An application of 1D convolution and deep learning to remote sensing modelling of Secchi depth in the northern Adriatic Sea
BIG EARTH DATA(2024)
摘要
This paper presents a novel approach for predicting the water quality indicator - Secchi disk depth (ZSD). ZSD indirectly reflects water clarity and serves as a proxy for other quality parameters. This study utilizes Deep Neural Network (DNN) trained on satellite remote sensing and measured data from three sources: two datasets obtained from official agencies in Croatia and Slovenia, and one citizen science data source, all covering the northern coastal region of the Adriatic Sea. The proposed model uses 1D Convolutional Neural Network (CNN) in the spectral dimension to predict ZSD. The model's performance indicates a strong fit to the observed data, proving capability of 1D-CNN to capture changes in water transparency. On the test dataset, the model achieved a high R-squared value of 0.890, a low root mean squared error (RMSE) of 0.023 and mean absolute error (MAE) of 0.014. These results demonstrate that employing a 1D-CNN in the spectral dimension of Sentinel-3 OLCI data is an effective approach for predicting water quality. These findings have significant implications for monitoring ZSD in coastal areas. By integrating diverse data sources and leveraging advanced machine learning algorithms, a more accurate and comprehensive assessment of water quality can be achieved.
更多查看译文
关键词
Secchi,Sentinel-3,OLCI,1D-CNN,Adriatic sea
AI 理解论文
溯源树
样例
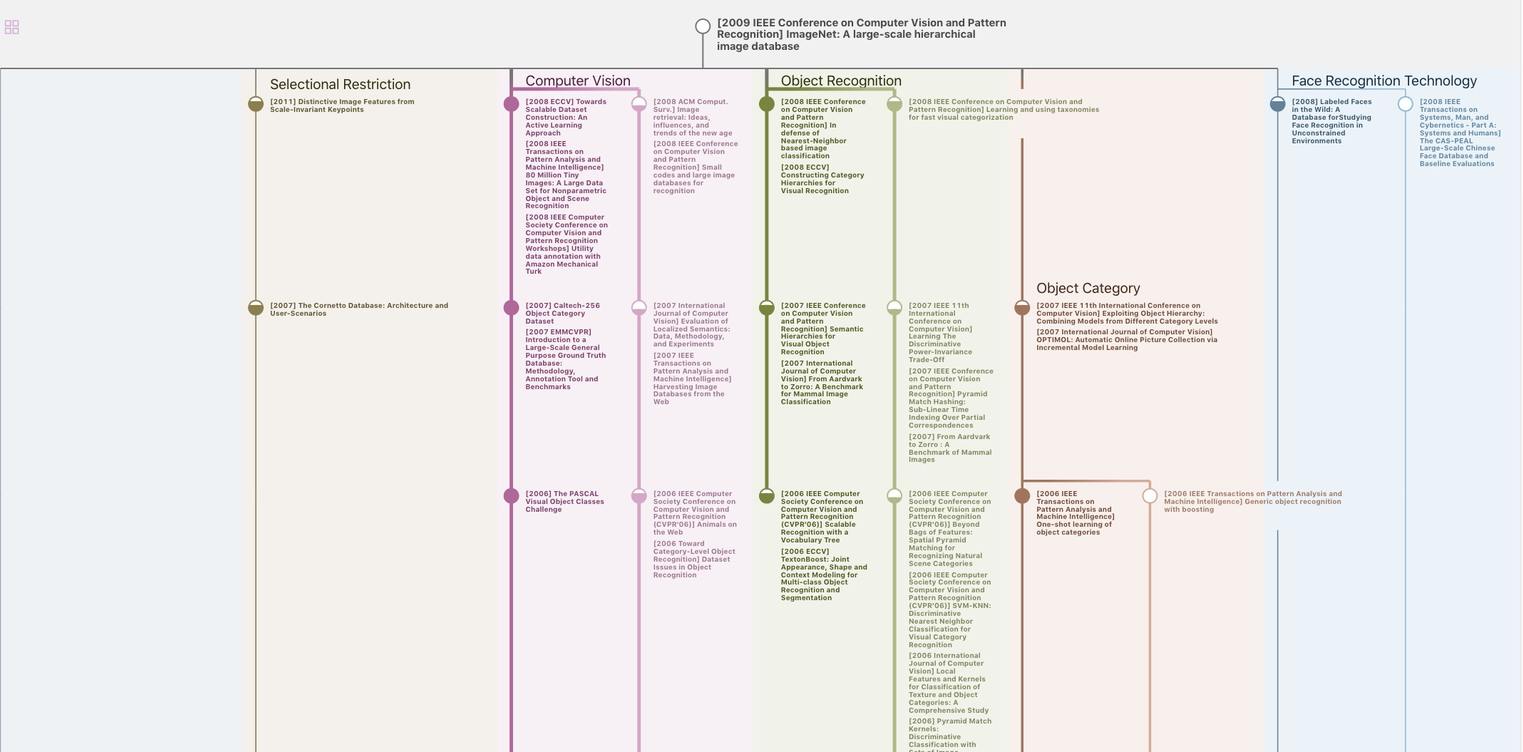
生成溯源树,研究论文发展脉络
Chat Paper
正在生成论文摘要