Tractable skew-normal approximations via matching
JOURNAL OF STATISTICAL COMPUTATION AND SIMULATION(2024)
摘要
Many approximate Bayesian inference methods assume a particular parametric form for approximating the posterior distribution. A Gaussian distribution provides a convenient density for such approaches; examples include the Laplace, penalized quasi-likelihood, Gaussian variational, and expectation propagation methods. Unfortunately, these all ignore potential posterior skewness. The recent work of Durante et al. [Skewed Bernstein-von Mises theorem and skew-modal approximations; 2023. ArXiv preprint arXiv:2301.03038.] addresses this using skew-modal (SM) approximations, and is theoretically justified by a skewed Bernstein-von Mises theorem. However, the SM approximation can be impractical to work with in terms of tractability and storage costs, and uses only local posterior information. We introduce a variety of matching-based approximation schemes using the standard skew-normal distribution to resolve these issues. Experiments were conducted to compare the performance of this skew-normal matching method (both as a standalone approximation and as a post-hoc skewness adjustment) with the SM and existing Gaussian approximations. We show that for small and moderate dimensions, skew-normal matching can be much more accurate than these other approaches. For post-hoc skewness adjustments, this comes at very little cost in additional computational time.
更多查看译文
关键词
Approximate Bayesian inference,moment matching,simulation,skew-normal distribution
AI 理解论文
溯源树
样例
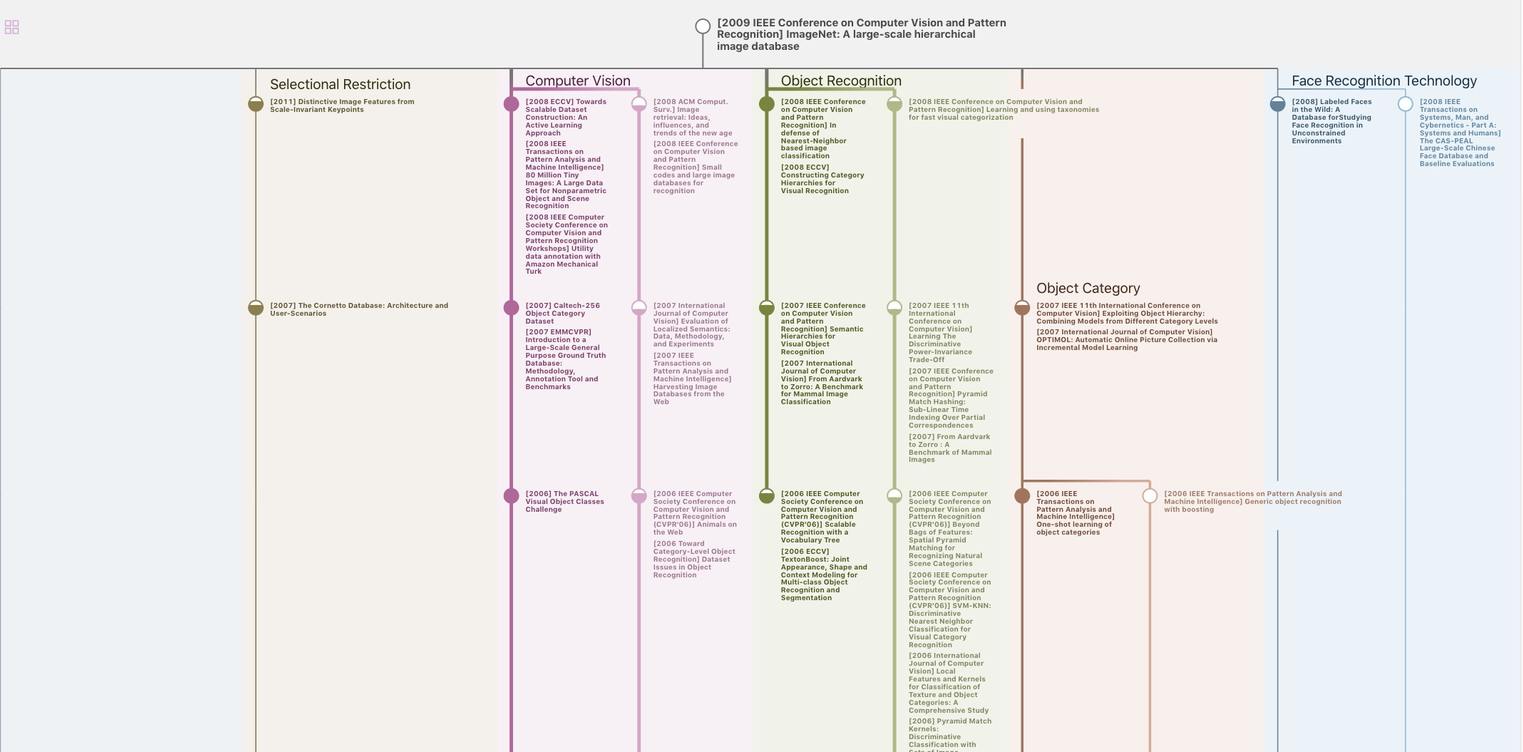
生成溯源树,研究论文发展脉络
Chat Paper
正在生成论文摘要