High or low? Exploring the restorative effects of visual levels on campus spaces using machine learning and street view imagery
URBAN FORESTRY & URBAN GREENING(2023)
摘要
According to the Attentional Restoration Theory (ART), cognitive restoration (e.g., Fascination) may occur when the physical environment exhibits high restorative quality. However, these studies usually ignore the effect of different levels of visual features on restoration quality, and small-scale questionnaires are difficult to use to comprehensively evaluate the restoration quality of a space. In this study, we propose a machine learning based method for high-resolution, large-scale assessment of the restoration quality of campus environments using Street View Images (SVIs). First, visual features are extracted from campus SVIs using computer vision method. Second, an online survey using the PRS-11 questionnaire (containing four indicators: Being-away, Coherence, Scope, and Fascination) was conducted to label the images. Finally, we developed a regression model to predict campus restorative quality and to model the non-linear relationship between the visual features of SVIs and this quality. We studied 1088 SVIs in the Lihu campus of Jiangnan University (JNU) to verify the feasibility of our method, and the results showed that SVIs can accurately help us predict the restoration quality of the campus environment on a large scale (R2 = 0.726). Next, we examined the variance in visual features between campus spaces with different levels of restorative quality, and investigated the effect of different levels of visual features on restoration quality. We found that contributions of high-level visual features to restoration, such as trees, are robust (Adj R2 = 0.504) compared to low-level visual features (Adj R2 = 0.032) that included such as color information. This provides a new perspective for assessing recovery environments and designing healthy campus environments. The code is shared at: https://github.com/MMHHRR/Restorative_Quality
更多查看译文
关键词
Restoration,Street view images,Machine learning,Healthy campus design,Attention restoration theory
AI 理解论文
溯源树
样例
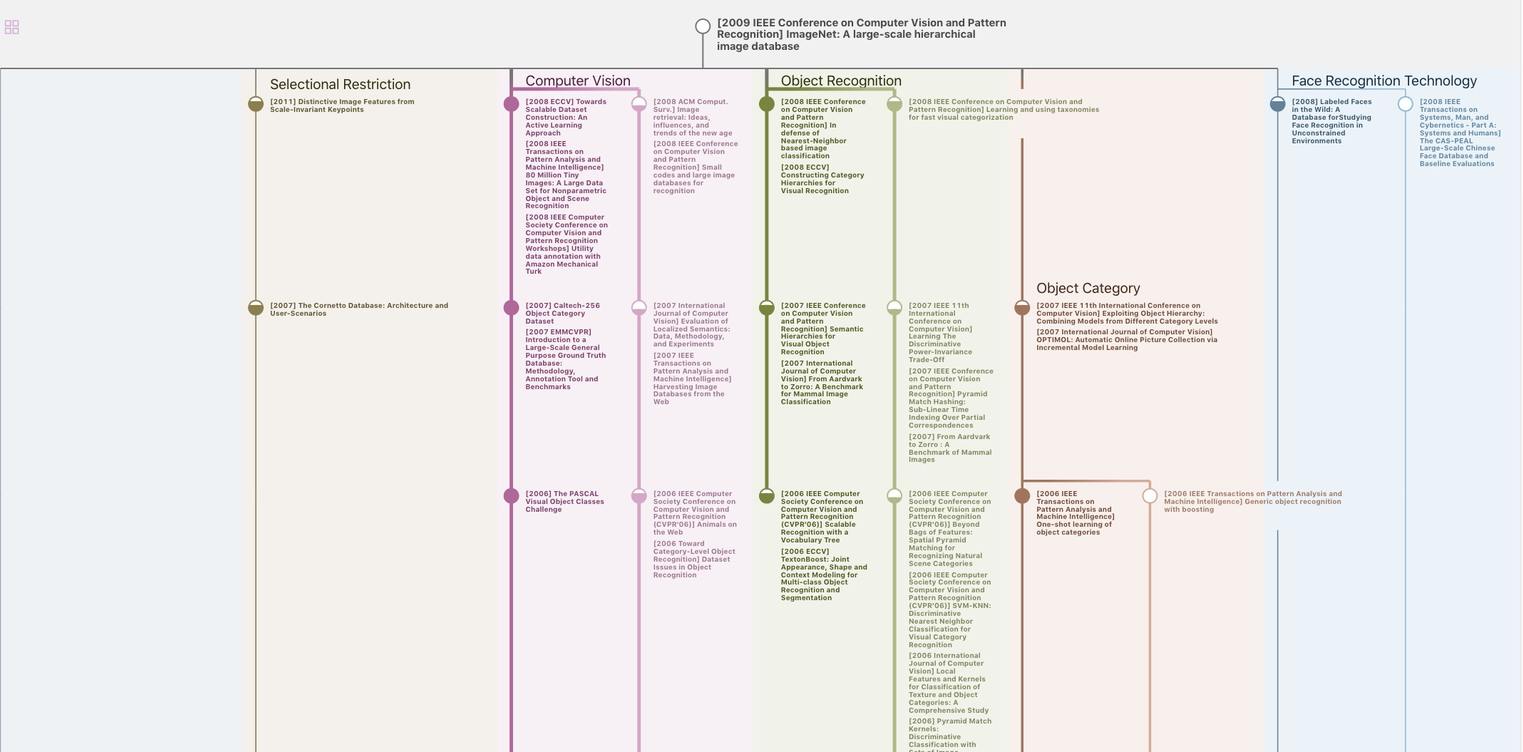
生成溯源树,研究论文发展脉络
Chat Paper
正在生成论文摘要