LGABL: UHD Multi-Exposure Image Fusion via Local and Global Aware Bilateral Learning
IEEE TRANSACTIONS ON EMERGING TOPICS IN COMPUTATIONAL INTELLIGENCE(2024)
摘要
Multi-exposure image fusion (MEF) technology is to generate a normally exposed image by fusing images with different exposure levels. Most of the existing models use CNNs to capture the features, which may have difficulty modeling the responses between pixels that are far apart in the spatial domain due to the fixed receptive field, especially for pixel-dense ultra high definition (UHD) images. Furthermore, most models are trapped by the parameter size and cannot process high-resolution images in real time with limited resources. To address these problems, we propose a local and global aware bilateral learning approach (LGABL) in this paper. Our model integrates the local and global features. We design the Non-stem MLP-Mixer to extract global information in different dimensions without CNN stems. In addition, the local features are obtained by the generalized CNN model. Finally, the merged features are utilized to yield a bilateral grid to reconstruct the images, and a grid pooler is set behind to constrain the outlier points. Experimental results demonstrate that LGABL has the capability to run a 4 K resolution image on a single RTX 3090 GPU shader with 24G RAM in real time, and our model exhibits favorable performance compared to state-of-the-art methods on various benchmarks.
更多查看译文
关键词
Multi-exposure image fusion,ultra high definition,global information,bilateral learning
AI 理解论文
溯源树
样例
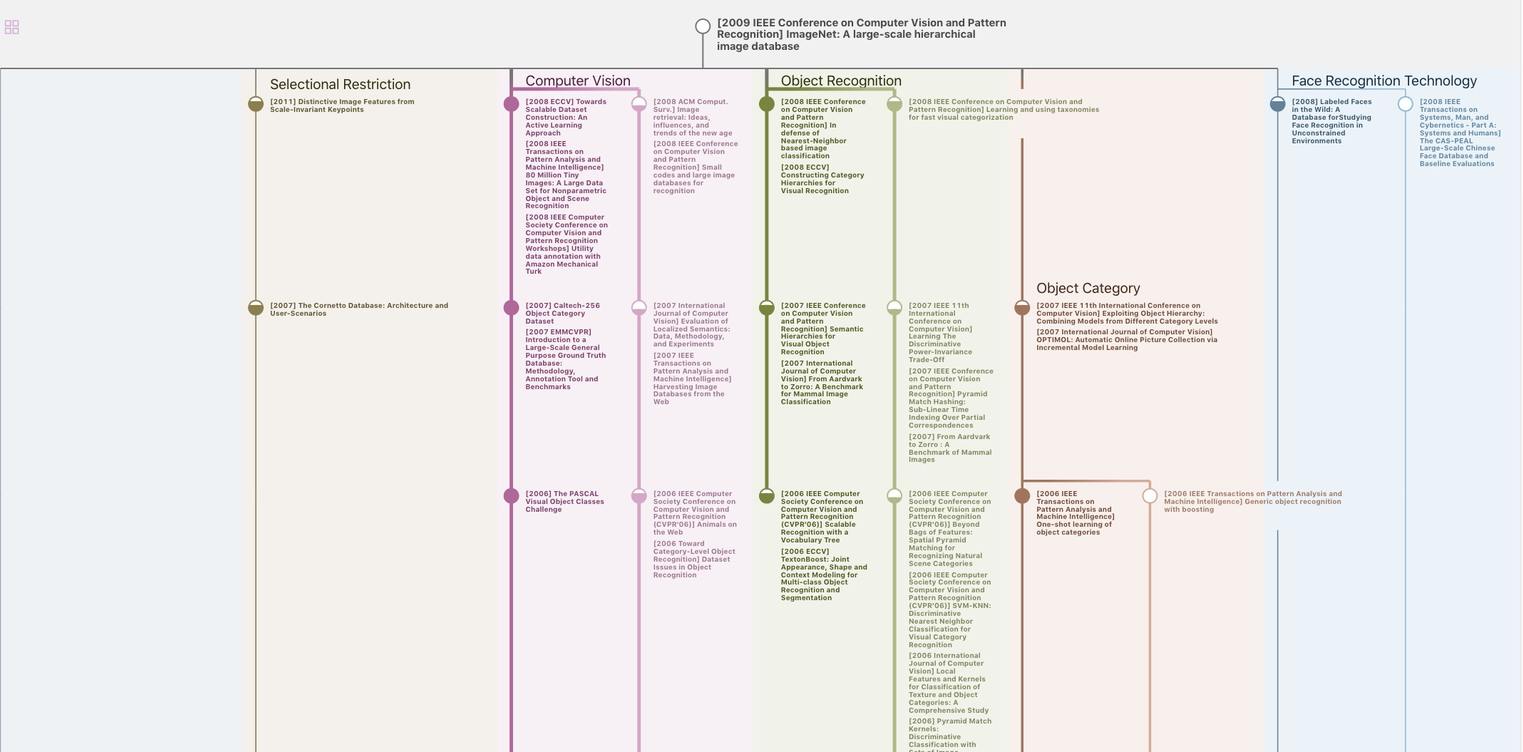
生成溯源树,研究论文发展脉络
Chat Paper
正在生成论文摘要