Cross-media retrieval via fusing multi-modality and multi-grained data
SCIENTIA IRANICA(2023)
摘要
Traditional cross-media retrieval methods mainly focus on coarse-grained data that reflect global characteristics while ignoring the fine-grained descriptions of local details. Meanwhile, traditional methods cannot accurately describe the correlations between the anchor and the irrelevant data. This paper aims to solve the abovementioned problems by proposing to fuse coarse-grained and fine-grained features and a multi-margin triplet loss based on a dual-framework. (1) Framework I: A multi-grained data fusion framework based on Deep Belief Network, and (2) Framework II: A multi-modality data fusion framework based on the multi-margin triplet loss function. In Framework I, the coarse-grained and fine-grained features fused by the joint Restricted Boltzmann Machine are input into Framework II. In Framework II, we innovatively propose the multi-margin triplet loss. The data, which belong to different modalities and semantic categories, are stepped away from the anchor in a multi-margin way. Experimental results show that the proposed method achieves better cross-media retrieval performance than other methods with different datasets. Furthermore, the ablation experiments verify that our proposed multi-grained fusion strategy and the multi-margin triplet loss function are effective. (c) 2023 Sharif University of Technology. All rights reserved.
更多查看译文
关键词
retrieval,cross-media,multi-modality,multi-grained
AI 理解论文
溯源树
样例
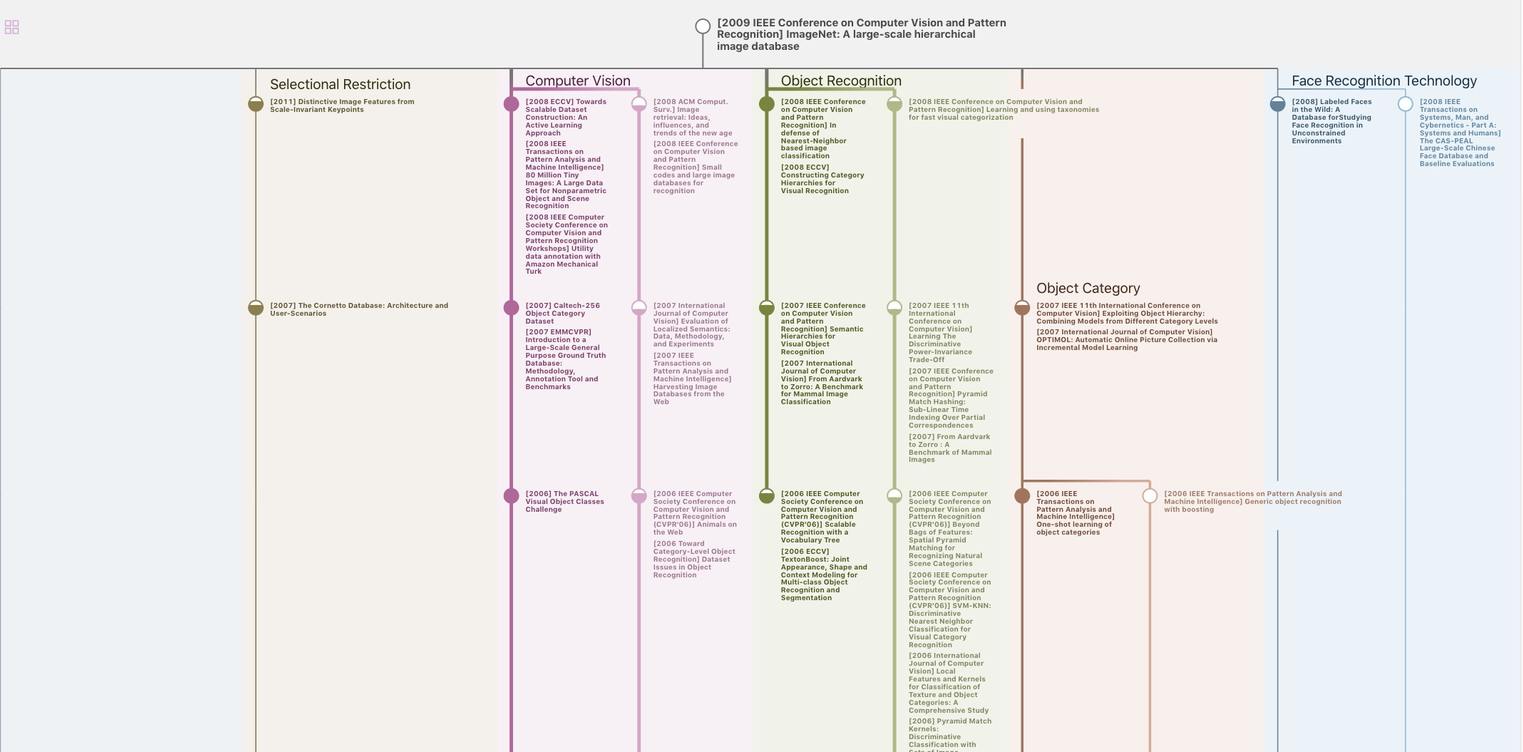
生成溯源树,研究论文发展脉络
Chat Paper
正在生成论文摘要