A Modified Maximum Entropy Inverse Reinforcement Learning Approach for Microgrid hnergy Scheduling
2023 IEEE POWER & ENERGY SOCIETY GENERAL MEETING, PESGM(2023)
摘要
Increasing popularity of integrating distributed energy resources (DERs) into the power system brings a challenge to optimize the dispatch policy for microgrid energy scheduling. The reinforcement learning methods suffer from a long-time problem with the empirical assumption of the reward function for the microgrid system. Although the traditional inverse reinforcement. learning (IRL) approaches can solve this problem to some extent, they encounter a limitation of extensive computations for state visitation frequency in the large and continuous state space. To alleviate this limitation, we propose a modified maximum entropy IRL (MMIRL) method to extract the reward function from the expert demonstrations for solving the microgrid energy scheduling problem. The computation of state visitation frequency is avoided by calculating the difference between the expert feature expectation and learner feature expectation. The microgrid optimization is suitable for using state-action (s, a) feature than state s feature only to recover the reward, and this setting drives the need for a computationally efficient method. To this end, the proposed MMIRL algorithm is designed to recover the reward function and learn the dispatch policy compared to the conventional approaches for microgrid energy scheduling. Case studies are performed in an energy arbitrage problem and a microgrid system with DERs, respectively. Results substantiate that the proposed MMIRL approach can learn the dispatch policy with more than 99% accuracy and outperforms other comparative methods in both cases.
更多查看译文
关键词
Distributed energy resources, reinforcement learning, maximum entropy inverse reinforcement learning, microgrid energy scheduling, and operation optimization
AI 理解论文
溯源树
样例
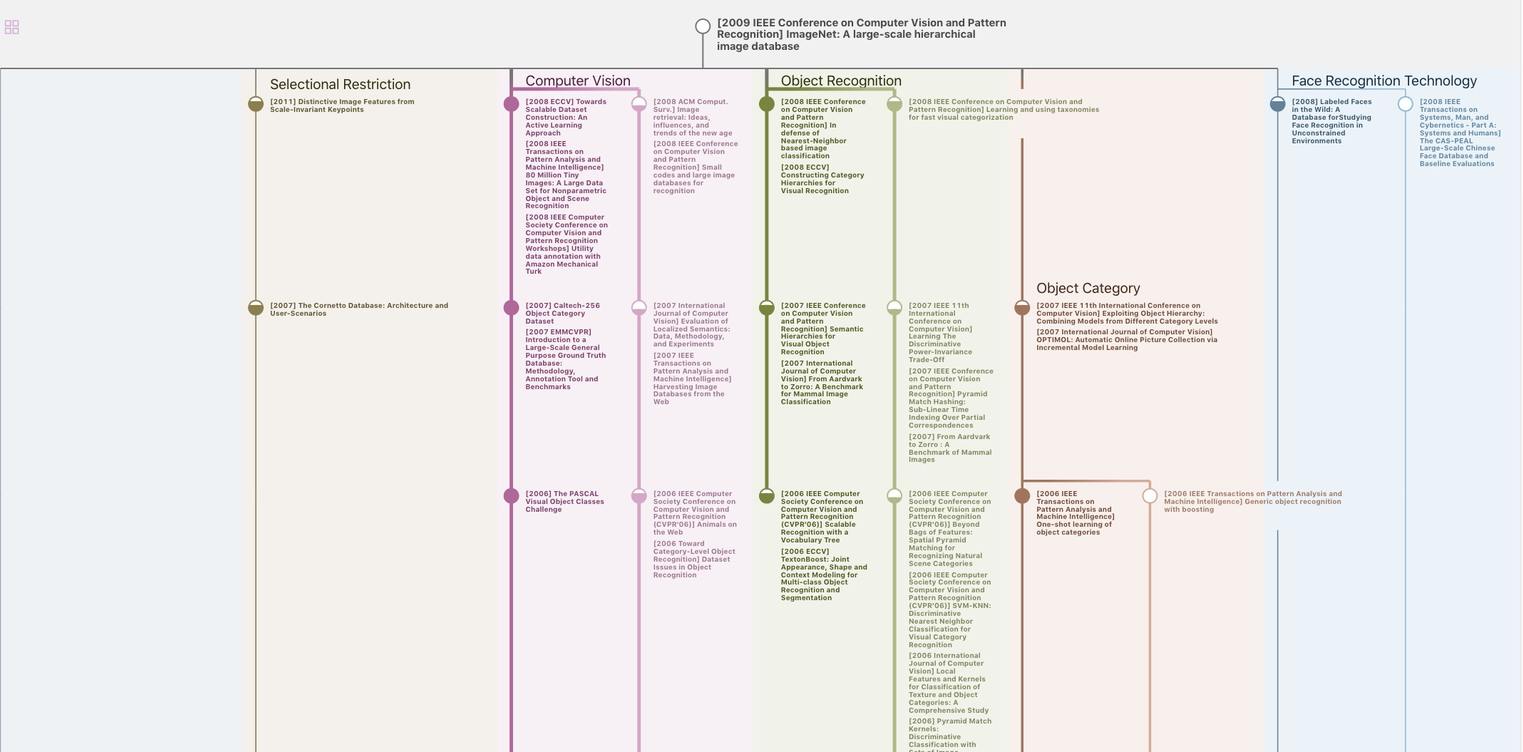
生成溯源树,研究论文发展脉络
Chat Paper
正在生成论文摘要