Complex PQD Classification Using Time-Frequency Analysis and Multiscale Parallel Attention Residual Network
IEEE TRANSACTIONS ON INDUSTRIAL ELECTRONICS(2023)
摘要
Accurate identification of complex power quality disturbances (PQDs) is highly important for the pollution control of modern power systems. However, the occurrence of nonlinear loads causes the grid signals distorted and unstable, resulting in the difficulty of efficient classification. This article aims to develop an effective detection method for complex PQD automatic classification. First, a modified Kaiser window-based S-Transform (MKS) is proposed for converting the PQD time-series signals to time-frequency feature matrix, where the time-frequency performance of MKS can be improved through setting different window control functions in low-frequency and high-frequency parts, respectively. Next, a multiscale parallel attention residual network (MPARN) is presented to extract and classify disturbance information based on the optimized residual structure. Integrating MKS and MPARN, an automatic classification framework is further proposed to identify various PQDs. Simulation and hardware platform experiments demonstrate that our classification strategy can obtain superior performance than several state-of-the-art methods for complex even nonlinear PQD identification under different noise levels.
更多查看译文
关键词
Attention mechanism,convolutional neural network (CNN),power quality disturbances (PQD),residual networks (ResNets),time-frequency analysis
AI 理解论文
溯源树
样例
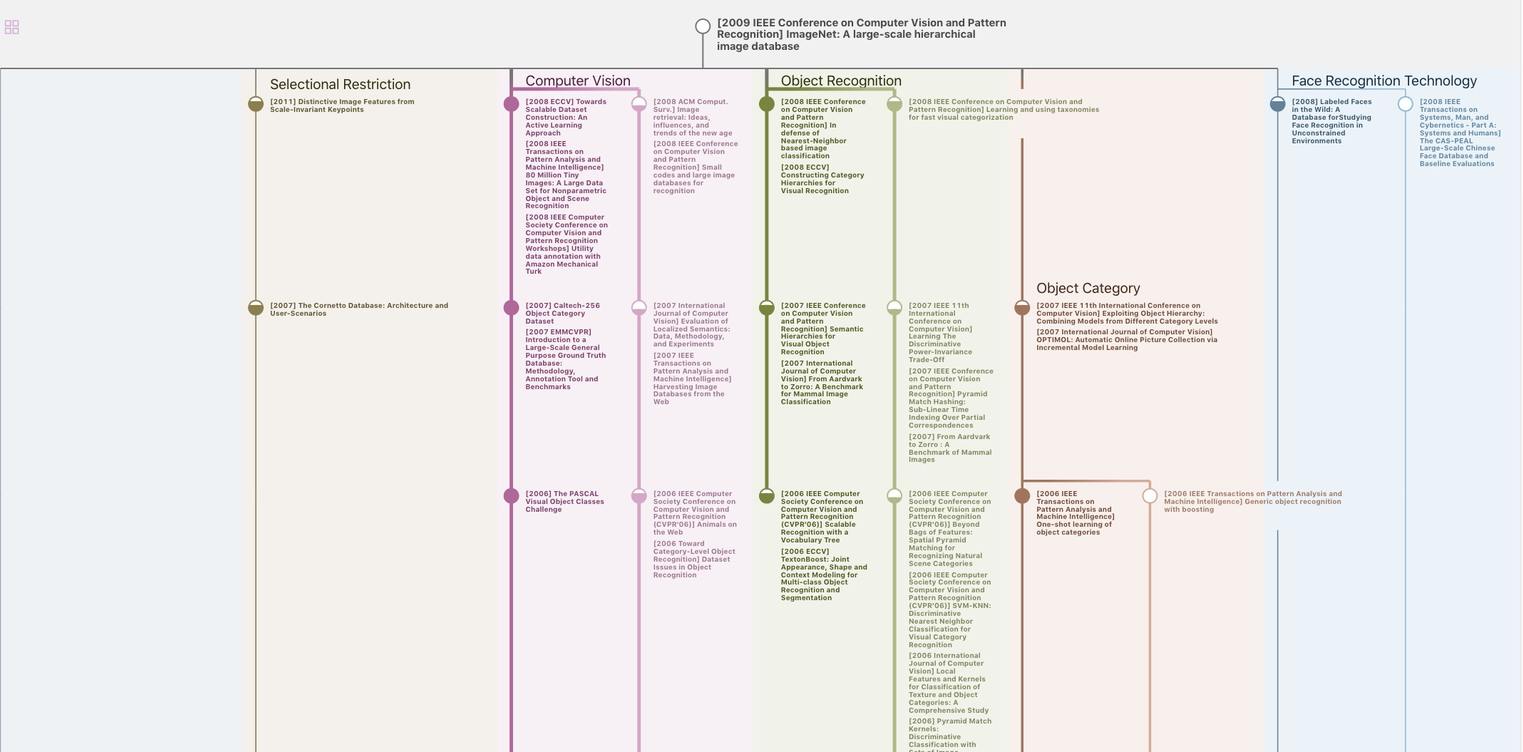
生成溯源树,研究论文发展脉络
Chat Paper
正在生成论文摘要