Machine learning approach with random forest and synthetic minority over-sampling techniquemay be optimal in non-invasive euploidy detection: a preliminary study
FERTILITY AND STERILITY(2023)
Abstract
The pre-implantation screening test for the diagnosis of euploidy has become indispensable in assisted reproductive interventions, but may be hard to access due to high cost. In light of the recent advancements, there exists the possibility of an artificial intelligence model based on non-invasive parameters that could hold substantial potential for the diagnosis of euploidy at a reduced cost. In this preliminary study, we aim to find the optimal strategy to predict the euploidy outcome of blastocysts by using different machine-learning approaches. In this analytical study, the database, including clinical characteristics and timelapse data from 522 euploidy and 914 non-euploidy embryos, was obtained from Taipei Fertility Center, Taipei, Taiwan. A total of 16 attributes were extracted and divided in a 7:3 ratio (training set: test set) for the model training. Logistic regression and machine learning methods including Random Forest (RF), Light GBM (LGBM), Ridge, eXtreme Gradient Boosting (XGB) Decision Tree (DT), Extra Tree (ET), K-Nearest Neighbor (KNN), Stochastic Gradient Descent (SGD), and Support Vector Classifier (SVC) were used for building prediction models. Synthetic Minority Over-sampling Technique (SMOTE) was used to overcome the overfitting problem posed by random oversampling. Retrospective data collection was approved by Institutional Review Board (No. A202205161). Our available databases, including maternal age, AMH, BMI, history of previous IVF cycles or artificial sperm retrieval, embryo morphology characteristics on day 3 and day 5, and timelapse data, were included in the analysis. In view of our data, SMOTE improved the accuracies in most of the included models. RF outperformed other machine learning methods with the highest accuracy (0.76±0.02) and was chosen for further analysis. In validation with test data, RF model showed an AUC of 0.84 in euploidy prediction (Accuracy=0.75, Sensitivity=0.79, Specificity=0.71, Precision=0.71, f1 score=0.75). In comparison to RF, conventional logistic regression model was associated with a much lower potential of predicting euploidy status: AUC=0.73, Accuracy=0.68, Sensitivity=0.68, Specificity=0.68, Precision=0.68, f1 score=0.68. However, after excluding time-lapse data, an RF model comprised of clinical data and embryo morphological characteristics demonstrated a non-inferior efficacy in euploidy prediction (AUC=0.83, Accuracy=0.75, Sensitivity=0.83, Specificity=0.68, Precision=0.68, f1 score=0.74) as compared with the above-mentioned RF model. The machine learning approach using RF in conjunction with SMOTE data augmentation outperformed conventional logistic regression model in euploidy prediction. Embryo morphokinetic characteristics appeared to have minimal impact on predicting embryo outcomes.
MoreTranslated text
Key words
random forest,machine learning,detection,over-sampling,non-invasive
AI Read Science
Must-Reading Tree
Example
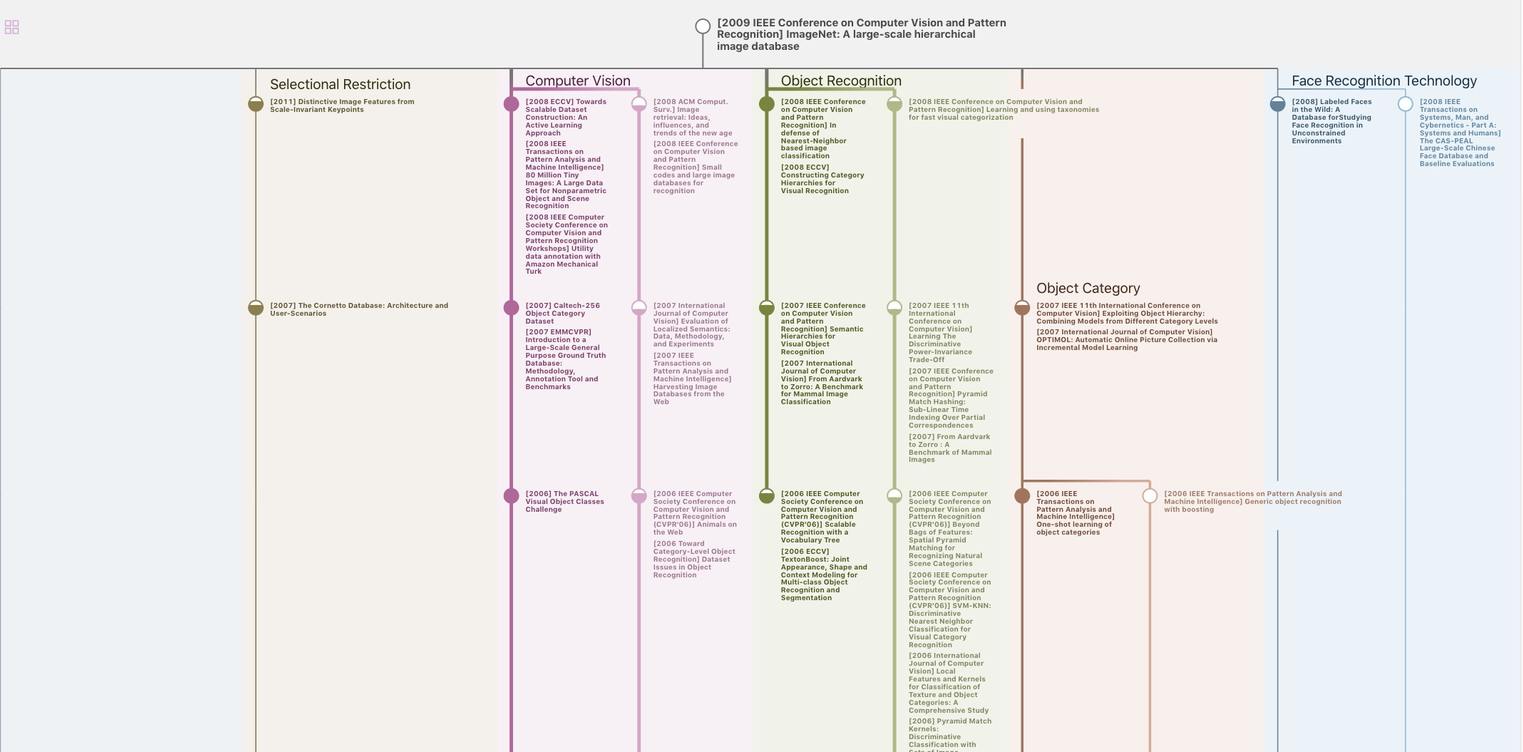
Generate MRT to find the research sequence of this paper
Chat Paper
Summary is being generated by the instructions you defined