and RNA-binding sequences (RBDome) across organisms. Many novel putative RNA-binding proteins (RBPs) were discovered, including those that lack recognisable RNA-binding domains. Yet the extent of noise, including false-positive identifications, associated with these methodologies is difficult to quantify as experimental approaches for validating the results are generally low throughput. To address this, we introduce pyRBDome, a pipeline for in-depth in silico enhancement of RNA-binding proteome data. It does so by comparing experimental results with RNA-binding site (RBS) predictions from several distinct machine learning tools and integrates high-resolution structural data of protein-RNA complexes when available. By providing a statistical evaluation of RBDome data, users can rapidly identify protein sequences from RBDome experiments most likely to be bona fide RNA-binders. Furthermore, by leveraging the predictions collated by pyRBDome, we have enhanced the sensitivity and specificity of RBS detection through training new ensemble machine learning models. We describe a pyRBDome analysis of a large human RBDome dataset and conducted a comparision with know structural data. These analyses reinforced the significance of stacking interactions in UV cross-linking protein-RNA interactions. Surprisingly, our analyses revealed two contrasting findings: While UV cross-linked amino acids were more likely to contain predicted RBSs, they infrequently bind RNA in high-resolution structures. Given the known limitations of structural data as benchmarks, these finding highlights the utility of pyRBDome as a valuable alternative approach for enhancing confidence in RBDome datasets. Finally, our comprehensive analysis of hundreds of (putative) RBPs offers a valuable resource for RBP enthusiasts.
### Competing Interest Statement
The authors have declared no competing interest.
更多查看译文
AI 理解论文
溯源树
样例
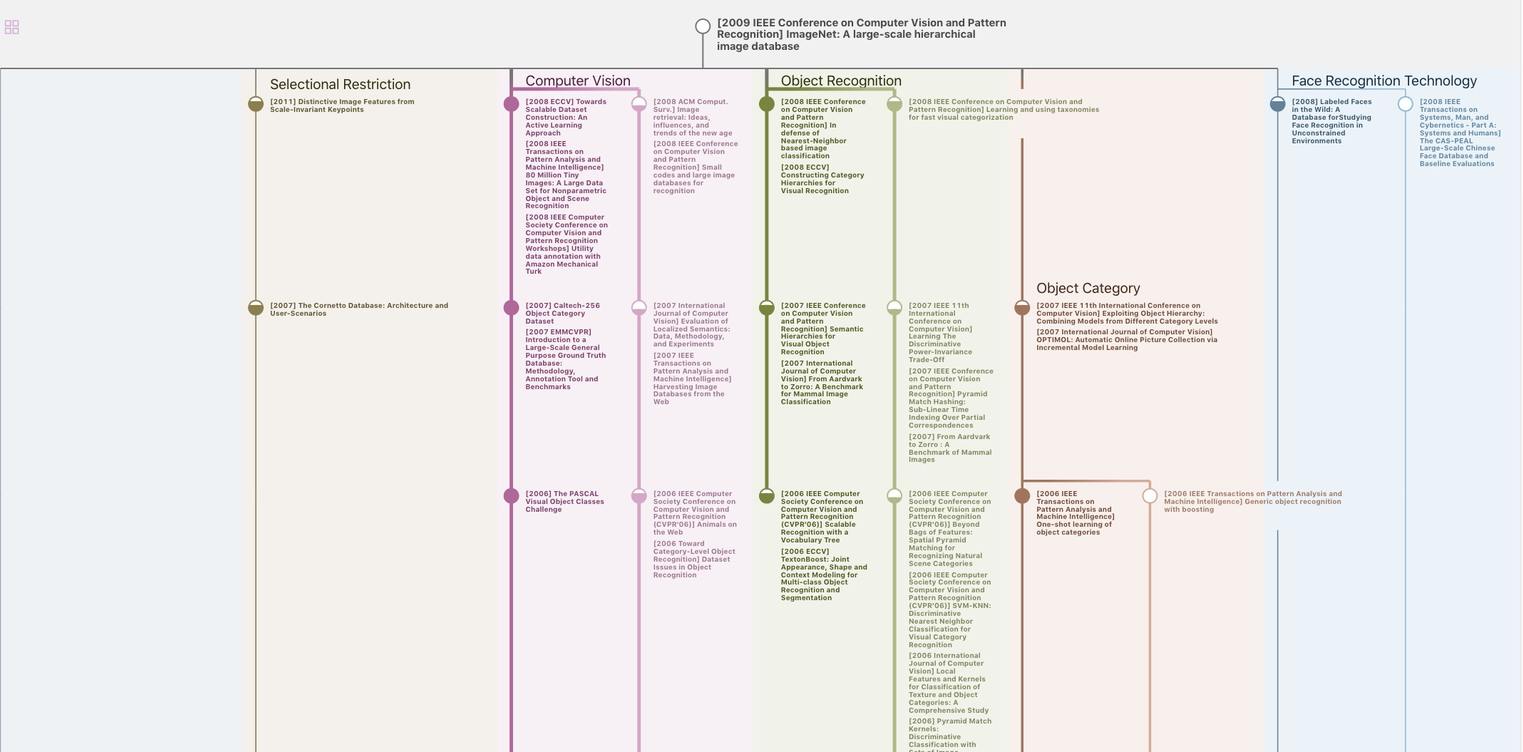
生成溯源树,研究论文发展脉络
Chat Paper
正在生成论文摘要