Learning the Color Space for Effective Fabric Defect Detection
IEEE TRANSACTIONS ON EMERGING TOPICS IN COMPUTATIONAL INTELLIGENCE(2024)
摘要
Fabric defect detection has been an important but challenging problem. One crucial problem lies in that the fabric colors and textures highly affect the detection performance. Especially, defects with low contrast to the fabric patterns are difficult to detect. We find that the color space is one important issue, and defects exhibit diverse discriminative levels given different color spaces. This observation inspired us to seek for an optimal color space for the defect detection problem. We propose a Color Conversion Network (CCN), which learns to project RGB colors into an optimized color space, where the fabric textures and the defective regions are the most distinguishable, to improve the defect detection performance. By introducing a contrastive loss, CCN can maximize the distance between defects and normal patterns effectively. The proposed CCN can be adopted as an add-in module that flexibly integrates with existing approaches to achieve universal improvement. Experiments demonstrate that the CCN module increases the fabric defect detection performance significantly with four state-of-the-art fabric defect detection approaches.
更多查看译文
关键词
Anomaly detection,color metric learning,color space,fabric defect detection
AI 理解论文
溯源树
样例
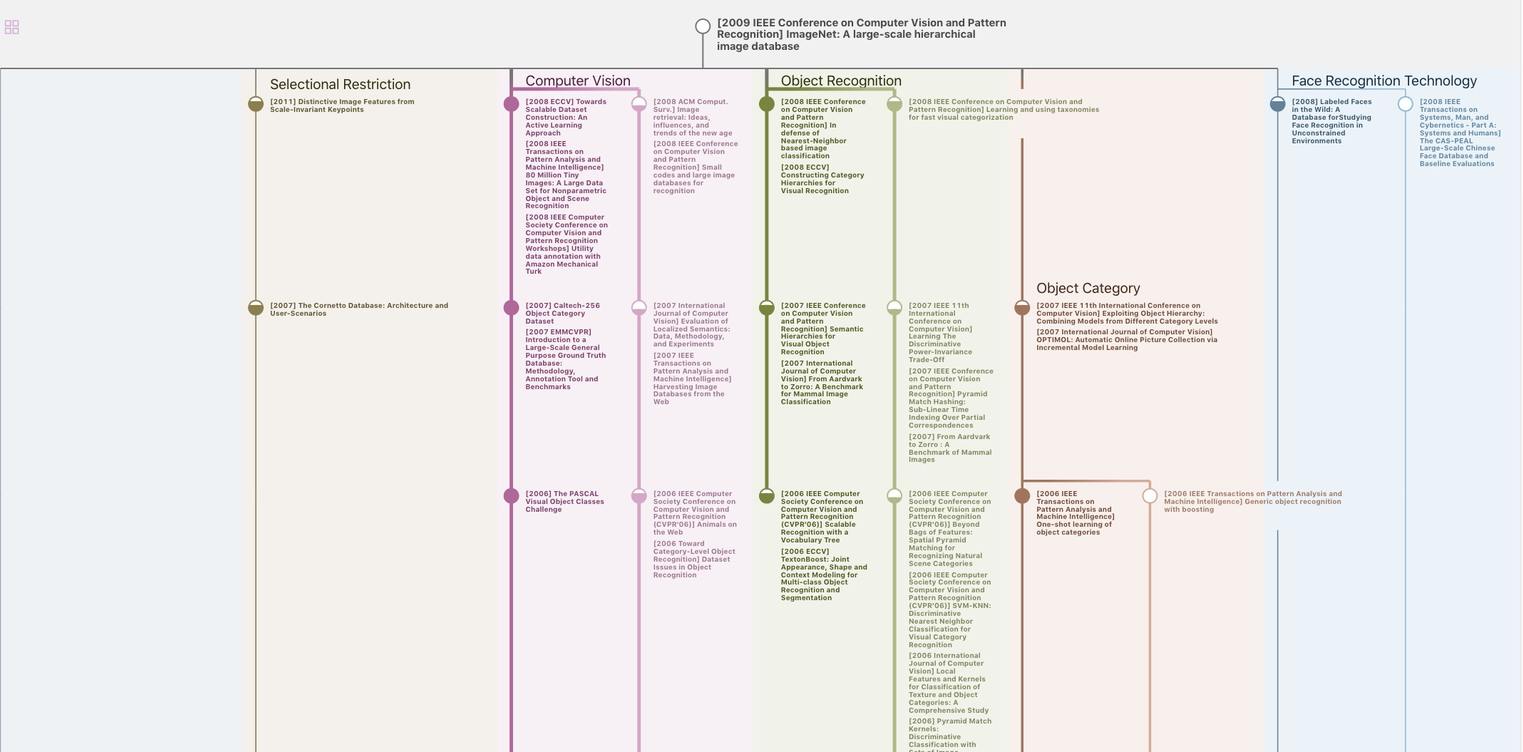
生成溯源树,研究论文发展脉络
Chat Paper
正在生成论文摘要