Multi-fidelity data-driven design and analysis of reactor and tube simulations
COMPUTERS & CHEMICAL ENGINEERING(2023)
摘要
Optimizing complex reactor geometries is vital to promote enhanced efficiency. We present a framework to solve this nonlinear, computationally expensive, and derivative-free problem. Gaussian processes are used to learn a multi-fidelity model of reactor simulations correlating multiple continuous mesh fidelities. The search space of reactor geometries is explored through lower fidelity simulations, evaluated based on a weighted acquisition function, trading off information gain with cost. Within our framework, DARTS, we derive a novel criteria for dictating optimization termination, ensuring a high fidelity solution is returned before budget is exhausted. We investigate the design of helical-tube reactors under pulsed-flow conditions, which have demonstrated outstanding mixing characteristics. To validate our results, we 3D print and experimentally validate the optimal reactor geometry, confirming mixing performance. Our approach is applicable to a broad variety of expensive simulation-based optimization problems, enabling the design of novel parameterized chemical reactors.
更多查看译文
关键词
Bayesian optimization, Data-driven optimization, Multi-fidelity, Computational fluid dynamics, Reactor design, Additive manufacturing
AI 理解论文
溯源树
样例
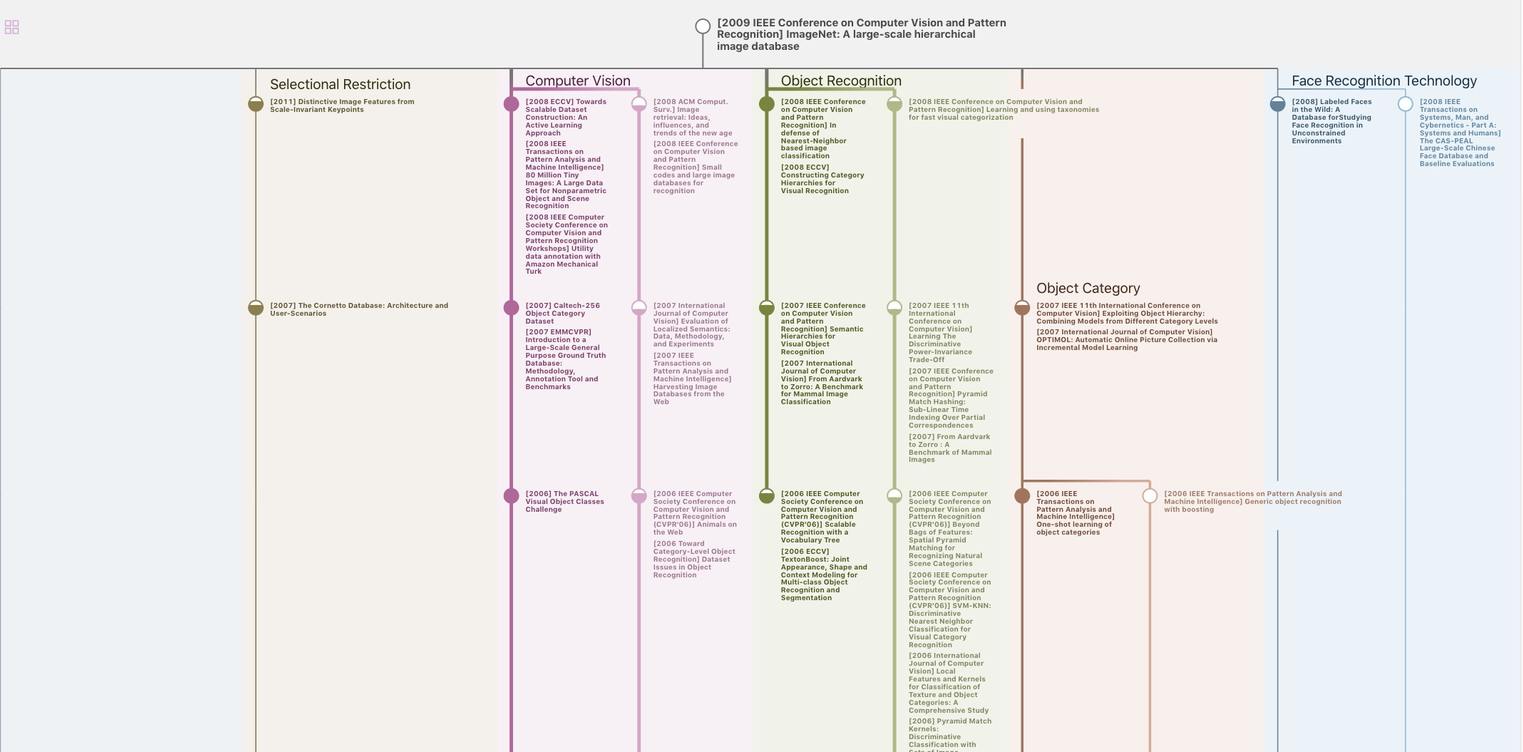
生成溯源树,研究论文发展脉络
Chat Paper
正在生成论文摘要