A Novel Active-Learning Kriging Reliability Analysis Method Based on Parallelized Sampling Considering Budget Allocation
IEEE TRANSACTIONS ON RELIABILITY(2023)
摘要
Active-learning Kriging models have gained more and more popularity for structural reliability analysis (SRA) in recent years. Improving the efficiency of simulations while maintaining high accuracy is essential for building Kriging-based SRA approaches. In this article, we propose a novel active-learning Kriging reliability analysis method based on parallelized sampling considering budget allocation (ALK-PBA). A parallelized sampling strategy based on clustering and budget allocation is developed. The low-discrepancy candidate samples are employed to provide representative candidate samples, and the adaptive truncated region is applied to select samples of relative high probability density. Then, the method is used for clustering samples. After identifying clusters, ALK-PBA allocates new training samples to each cluster according to a chosen learning function. This process is repeated iteratively to renew the Kriging model. Several numerical cases have been evaluated using the proposed ALK-PBA, and the results have demonstrated its high accuracy and efficiency for SRA. Moreover, the proposed method is employed to simulate three performance functions of different dimensions under various learning functions, and recommendations for choosing learning functions for the discussed problems are provided in this article.
更多查看译文
关键词
Adaptive truncated regions,budget allocation,density-based spatial clustering of applications with noise (DBSCAN),Kriging,low-discrepancy sampling,reliability analysis
AI 理解论文
溯源树
样例
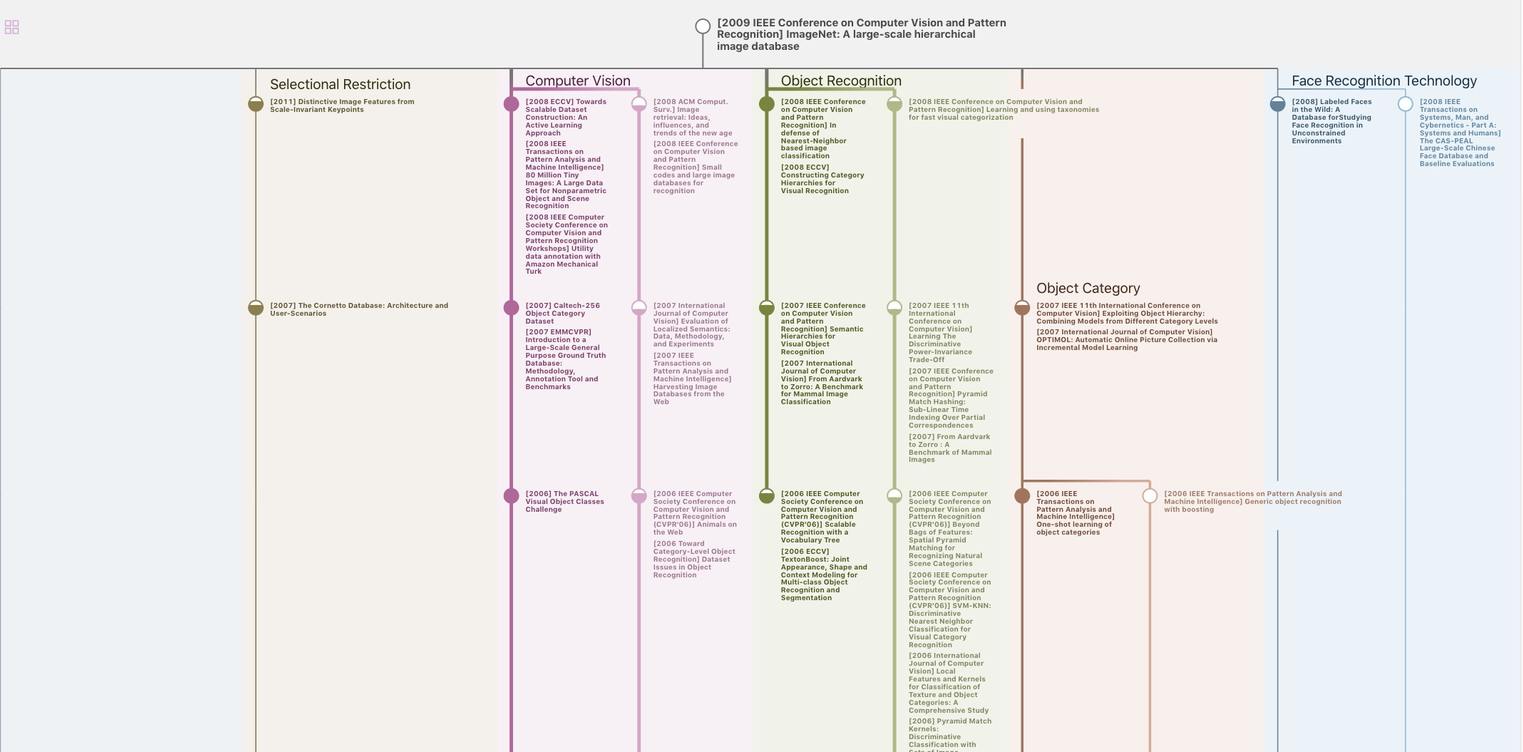
生成溯源树,研究论文发展脉络
Chat Paper
正在生成论文摘要