Weighted Order-p Tensor Nuclear Norm Minimization and Its Application to Hyperspectral Image Mixed Denoising
IEEE GEOSCIENCE AND REMOTE SENSING LETTERS(2023)
摘要
Recently, tensor singular value decomposition (t-SVD) has demonstrated excellent performance in various high-dimensional information processing applications. However, in adapting t-SVD to handle the typical tensor data restoration tasks, such as hyperspectral image (HSI) denoising, the following questions remain inadequately addressed: 1) the existing tensor nuclear norm minimization (TNN) regime treats all tensor singular values alike; thus, it lacks flexibility and dominance in dealing with the sophisticated HSI tensor; 2) the existing tSVD-based denoising methods cannot directly process order -p (p > 3) tensors; thus, they fail to comprehensively exploit the high-dimensional structural correlation of the HSI tensor along different modes. To address the above challenges, in this study, we first generalize a novel weighted order -p TNN minimization regime, which integrates the adaptively reweighting strategy for matrix, third-order, and order -p tensors in a unified architecture. Subsequently, an efficient subspace low-rank learning model is established, using HSI denoising tasks as an application example to corroborate the superiority of the proposed regime in approximating the high-dimensional low-rank structure of natural tensor data. Extensive experimental results substantiate that our effort surpasses existing state-of-the-art low-rank tensor recovery methods in both restoration accuracy and efficiency.
更多查看译文
关键词
Tensors, Minimization, Noise reduction, Transforms, Task analysis, Matrix decomposition, Geoscience and remote sensing, High-order tensor nuclear norm (TNN), hyperspectral image (HSI) denoising, low-rank tensor approximation, reweighting strategy
AI 理解论文
溯源树
样例
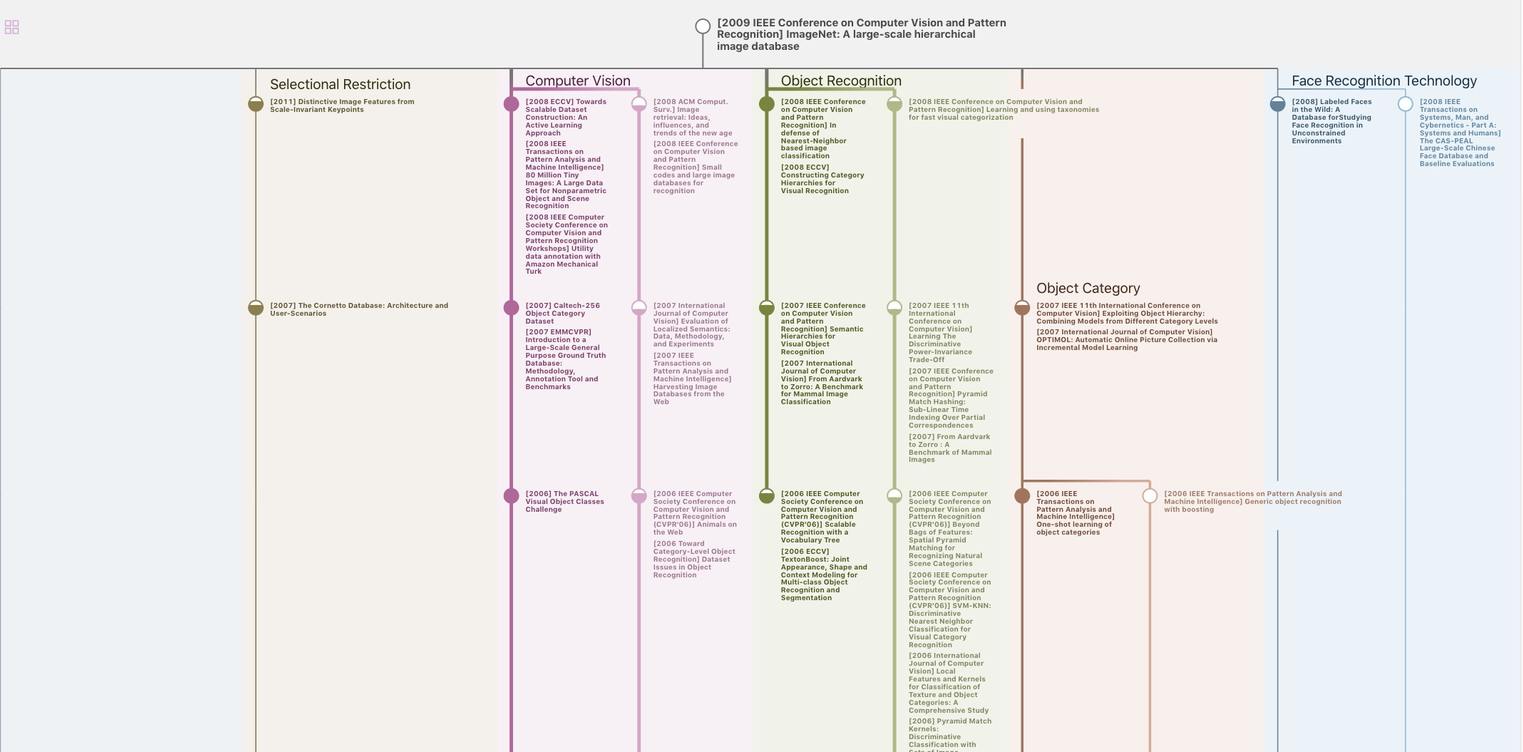
生成溯源树,研究论文发展脉络
Chat Paper
正在生成论文摘要