Applied Machine Learning to Analyze and Predict CO2 Adsorption Behavior of Metal-Organic Frameworks
Carbon capture science & technology(2023)
摘要
Machine learning provides new insights for designing MOFs with high CO2 adsorption capacity and understanding the CO2 adsorption mechanism. In this work, 348 data points from published reports were collected and four tree-based models were designed to predict the CO2 adsorption capacity of MOFs by machine learning. The results showed that the Random Forest (RF) had the best prediction performance (R2train = 0.970, R2test = 0.896). Feature importance analysis revealed the relative importance of CO2 adsorption parameters (73 %), textures (23 %) and metal centers of MOFs (4 %) for the CO2 adsorption process. Single and synergistic effects of different features were observed through partial dependence analysis. MOFs with Cu, Fe, Co, and Ni metal centers exhibited a promoting effect on CO2 adsorption. In addition, under high pressure, well-developed textures had significant positive impact on CO2 adsorption capacity, while under medium and low pressure, textures were not determining factors.
更多查看译文
关键词
MOFs,Machine learning,Random forest,Features analysis,CO2 adsorption
AI 理解论文
溯源树
样例
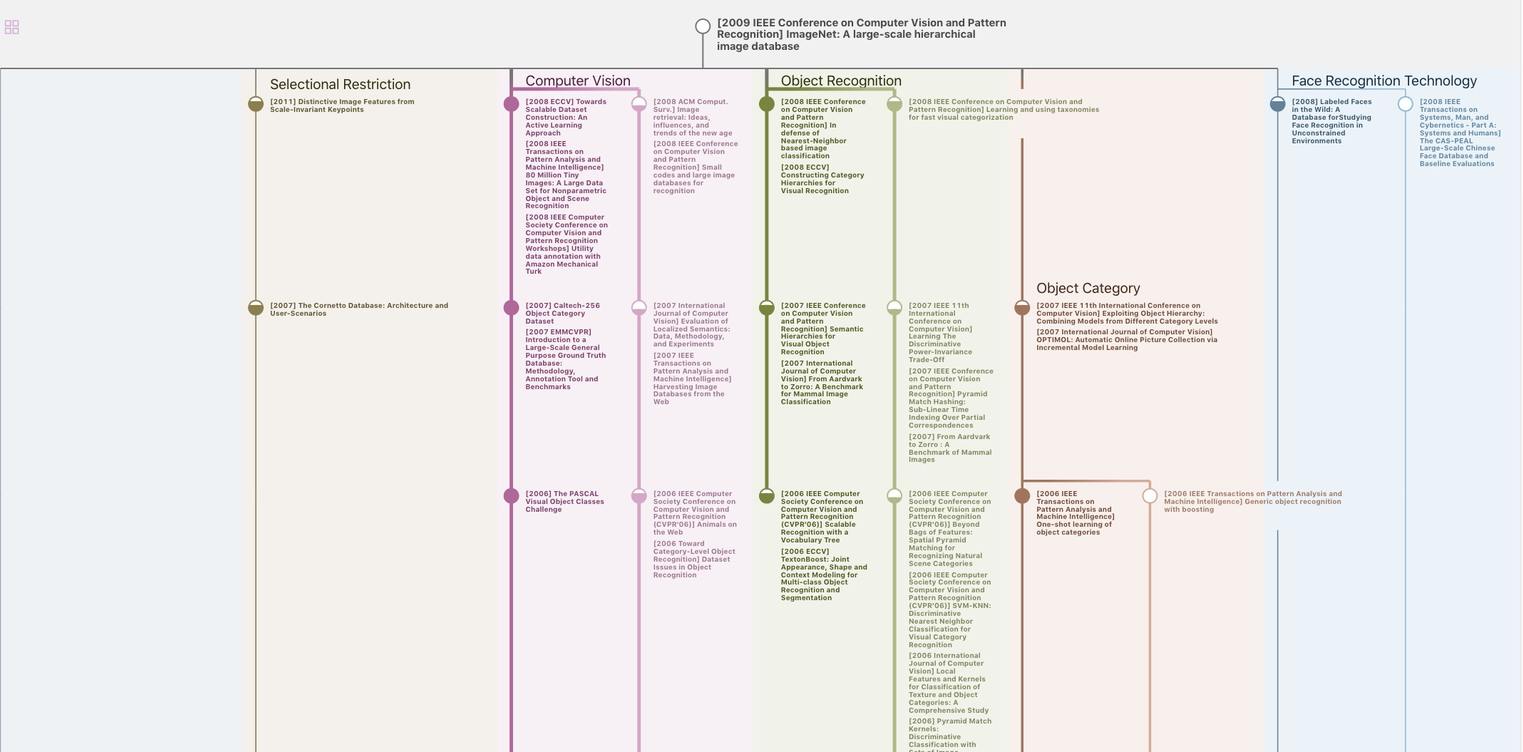
生成溯源树,研究论文发展脉络
Chat Paper
正在生成论文摘要