Condition control training-based ConVMLP-ResU-Net for semantic segmentation of esophageal cancer in 18F-FDG PET/CT images
PHYSICAL AND ENGINEERING SCIENCES IN MEDICINE(2023)
摘要
The precise delineation of esophageal gross tumor volume (GTV) on medical images can promote the radiotherapy effect of esophagus cancer. This work is intended to explore effective learning-based methods to tackle the challenging auto-segmentation problem of esophageal GTV. By employing the progressive hierarchical reasoning mechanism (PHRM), we devised a simple yet effective two-stage deep framework, ConVMLP-ResU-Net. Thereinto, the front-end ConVMLP integrates convolution (ConV) and multi-layer perceptrons (MLP) to capture localized and long-range spatial information, thus making ConVMLP excel in the location and coarse shape prediction of esophageal GTV. According to the PHRM, the front-end ConVMLP should have a strong generalization ability to ensure that the back-end ResU-Net has correct and valid reasoning. Therefore, a condition control training algorithm was proposed to control the training process of ConVMLP for a robust front end. Afterward, the back-end ResU-Net benefits from the yielded mask by ConVMLP to conduct a finer expansive segmentation to output the final result. Extensive experiments were carried out on a clinical cohort, which included 1138 pairs of F-18-FDG positron emission tomography/computed tomography (PET/CT) images. We report the Dice similarity coefficient, Hausdorff distance, and Mean surface distance as 0.82 +/- 0.13, 4.31 +/- 7.91 mm, and 1.42 +/- 3.69 mm, respectively. The predicted contours visually have good agreements with the ground truths. The devised ConVMLP is apt at locating the esophageal GTV with correct initial shape prediction and hence facilitates the finer segmentation of the back-end ResU-Net. Both the qualitative and quantitative results validate the effectiveness of the proposed method.
更多查看译文
关键词
Automatic segmentation, Esophageal gross tumor volume, ConVMLP-ResU-Net, Condition control training algorithm, PET/CT
AI 理解论文
溯源树
样例
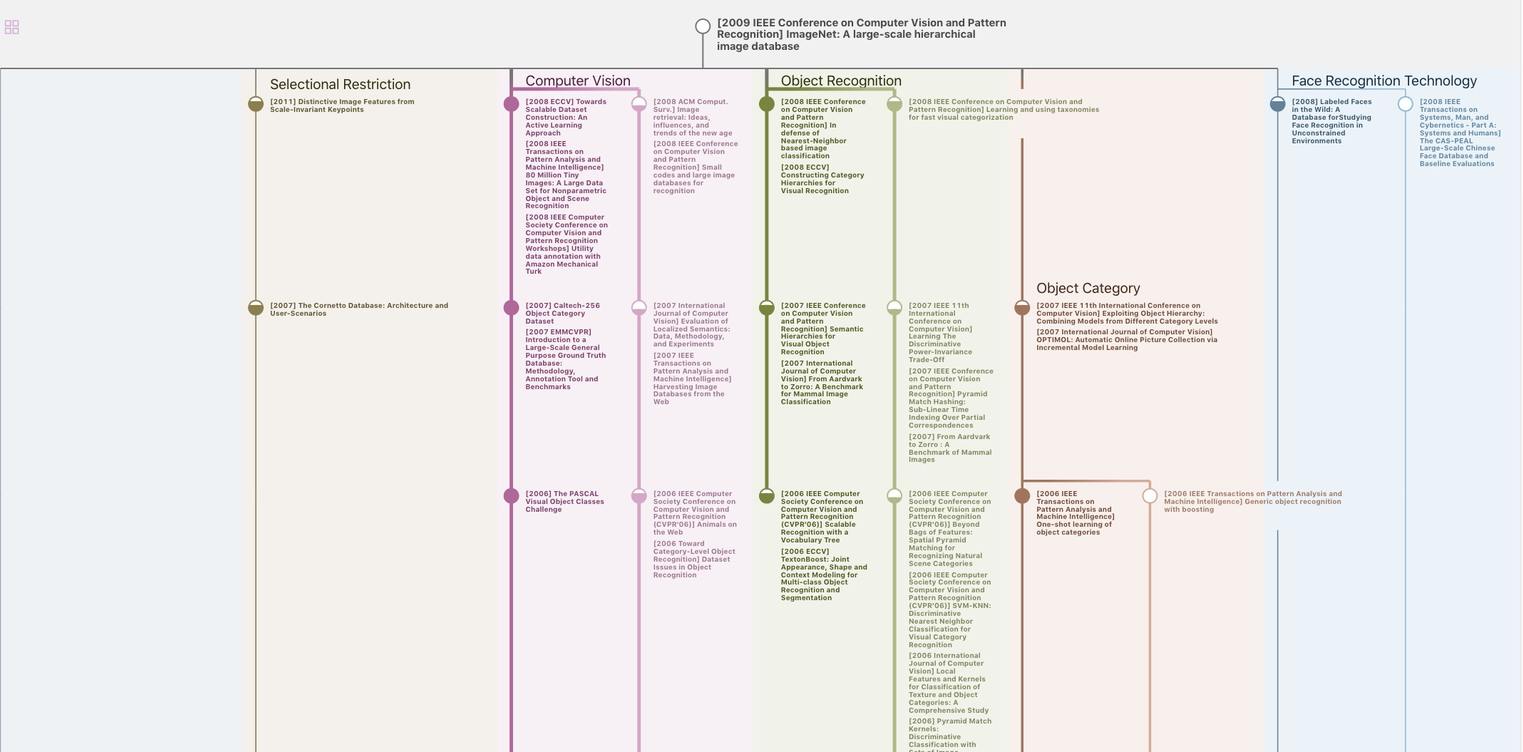
生成溯源树,研究论文发展脉络
Chat Paper
正在生成论文摘要