Binary-Stochasticity-Enabled Highly Efficient Neuromorphic Deep Learning Achieves Better-than-Software Accuracy
ADVANCED INTELLIGENT SYSTEMS(2024)
摘要
In this work, the requirement of using high-precision (HP) signals is lifted and the circuits for implementing deep learning algorithms in memristor-based hardware are simplified. The use of HP signals is required by the backpropagation learning algorithm since the gradient descent learning rule relies on the chain product of partial derivatives. However, it is both challenging and biologically implausible to implement such an HP algorithm in noisy and analog memristor-based hardware systems. Herein, it is demonstrated that the requirement for HP signals handling is not necessary and more efficient deep learning can be achieved when using a binary stochastic learning algorithm. The new algorithm proposed in this work modifies elementary neural network operations, which improves energy efficiency by two orders of magnitude compared to traditional memristor-based hardware and three orders of magnitude compared to complementary metal-oxide-semiconductor-based hardware. It also provides better accuracy in pattern recognition tasks than the HP learning algorithm benchmarks. Herein, a binary stochastic (BS) deep learning algorithm which is both biologically plausible and hardware friendly is proposed. The BS algorithm fully supports online training of memristor-based deep learning and shows better-than-software training and inference accuracy on benchmark tasks. It also improves energy efficiency by more than three orders of magnitude compared with conventional implementations.image (c) 2023 WILEY-VCH GmbH
更多查看译文
关键词
backpropagation,deep learning,neuromorphic computing,signal binarization,stochastic sampling
AI 理解论文
溯源树
样例
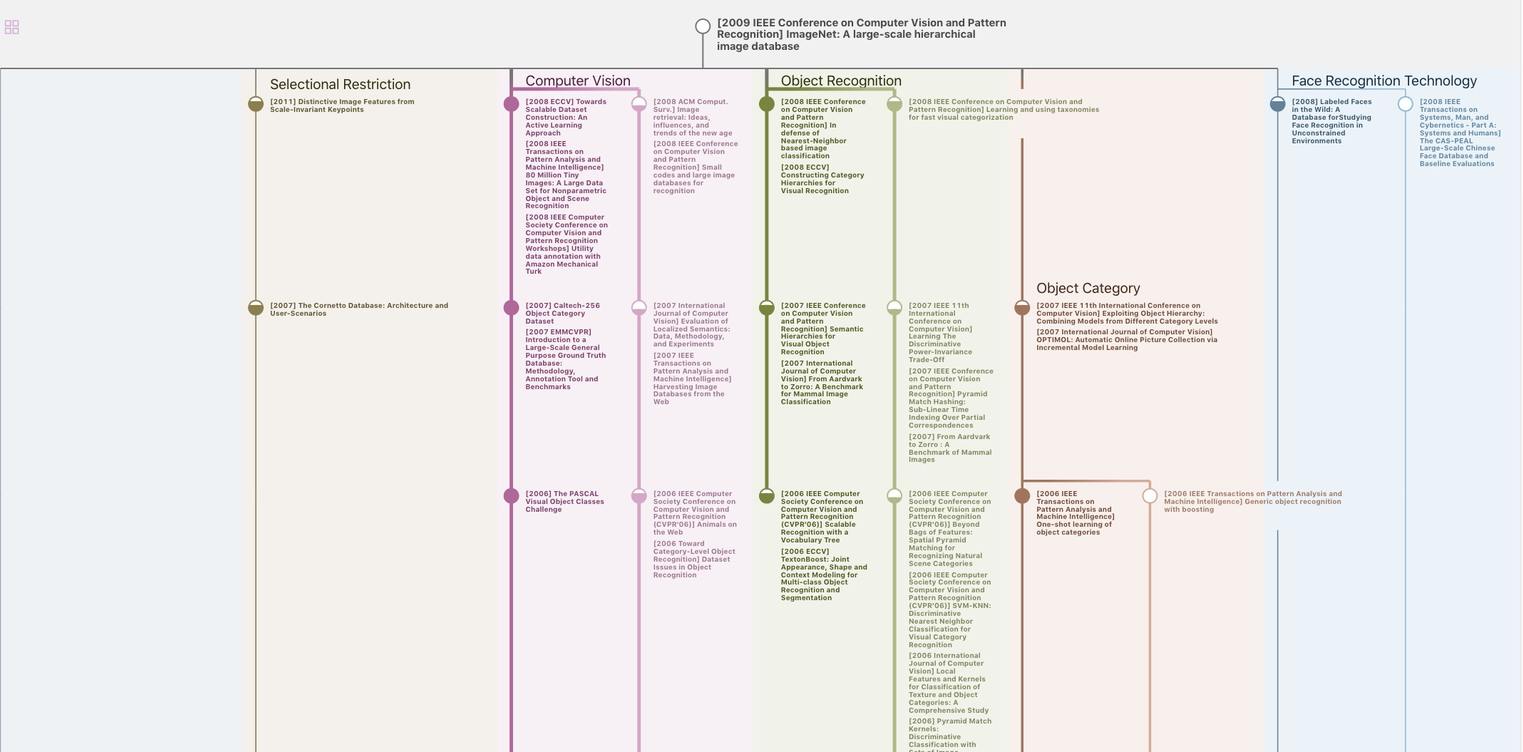
生成溯源树,研究论文发展脉络
Chat Paper
正在生成论文摘要