Underreporting in COVID-19 Case Data Causes Policy Objectives Being Unmet
IEEE TRANSACTIONS ON COMPUTATIONAL SOCIAL SYSTEMS(2023)
摘要
Since the start of the COVID-19 pandemic, social distancing has been key to curb the spread of severe acute respiratory syndrome coronavirus 2 (SARS-CoV-2). Social distancing policies have largely been made based on reported case data, which are often underreported. There is little to no understanding of how underreporting in case data may affect the performance of social distancing policies designed using such data. This article aims to fill this gap. We evaluate three policy-making rationales (called "controllers"): a flattening-the-curve (FC) controller that maximizes allowable transmission rate while ensuring hospital capacity is not exceeded, a time-limited-intervention (TLI) controller to minimize the number of infectious people with policies of fixed durations, and a minimizing-health-and-economic-costs-with-reinforcement-learning (MC-RL) controller to minimize weighted public health and economic costs. Each controller is applied in six scenarios where there is no underreporting, underreporting without correcting it, and underreporting with corrections. It is found that both the recommended policies by a controller and the resulting disease trajectories would be different under different scenarios, in which direction underreporting affects policies and disease trajectories depending on three factors: time elapsed since start of pandemic, local hospital capacity, and disease severity. Our findings demonstrate the high stakes of ignoring the underreporting issue or simply upscaling the case data: policies designed cannot achieve preset control objectives and could bring unexpected public health and economic outcomes.
更多查看译文
关键词
Bias correction,comparative analysis,controller,COVID-19,nonpharmaceutical interventions (NPIs),underreporting
AI 理解论文
溯源树
样例
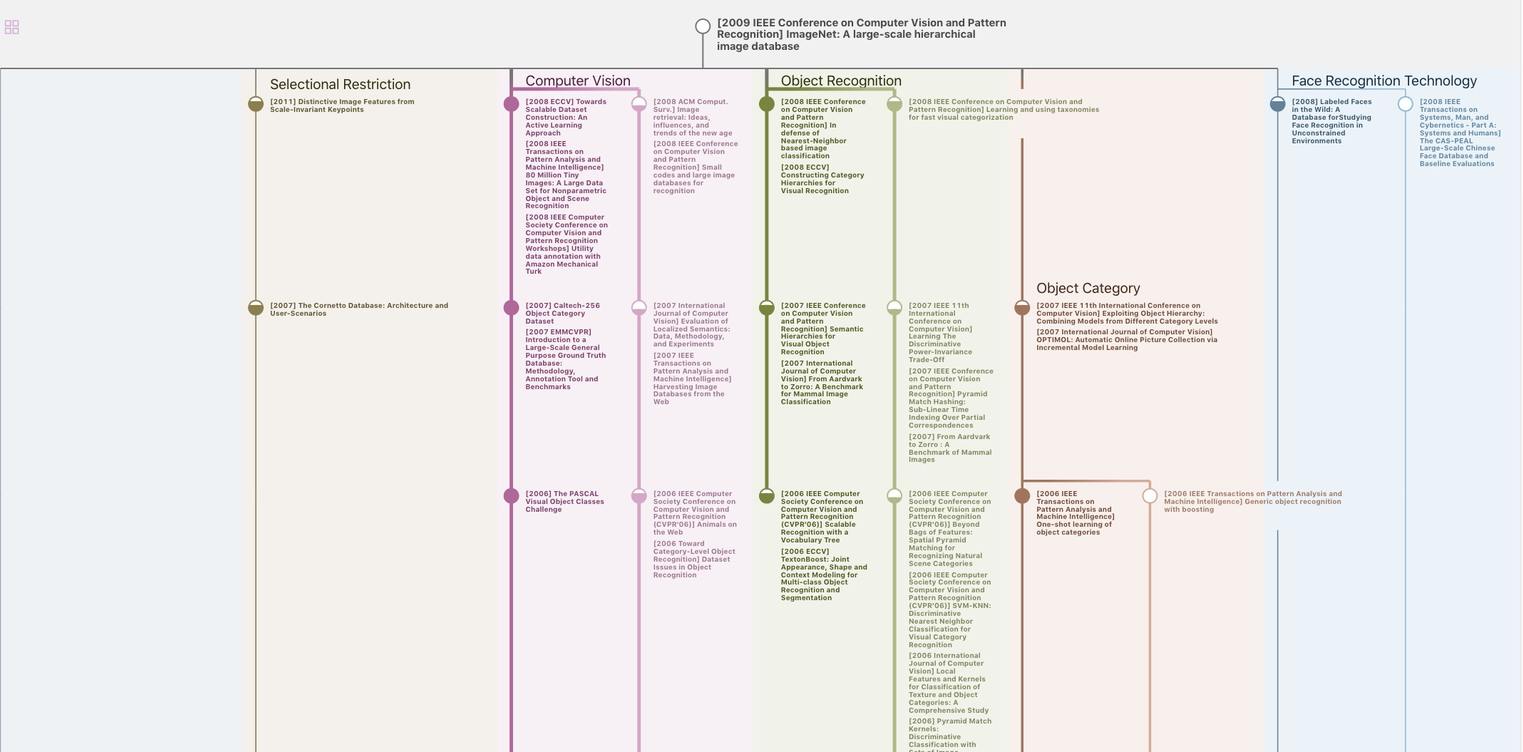
生成溯源树,研究论文发展脉络
Chat Paper
正在生成论文摘要