Dynamic Multiobjective Evolutionary Optimization via Knowledge Transfer and Maintenance
IEEE TRANSACTIONS ON SYSTEMS MAN CYBERNETICS-SYSTEMS(2024)
Abstract
This article suggests a new dynamic multiobjective evolutionary algorithm (DMOEA) with Knowledge Transfer and Maintenance, called KTM-DMOEA, which aims to alleviate the negative transfer and enhance the optimization efficiency. Two strategies, i.e., knowledge transfer prediction (KTP) and knowledge maintenance sampling (KMS), are proposed to excavate useful knowledge from historical environments. Particularly, KTP is a discriminative predictor designed to reduce the feature and distribution divergences across distinct environments, which classifies high-quality solutions from a large number of randomly generated solutions in new environment. Moreover, KMS is a generative predictor by modeling the distribution of elitist solutions in last environment, which can sample superior solutions in new environment according to the dynamic change trends. In this way, the advantages of KTP and KMS strategies are combined to produce a superior initial population in new environment, which help to alleviate the negative transfer and resultantly enhance the overall performance of KTM-DMOEA. When compared to several recently reported DMOEAs, the experimental results validate the advantages of KTM-DMOEA in tackling most cases of benchmark and real-world problems.
MoreTranslated text
Key words
Optimization,Maintenance engineering,Heuristic algorithms,Sociology,Knowledge transfer,Diversity reception,Transfer learning,Dynamic multiobjective optimization,evolutionary algorithm,knowledge maintenance,knowledge transfer
AI Read Science
Must-Reading Tree
Example
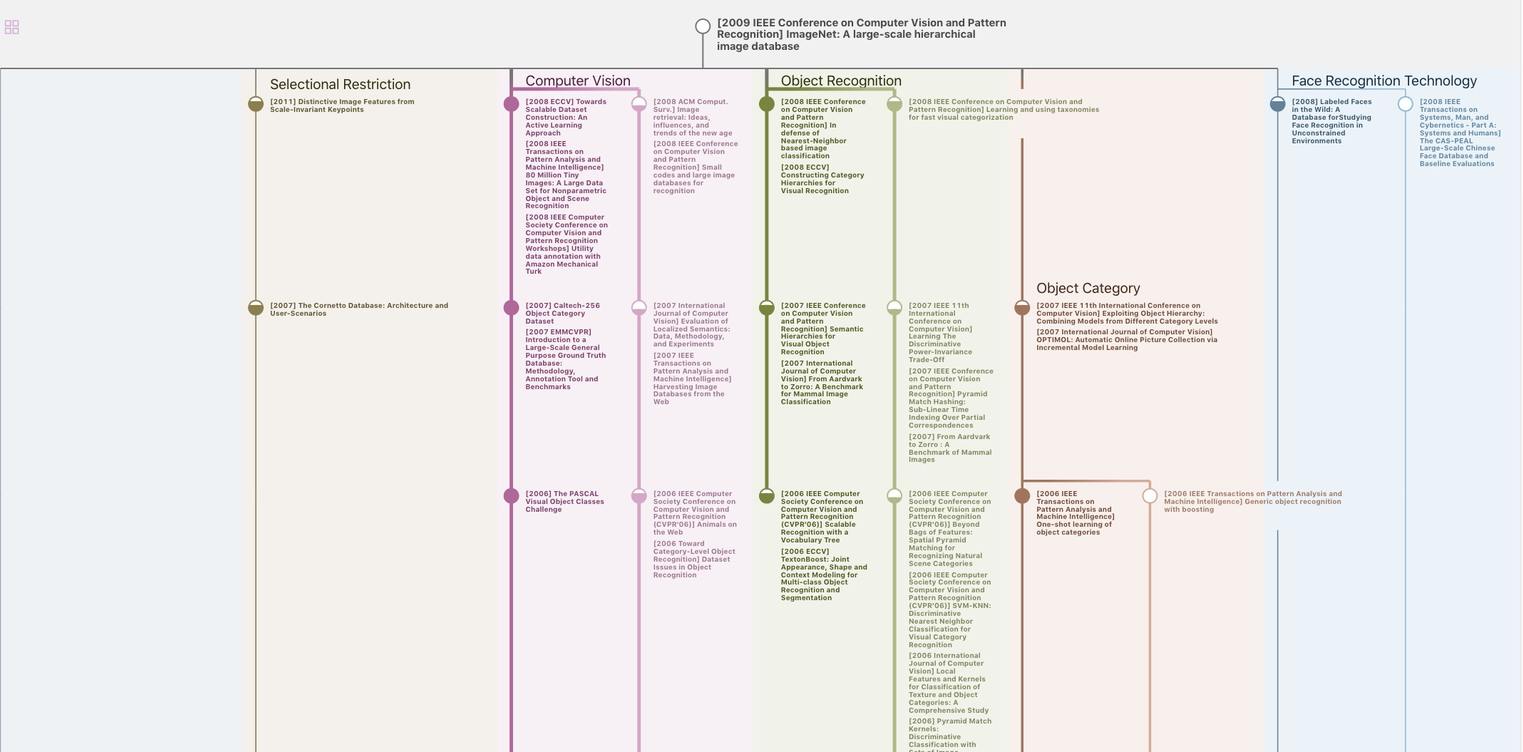
Generate MRT to find the research sequence of this paper
Chat Paper
Summary is being generated by the instructions you defined