Domain Adaptation for In-Air to Underwater Image Enhancement via Deep Learning
IEEE TRANSACTIONS ON EMERGING TOPICS IN COMPUTATIONAL INTELLIGENCE(2024)
摘要
Underwater image enhancement has drawn a lot of attention due to its significance in ocean exploration. Since it is extremely hard to obtain a degraded image and its clear version in the same scene, most deep learning-based methods utilize synthetic datasets for training. However, synthetic datasets often fail to describe the natural appearance and show poor capabilities of generalization to real scenes. In this study, we present an in-air to underwater image enhancement (IUIE) framework that overcomes the limitations of underwater synthetic datasets. Specifically, the presented framework consists of a color correction step and a domain adaptation step. In the color correction step, we propose a color correction mechanism by utilizing the white balance algorithm to eliminate color bias and produce a more natural appearance. In the domain adaptation step, the network can reduce the domain gap between the in-air domain and the underwater domain and obtain a better generalization in real scenes. To accommodate spatially varying textures and edges, we propose a residual adaptive module in the domain adaptation network. Experimental results on the test data indicate that our IUIE framework not only obtains more remarkable UIQM and BRISQUE scores than the previous methods do but also achieves superior results in visual perception.
更多查看译文
关键词
Image color analysis,Adaptation models,Image enhancement,Synthetic data,Task analysis,Histograms,Feature extraction,Underwater image enhancement,domain adaptation,deep learning
AI 理解论文
溯源树
样例
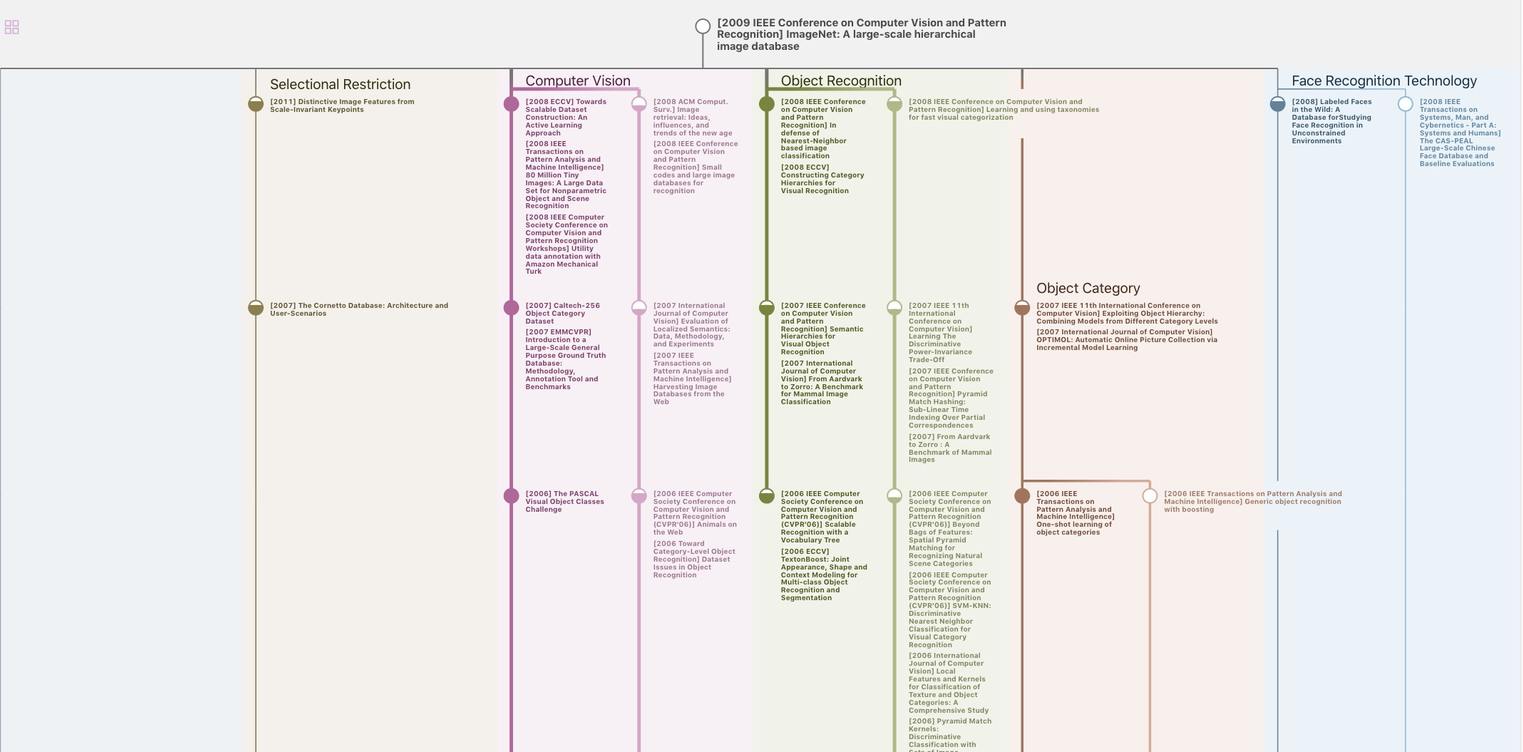
生成溯源树,研究论文发展脉络
Chat Paper
正在生成论文摘要