Classification of urban interchange patterns using a model combining shape context descriptor and graph convolutional neural network
GEO-SPATIAL INFORMATION SCIENCE(2023)
摘要
Pattern recognition is critical to map data handling and their applications. This study presents a model that combines the Shape Context (SC) descriptor and Graph Convolutional Neural Network (GCNN) to classify the patterns of interchanges, which are indispensable parts of urban road networks. In the SC-GCNN model, an interchange is modeled as a graph, wherein nodes and edges represent the interchange segments and their connections, respectively. Then, a novel SC descriptor is implemented to describe the contextual information of each interchange segment and serve as descriptive features of graph nodes. Finally, a GCNN is designed by combining graph convolution and pooling operations to process the constructed graphs and classify the interchange patterns. The SC-GCNN model was validated using interchange samples obtained from the road networks of 15 cities downloaded from OpenStreetMap. The classification accuracy was 87.06%, which was higher than that of the image-based AlexNet, GoogLeNet, and Random Forest models.
更多查看译文
关键词
Road networks,interchange pattern,classification,Graph Convolutional Neural Networks (GCNNs),Shape Context (SC) descriptor
AI 理解论文
溯源树
样例
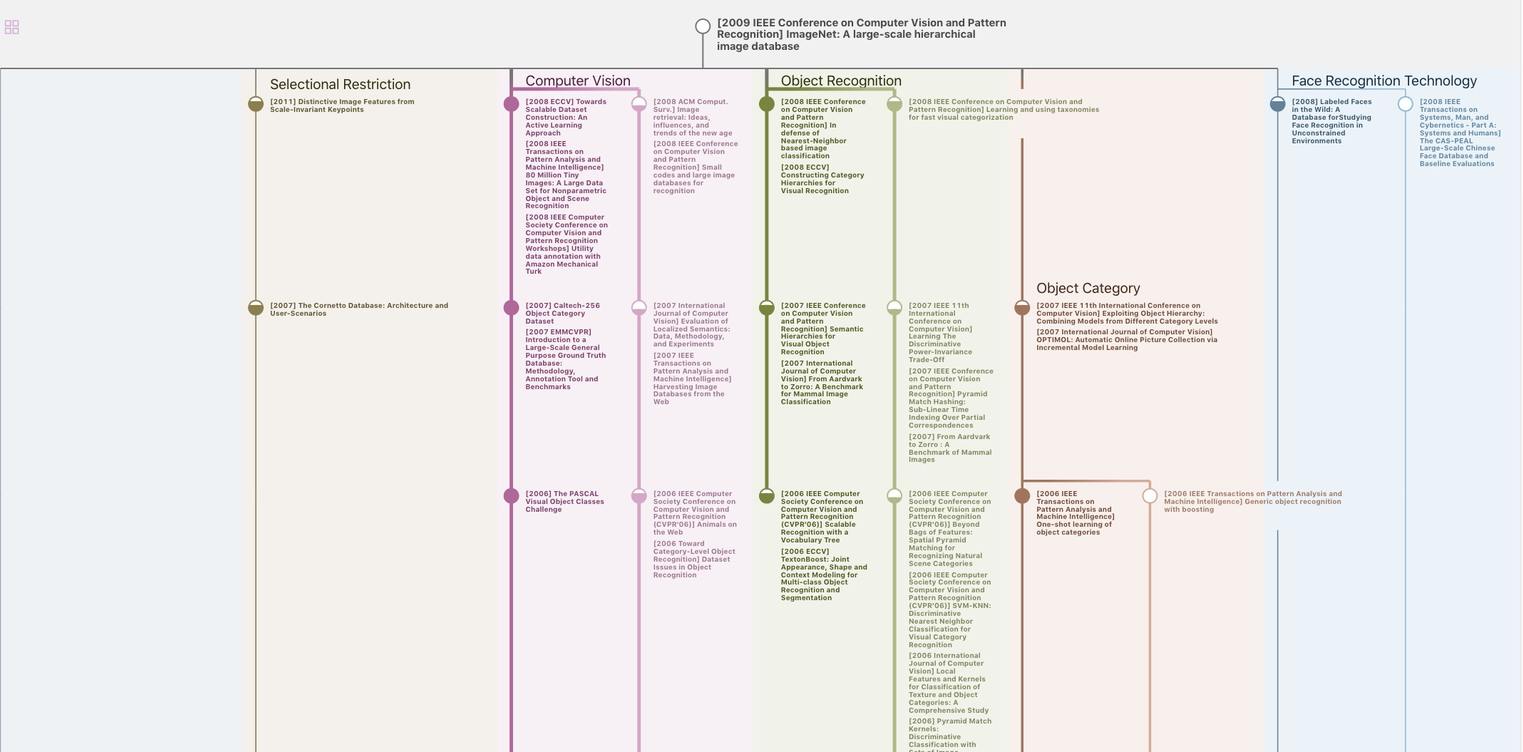
生成溯源树,研究论文发展脉络
Chat Paper
正在生成论文摘要