Hypothesis selection with Monte Carlo tree search for feature-based simultaneous localization and mapping in non-static environments
INTERNATIONAL JOURNAL OF ROBOTICS RESEARCH(2023)
摘要
A static world assumption is often used when considering the simultaneous localization and mapping (SLAM) problem. In reality, especially when long-term autonomy is the objective, this is not a valid assumption. This paper studies a scenario where landmarks can occupy multiple discrete positions at different points in time, where each possible position is added to a multi-hypothesis map representation. A selector-mixture distribution is introduced and used in the observation model. Each landmark position hypothesis is associated with one component in the mixture. The landmark movements are modeled by a discrete Markov chain and the Monte Carlo tree search algorithm is suggested to be used as component selector. The non-static environment model is further incorporated into the factor graph formulation of the SLAM problem and is solved by iterating between estimating discrete variables with a component selector and optimizing continuous variables with an efficient state-of-the-art nonlinear least squares SLAM solver. The proposed non-static SLAM system is validated in numerical simulation and with a publicly available dataset by showing that a non-static environment can successfully be navigated.
更多查看译文
关键词
Monte Carlo tree search, non-static environment, simultaneous localization and mapping, multi-hypothesis
AI 理解论文
溯源树
样例
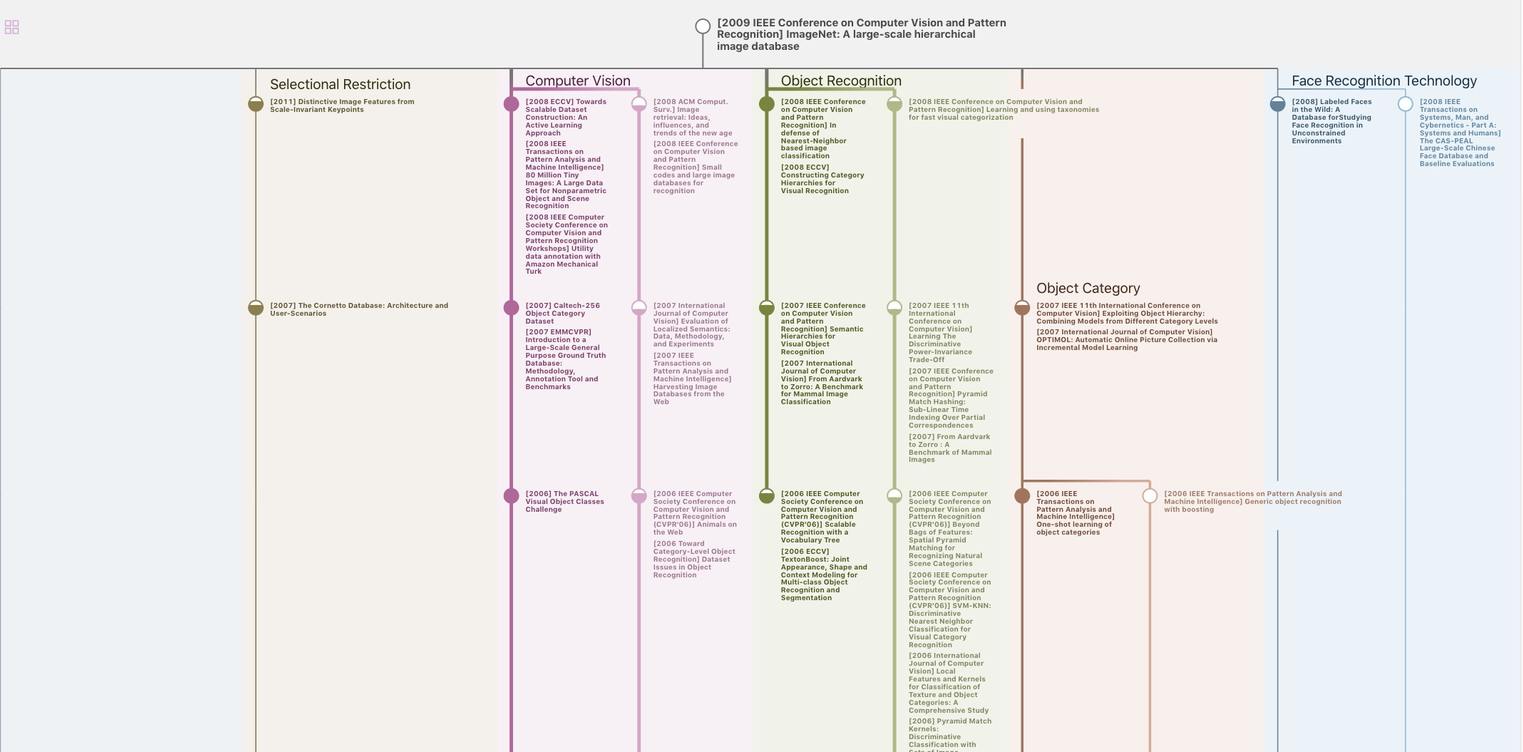
生成溯源树,研究论文发展脉络
Chat Paper
正在生成论文摘要