Enhancing gene expression prediction in major psychiatric disorders via co-expression models
EUROPEAN NEUROPSYCHOPHARMACOLOGY(2023)
摘要
Imputed gene expression based on common variants is instrumental to understanding the biology of psychiatric risk. By identifying trans-eQTLs via co-expression across different brain regions, we aimed to generate a platform for Transcriptome-Wide Association Studies with enhanced gene expression imputation, particularly for genes depleted for cis-eQTLs. We developed two prediction models derived from trans-eQTLs: i) the “Imputed Network Gene-Expression trans-eQTL” (INGENE), which exploits imputed co-expression partners to predict the expression of each target gene, and ii) the “Module Quantitative Trait Loci Eigengene” (MODULE) model, which generates polygenic scores associated with the eigenvalue of the co-expression module as a predictor of single-gene expression. We trained INGENE and MODULE on genotype and gene expression data of the Lieber Institute for Brain Development from tissue homogenate RNA-sequencing in the dorsolateral prefrontal cortex (DLPFC) (N=593), amygdala (N=463), hippocampus (N=237), caudate (N=214) and subgenual anterior cingulate cortex (sACC) (N = 512 EA). We included adults of European Ancestry (EA) (mean ages across regions: 45.9-49.6 years; female ratios across regions: 23%-31%). We trained the models on 25 published co-expression networks and 4 brain regions using the elastic net algorithm with 10-fold cross-validation (CV). We retained models if the average Pearson correlation between predicted and observed values during CV exceeded 0.1 (rCV > 0.1). In the testing phase, we applied the cis-based EpiXcan, INGENE and MODULE models to the Genotype-Tissue Expression (GTEx) cohort (DLPFC: 191, amygdala: 133, hippocampus: 182, caudate: 250, and ACC: 165) (age across regions: 20 – 79 years; female ratios across regions: 26%-30%). For each gene, we averaged the network-derived predictions and compared imputed and observed expression values using a linear model. Genes with opposite directionality between predicted and observed values were excluded. We then compared the performance of EpiXcan, INGENE and MODULE. Prediction models performed similarly across brain regions, EpiXcan explained an average variance of 2.6%-3.4% for 7,545-8,154 genes, while INGENE explained approximately 1% for 7357–7743 genes, and MODULE explained 2%-3% across 12,872-12,987 genes. The three techniques predicted 3,628-4,175 genes in common, with the majority of these genes being predicted by EpiXcan. However, INGENE and MODULE generated a large number of predictions, albeit with lower explained variance. Notably, 3,000-3,472 predicted genes overlapped between the trans-models across different brain regions. These findings highlight the considerable potential for combining cis- and trans-predictions to enhance predictive power, and effectively expand the number of imputed genes that can be deemed heritable with remarkable across-regions consistency. Our approach is promising to enhance Transcriptome-Wide Association Studies for schizophrenia, bipolar disorder, and major depression.
更多查看译文
关键词
gene expression prediction,gene expression,psychiatric disorders,co-expression
AI 理解论文
溯源树
样例
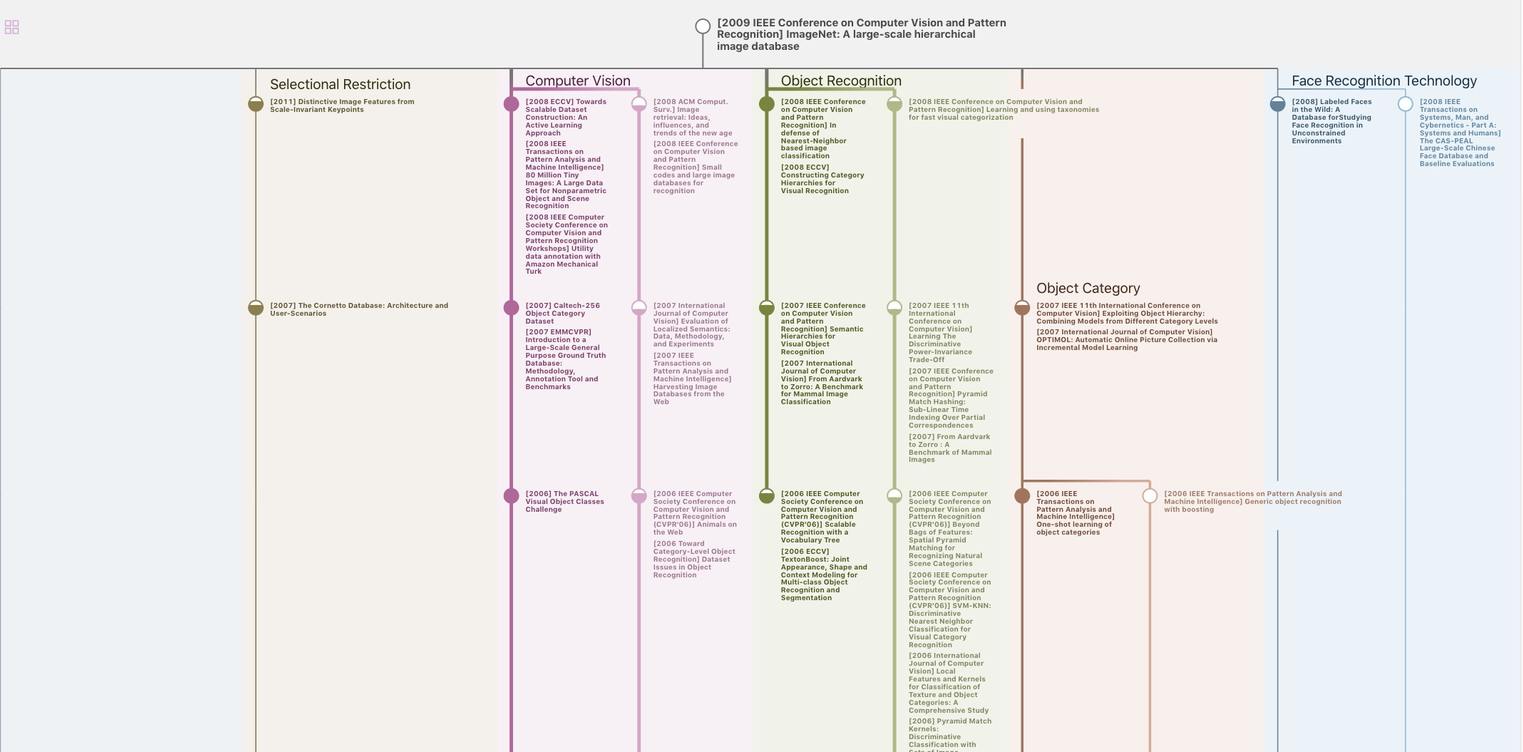
生成溯源树,研究论文发展脉络
Chat Paper
正在生成论文摘要