Reinforcement Learning-Based Optimal Fault-Tolerant Tracking Control of Industrial Processes
INDUSTRIAL & ENGINEERING CHEMISTRY RESEARCH(2023)
摘要
For industrial processes with actuator faults, a reinforcement learning (RL)-based optimal fault-tolerant tracking control using a data-driven RL approach is proposed. First, a new state space model including tracking dynamics and state increment information is constructed. Subsequently, a performance index function is proposed based on the new model with actuator faults, followed by the derivation of the value function and Q-function. An RL algorithm is then designed to learn the optimal control law and optimize the performance index, resulting in the determination of the optimal controller gain. The proposed method holds promise, as it enables the expansion of the tolerable fault range of the system-a problem that has remained unresolved using reliable control techniques. It also achieves a good control effect even before the fault is eliminated, and the system performance is further improved through learning. The effectiveness and superiority of the proposed method are validated through a case study using a three-capacity water tank by comparing its performance with that of a model-based fault-tolerant tracking control method.
更多查看译文
关键词
control,industrial,learning-based,fault-tolerant
AI 理解论文
溯源树
样例
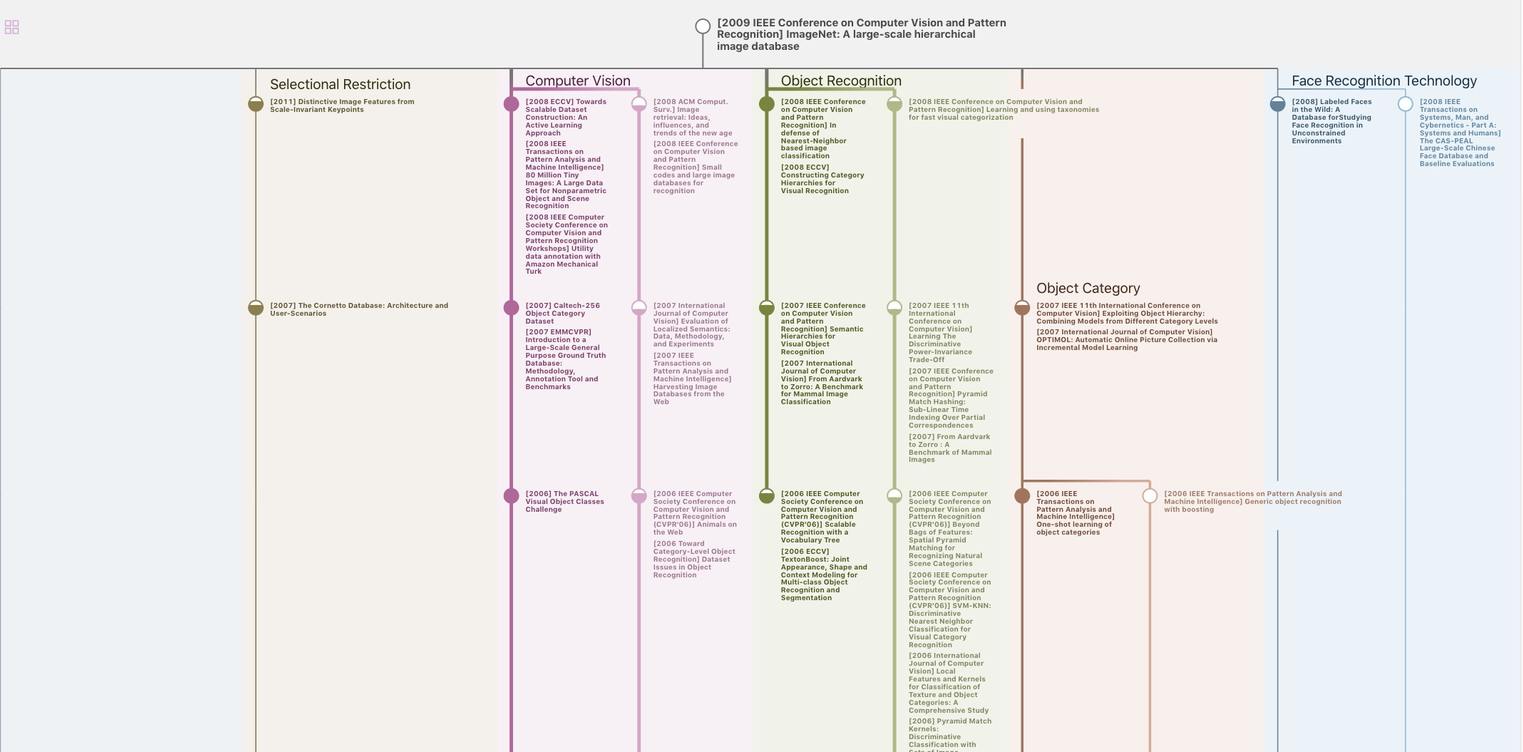
生成溯源树,研究论文发展脉络
Chat Paper
正在生成论文摘要