Multiview Spectral Clustering Based on Consensus Neighbor Strategy
IEEE TRANSACTIONS ON NEURAL NETWORKS AND LEARNING SYSTEMS(2023)
摘要
Multiview spectral clustering, renowned for its spatial learning capability, has garnered significant attention in the data mining field. However, existing methods assume that the optimal consensus adjacency matrix is confined within the space spanned by each view's adjacency matrix. This constraint restricts the feasible domain of the algorithm and hinders the exploration of the optimal consensus adjacency matrix. To address this limitation, we propose a novel and convex strategy, termed the consensus neighbor strategy, for learning the optimal consensus adjacency matrix. This approach constructs the optimal consensus adjacency matrix by capturing the consensus local structure of each sample across all views, thereby expanding the search space and facilitating the discovery of the optimal consensus adjacency matrix. Furthermore, we introduce the concept of a correlation measuring matrix to prevent trivial solution. We develop an efficient iterative algorithm to solve the resulting optimization problem, benefitting from the convex nature of our model, which ensures convergence to a global optimum. Experimental results on 16 multiview datasets demonstrate that our proposed algorithm surpasses state-of-the-art methods in terms of its robust consensus representation learning capability. The code of this article is uploaded to https://github.com/PhdJiayiTang/Consensus-Neighbor-Strategy.git.
更多查看译文
关键词
Laplace equations,Data mining,Adaptation models,Computational efficiency,Learning systems,Correlation,Convergence,Consensus adjacency matrix,consensus neighbor,convex model,multiview clustering (MVC),spectral clustering
AI 理解论文
溯源树
样例
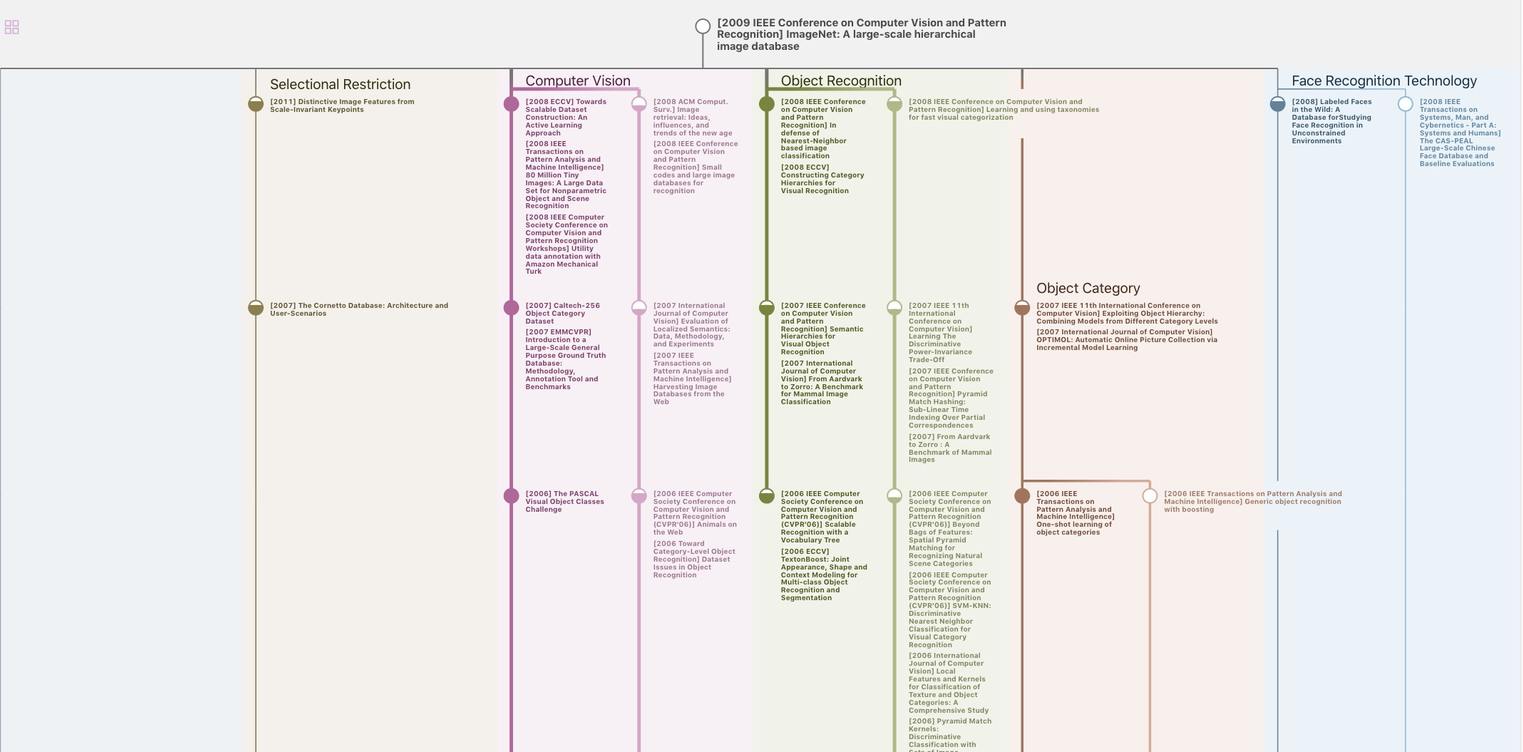
生成溯源树,研究论文发展脉络
Chat Paper
正在生成论文摘要