Mechanical properties prediction of ferrite ductile iron via machine learning
PHYSICA SCRIPTA(2023)
摘要
The rapid development of the wind power industry requires ductile iron to maintain high toughness while improving strength. Machine learning offers the possibility to accelerate various aspects of material development and performance optimization. In this paper, we established a composition-property dataset for ferritic ductile iron, and a variety of machine learning algorithms are compared to construct a composition-property model for ferritic ductile iron finally. The composition is chosen as the model input, and the outputs are four properties: tensile strength, Brinell hardness, yield strength and elongation. The correlation coefficients of the models constructed are above 0.96, and the mean absolute percentage errors are below 5%. The mean relative error between the model predictions and the experimental values is 4.43%, which effectively verifies the reliability of the composition-properties model of ductile iron constructed. This paper also uses the machine learning model to predict the effect law of each element content on the mechanical properties of ductile iron, and uses thermodynamic prediction of phase composition to verify the reliability of the machine learning method to predict the mechanical properties of ductile iron, which provides strong guidance for the design of new ductile iron with high strength and high plasticity at room temperature.
更多查看译文
关键词
ferrite ductile iron,mechanical properties prediction,machine learning,mechanical properties
AI 理解论文
溯源树
样例
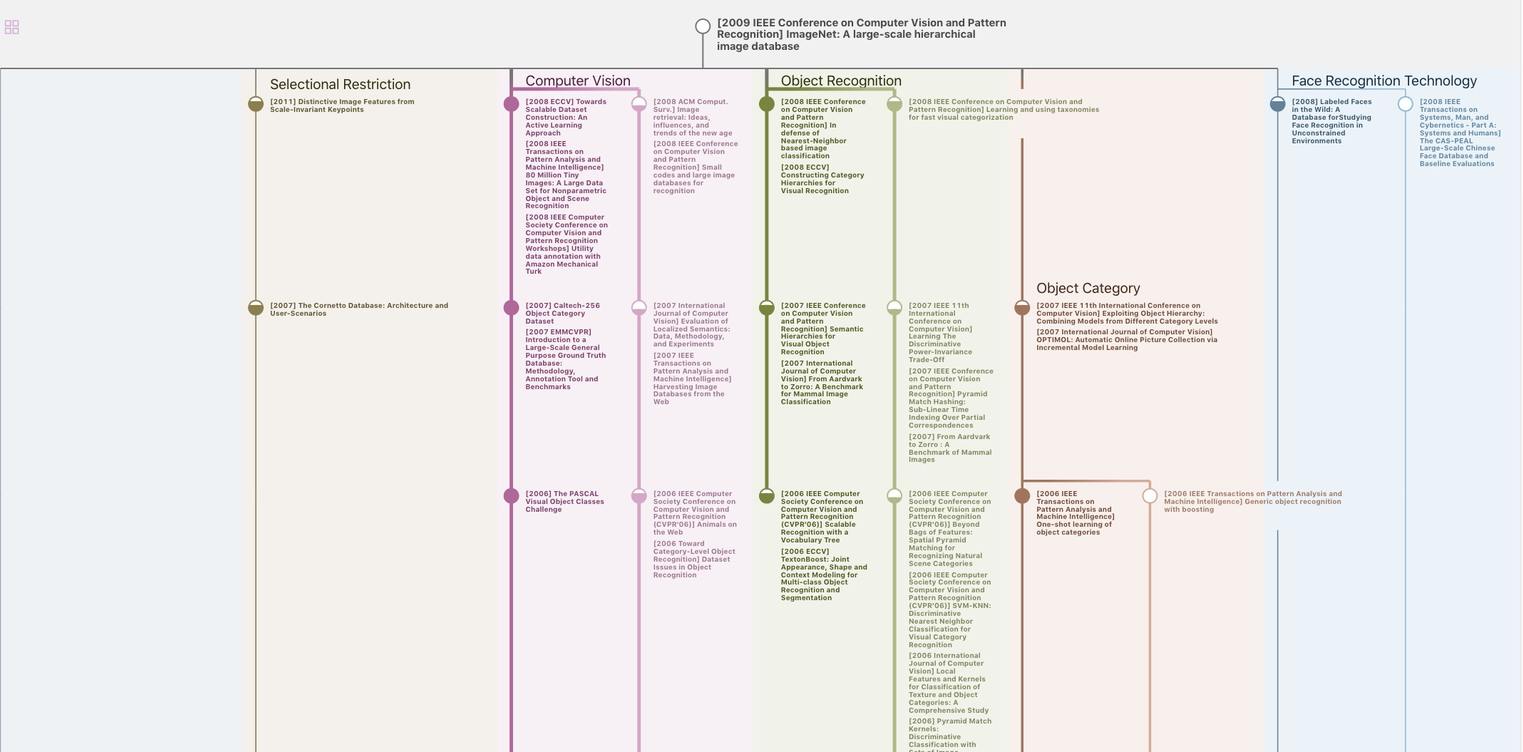
生成溯源树,研究论文发展脉络
Chat Paper
正在生成论文摘要