A novel method of health indicator construction and remaining useful life prediction based on deep learning
EKSPLOATACJA I NIEZAWODNOSC-MAINTENANCE AND RELIABILITY(2023)
Abstract
The construction of health indicators (HI) for traditional deep learning requires human training labels and poor interpretability. This paper proposes an HI construction method based on Stacked Sparse Autoencoder (SSAE) and combines SSAE with Long short-term memory (LSTM) network to predict the remaining useful life (RUL). Extracting features from a single domain may result in insufficient feature extraction and cannot comprehensively reflect the degradation status information of mechanical equipment. In order to solve the problem, this article extracts features from time domain, frequency domain, and time-frequency domain to construct a comprehensive original feature set. Based on monotonicity, trendiness, and robustness, the most sensitive features from the original feature set are selected and put into the SSAE network to construct HI for state partitioning, and then LSTM is used for RUL prediction. By comparing with the existing methods, it is proved that the prediction effect of the proposed method in this paper is satisfied.
MoreTranslated text
Key words
stacked sparse autoencoders,health indicators,long short-term memory networks,remaining useful life prediction
AI Read Science
Must-Reading Tree
Example
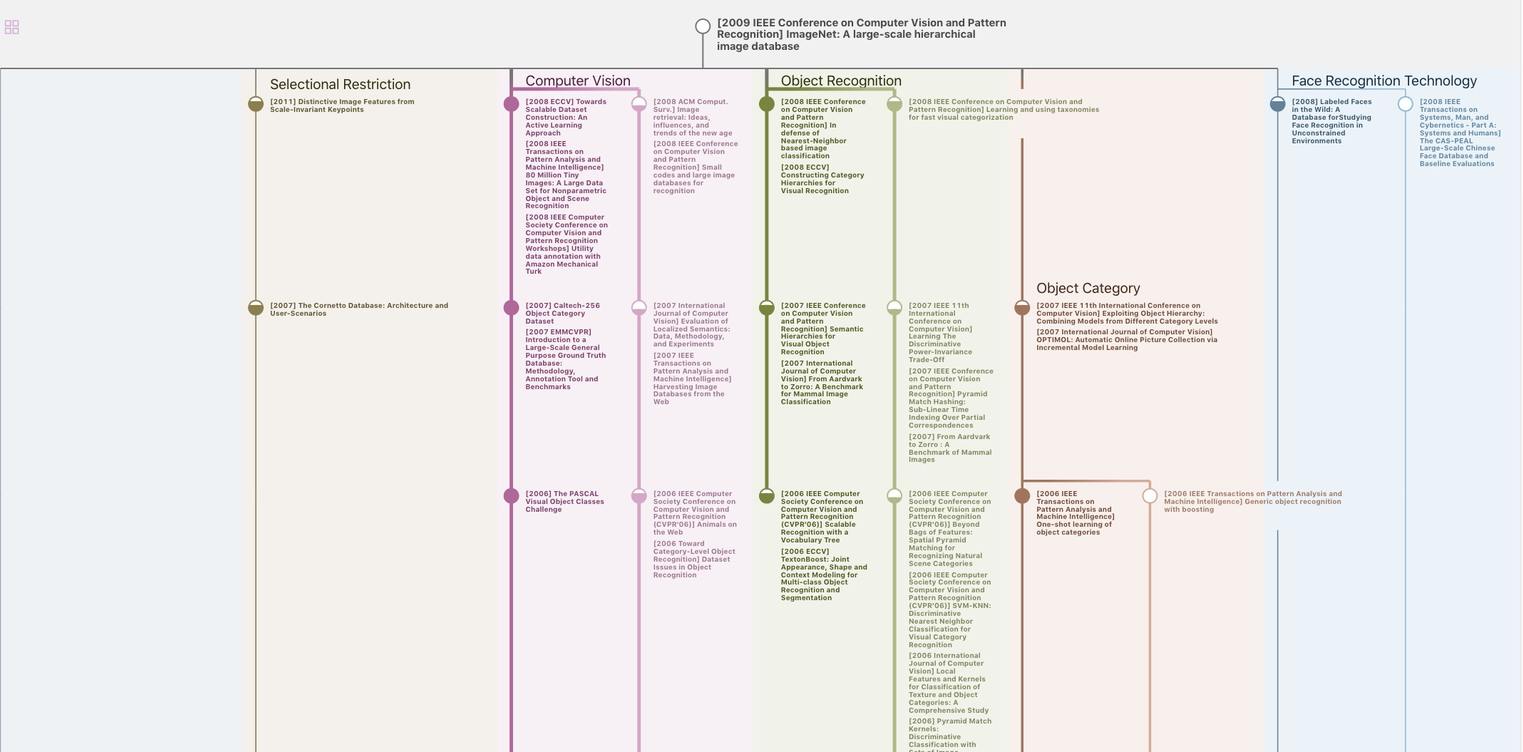
Generate MRT to find the research sequence of this paper
Chat Paper
Summary is being generated by the instructions you defined