Long-term capacity fade forecasting of vanadium redox flow battery with Gaussian process regression combined with informer model
JOURNAL OF POWER SOURCES(2023)
Abstract
To resolve the long sequence time-series forecasting problem of a battery, this study introduces the Gaussian process regression (GPR) model combined with the Informer model, namely I-GPR model. The Informer model is used to predict the future features of the battery capacity fade according to the experimental features, which are extracted through a user-defined convolution kernel from the actual voltage, current, temperature, and electrolyte flowrate of the battery. In the GRP model, the capacity fade, which is considered to follow a Gaussian distribution, is characterized as a joint Gaussian distribution. By expressing the distribution under the training datasets from the experimental features, the test datasets are obtained from the predicted features as the distribution input to regress the capacity fade with high fidelity at different cycle lengths and current densities. Compared with the experimental result, the proposed model revealed prediction average errors of 0.66%, 1.93%, 1.71% with the initial state of health (SOH) of 100%, and 0.50%, 1.58%, 2.01% with the initial SOH of 85%, and average root-mean-square error of 0.85%, 2.51%, 2.04% with the initial SOH of 100%, and 0.60%, 1.96%, 2.37% with the initial SOH of 85% at current densities of 80, 120, and 160 mA cm−2, respectively.
MoreTranslated text
Key words
Vanadium redox flow battery,Capacity fade,Forecasting,Informer model,Gaussian process regression
AI Read Science
Must-Reading Tree
Example
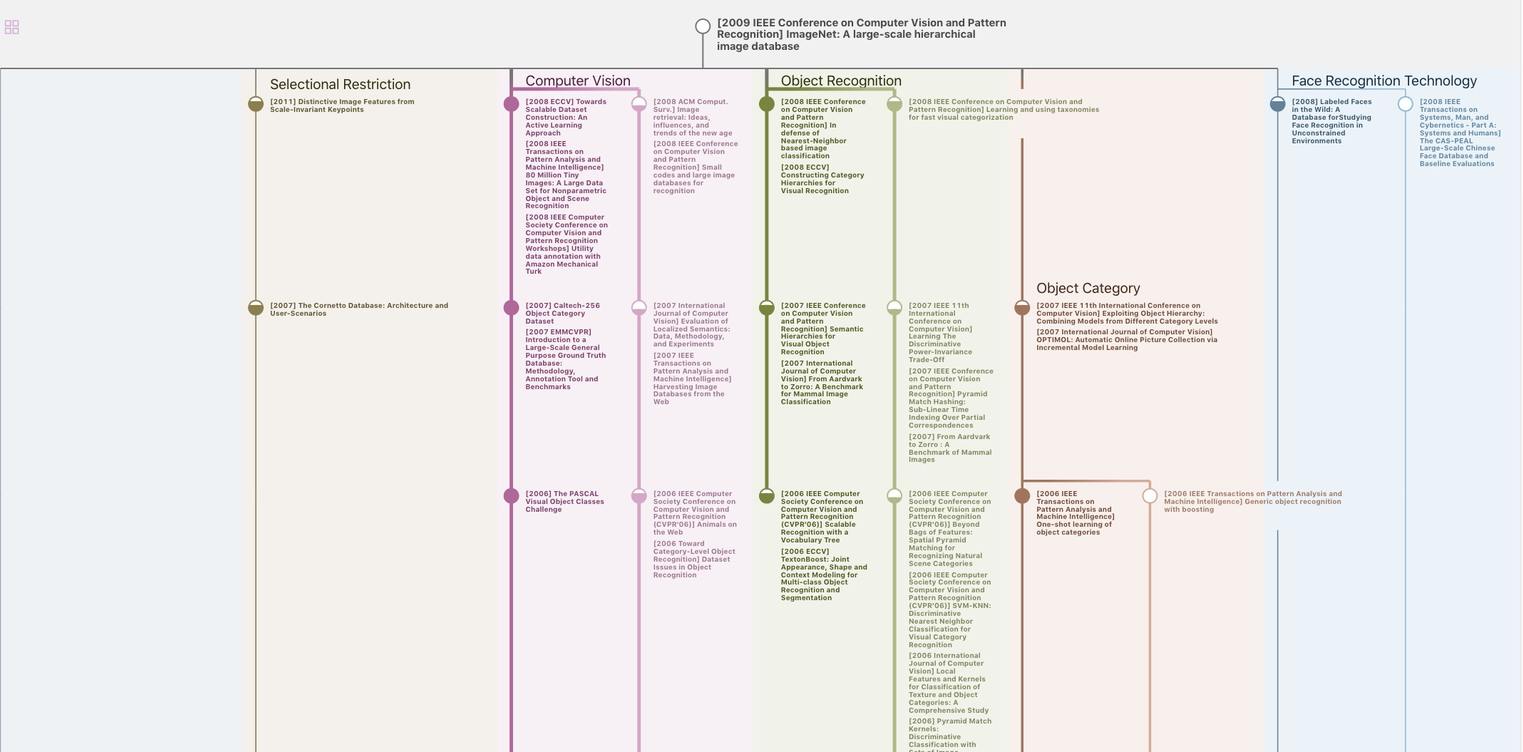
Generate MRT to find the research sequence of this paper
Chat Paper
Summary is being generated by the instructions you defined