Data-driven ductile damage model for damage-induced material degradation in forming
MANUFACTURING LETTERS(2023)
摘要
In forming processes, the material undergoes large and complex deformations that influence the shape and properties of the final component. Especially the local weakening of the microstructure in the form of ductile damage, defined as the nucleation, growth and coalescence of voids, has a significant impact on the component's performance. To predict the void area fraction in a dual-phase steel, a data-driven model is used, which is trained with high-resolution scanning electron microscope data. The model provides good quantitative predictions of the void area fraction for various tensile and bending tests. In the next step, the model is coupled to the mechanical properties using the homogenization approach of effective stresses. The model is calibrated using force-displacement curves of various tensile tests. Besides the void area fraction, the model is able to predict the corresponding mechanical properties in the sense of the force-displacement response and to account for the material softening during air bending. (c) 2023 The Authors. Published by ELSEVIER Ltd. This is an open access article under the CC BY-NC-ND license (https://creativecommons.org/licenses/by-nc-nd/4.0)
更多查看译文
关键词
Damage evolution,Machine learning,Artificial neural networks,Metal forming,Material softening
AI 理解论文
溯源树
样例
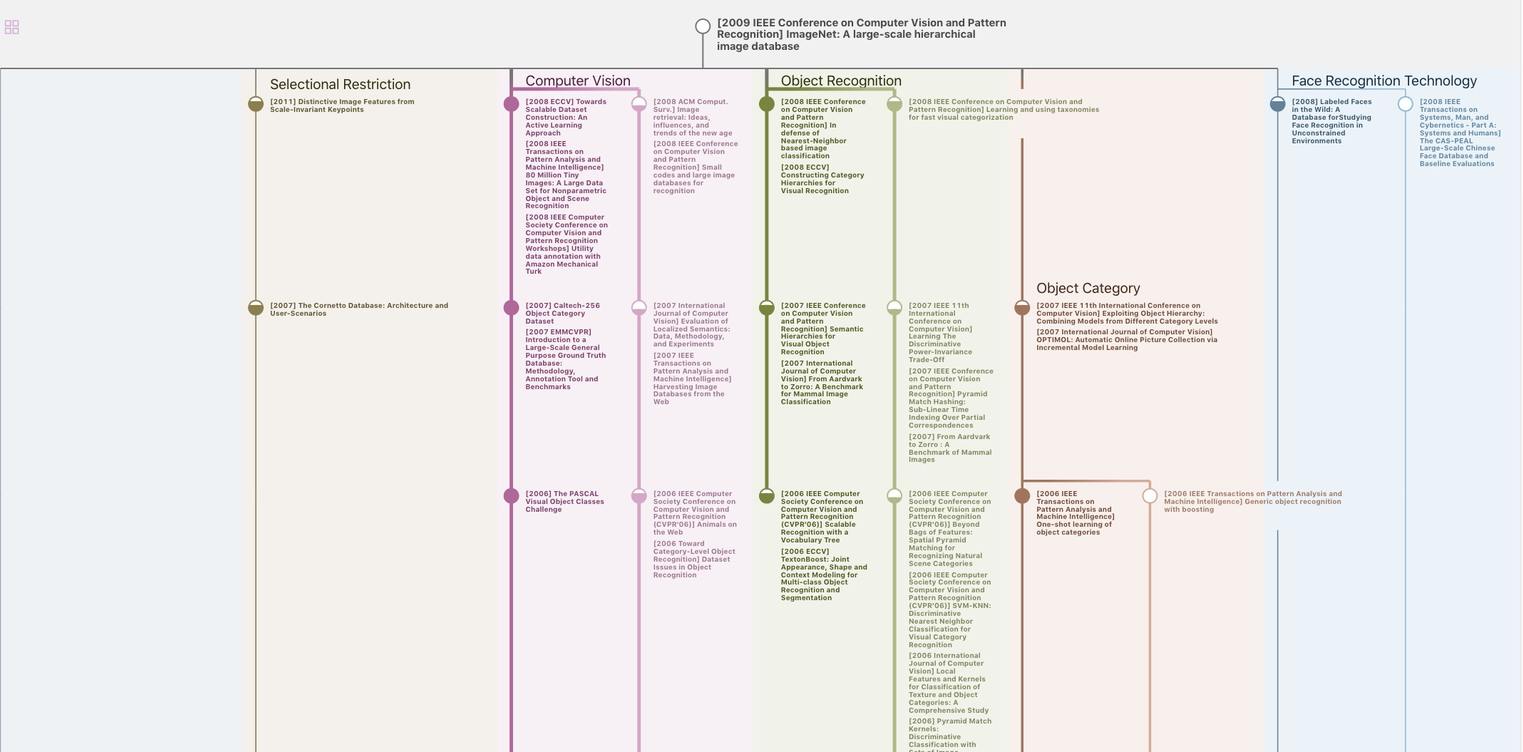
生成溯源树,研究论文发展脉络
Chat Paper
正在生成论文摘要