Sparse symbolscript-Laplace kernel online prediction for indoor localization in the Internet of Things
ENGINEERING APPLICATIONS OF ARTIFICIAL INTELLIGENCE(2023)
摘要
As an important component of IoT-oriented applications, the indoor positioning estimation is getting increasing concern with IoT's rapid development. However, the performance of indoor positioning is heavily relied on the complexity of the environment, which is always full of noises, such as Gaussian noise mixed with impulsive noise. These noises can deteriorate the precision performance of indoor positioning identification systems. In order to attack this problem, we propose a new kernel called generalized symbolscript-Laplace kernel to produce a new symbolscript-Laplace kernel adaptive filtering algorithm symbolscript which is combined with the recently proposed kernel mean p-power error criterion (KMPE). The proposed symbolscript has two vital features. Firstly, the symbolscript-Laplace kernel is employed to combat the Gaussian noise together with abrupt noise in real-world scenarios. Besides, the KMPE is utilized to obtain higher-order information in addition to second-order information which facilitates to suppress mixed noise. Furthermore, a Strengthened Surprise Criterion (SSC) is applied to symbolscript to reduce the size of neural networks. The symbolscript algorithm assisted by SSC is called Strengthened Surprise Criterion symbolscript-Laplace kernel adaptive filtering algorithm symbolscript Three experiments are carried out on two real -world scenarios to validate the effectiveness and accuracy performance. The experimental results demonstrate that the accuracy has been improved by at least 3.6%; meanwhile, the symbolscript neural network size can be reduced by up to 12.5%, without much loss of accuracy performance compared to symbolscript
更多查看译文
关键词
Kernel adaptive filtering,Indoor positioning,Impulsive noise,Internet of Things,Location accuracy
AI 理解论文
溯源树
样例
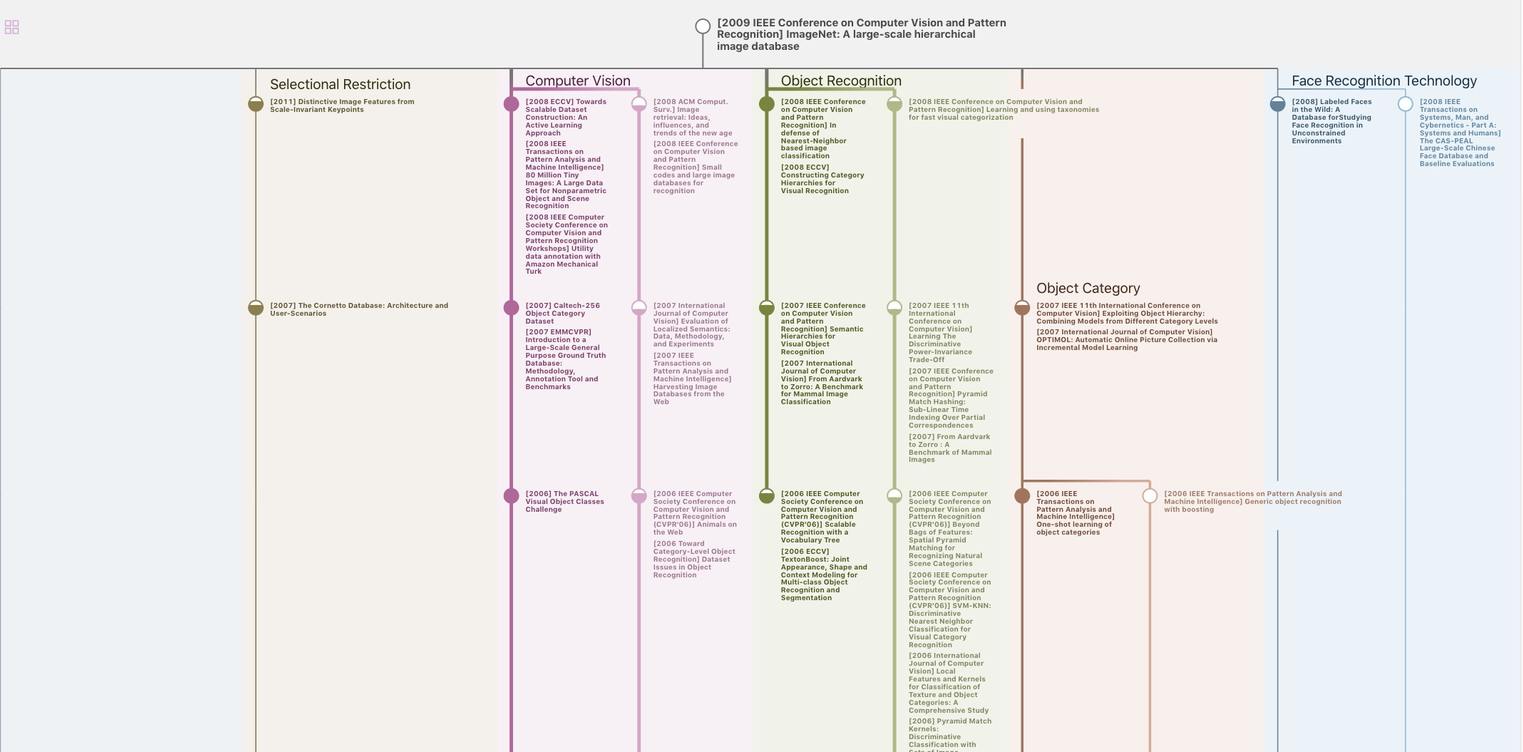
生成溯源树,研究论文发展脉络
Chat Paper
正在生成论文摘要