Fault Diagnosis of the LAMOST Fiber Positioner Based on a Long Short-term Memory(LSTM)Deep Neural Network
RESEARCH IN ASTRONOMY AND ASTROPHYSICS(2023)
摘要
The Large Sky Area Multi-Object Fiber Spectroscopic Telescope(LAMOST)has been in normal operation for more than 10 yr,and routine maintenance is performed on the fiber positioner every summer.The positioning accuracy of the fiber positioner directly affects the observation performance of LAMOST,and incorrect fiber positioner positioning accuracy will not only increase the interference probability of adjacent fiber positioners but also reduces the observation efficiency of LAMOST.At present,during the manual maintenance process of the positioner,the fault cause of the positioner is determined and analyzed when the positioning accuracy does not meet the preset requirements.This causes maintenance to take a long time,and the efficiency is low.To quickly locate the fault cause of the positioner,the repeated positioning accuracy and open-loop calibration curve data of each positioner are obtained in this paper through the photographic measurement method.Based on a systematic analysis of the operational characteristics of the faulty positioner,the fault causes are classified.After training a deep learning model based on long short-term memory,the positioner fault causes can be quickly located to effectively improve the efficiency of positioner fault cause analysis.The relevant data can also provide valuable information for annual routine maintenance methods and positioner designs in the future.The method of using a deep learning model to analyze positioner operation failures introduced in this paper is also of general significance for the maintenance and design optimization of fiber positioners using a similar double-turn gear transmission system.
更多查看译文
关键词
telescopes,techniques:image processing,methods:analytical,techniques:spectroscopic
AI 理解论文
溯源树
样例
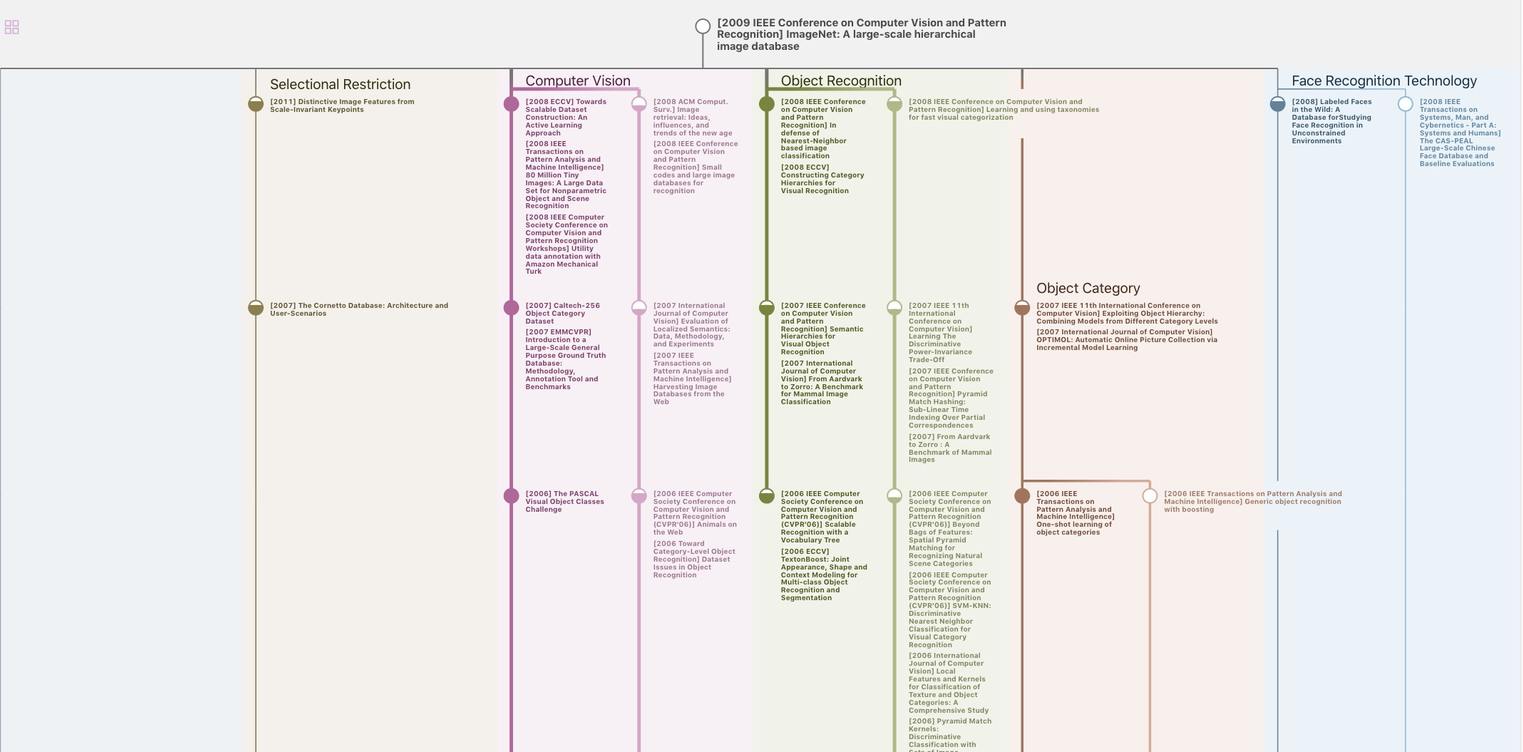
生成溯源树,研究论文发展脉络
Chat Paper
正在生成论文摘要