Online Learning Sample Filtering for Object Tracking
IEEJ TRANSACTIONS ON ELECTRICAL AND ELECTRONIC ENGINEERING(2024)
摘要
Object tracking tasks often face problems such as illumination and appearance changes due to various scenarios. To effectively solve such problems, single object tracking algorithms in recent years have improved the stability of the tracker by introducing online learning, reducing tracking drift, and tracking objects stably even with large deformations. However, on the one hand, employing online learning increases computation costs, causing the tracker to run slower. On the other hand, because it learns from the tracking results, the tracking template will only be correctly updated if the object is accurately tracked. Any incorrect update will result in tracking failure. In this paper, we propose a filtering algorithm for online learning sample processing in object tracking to remedy these issues. Our method can select valid training samples and discard unnecessary training samples in object tracking. Numerous experiments demonstrate that our algorithm improves the tracking accuracy, reduces the computation complexity, and improves the running speed and run-time. (c) 2023 Institute of Electrical Engineer of Japan and Wiley Periodicals LLC.
更多查看译文
关键词
object tracking,online learning,filter,template update
AI 理解论文
溯源树
样例
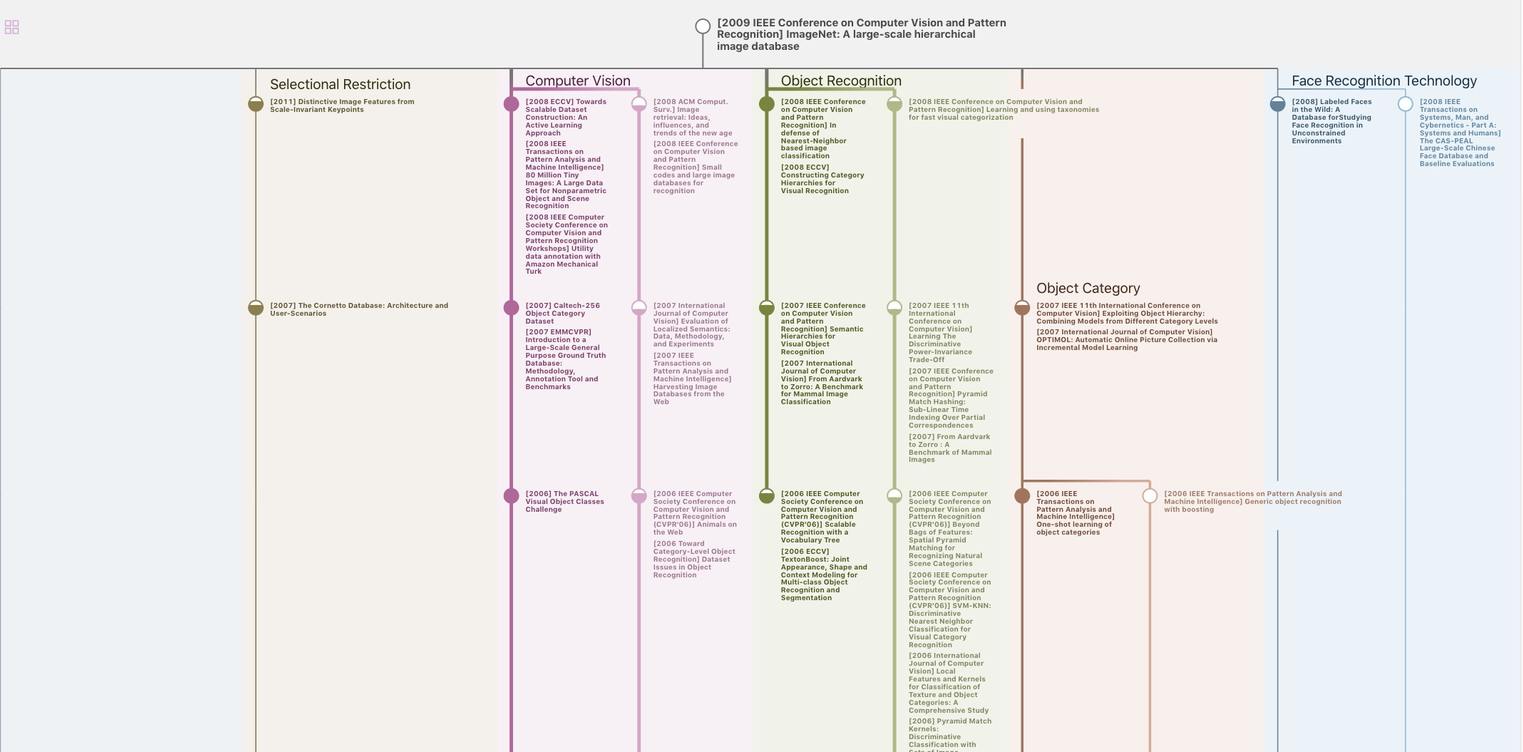
生成溯源树,研究论文发展脉络
Chat Paper
正在生成论文摘要