Development and internal-external validation of statistical and machine learning models for breast cancer prognostication: cohort study
BMJ-BRITISH MEDICAL JOURNAL(2023)
摘要
OBJECTIVE To develop a clinically useful model that estimates the 10 year risk of breast cancer related mortality in women (self-reported female sex) with breast cancer of any stage, comparing results from regression and machine learning approaches. DESIGN Population based cohort study. SETTING QResearch primary care database in England, with individual level linkage to the national cancer registry, Hospital Episodes Statistics, and national mortality registers. PARTICIPANTS 141 765 women aged 20 years and older with a diagnosis of invasive breast cancer between 1 January 2000 and 31 December 2020. MAIN OUTCOME MEASURES Four model building strategies comprising two regression (Cox proportional hazards and competing risks regression) and two machine learning (XGBoost and an artificial neural network) approaches. Internal-external cross validation was used for model evaluation. Random effects meta-analysis that pooled estimates of discrimination and calibration metrics, calibration plots, and decision curve analysis were used to assess model performance, transportability, and clinical utility. RESULTS During a median 4.16 years (interquartile range 1.76-8.26) of follow-up, 21 688 breast cancer related deaths and 11 454 deaths from other causes occurred. Restricting to 10 years maximum follow-up from breast cancer diagnosis, 20 367 breast cancer related deaths occurred during a total of 688 564.81 person years. The crude breast cancer mortality rate was 295.79 per 10 000 person years (95% confidence interval 291.75 to 299.88). Predictors varied for each regression model, but both Cox and competing risks models included age at diagnosis, body mass index, smoking status, route to diagnosis, hormone receptor status, cancer stage, and grade of breast cancer. The Cox model's random effects meta-analysis pooled estimate for Harrell's C index was the highest of any model at 0.858 (95% confidence interval 0.853 to 0.864, and 95% prediction interval 0.843 to 0.873). It appeared acceptably calibrated on calibration plots. The competing risks regression model had good discrimination: pooled Harrell's C index 0.849 (0.839 to 0.859, and 0.821 to 0.876, and evidence of systematic miscalibration on summary metrics was lacking. The machine learning models had acceptable discrimination overall (Harrell's C index: XGBoost 0.821 (0.813 to 0.828, and 0.805 to 0.837); neural network 0.847 (0.835 to 0.858, and 0.816 to 0.878)), but had more complex patterns of miscalibration and more variable regional and stage specific performance. Decision curve analysis suggested that the Cox and competing risks regression models tested may have higher clinical utility than the two machine learning approaches. CONCLUSION In women with breast cancer of any stage, using the predictors available in this dataset, regression based methods had better and more consistent performance compared with machine learning approaches and may be worthy of further evaluation for potential clinical use, such as for stratified follow-up.
更多查看译文
关键词
breast cancer prognostication,breast cancer,machine learning models,machine learning,internal-external
AI 理解论文
溯源树
样例
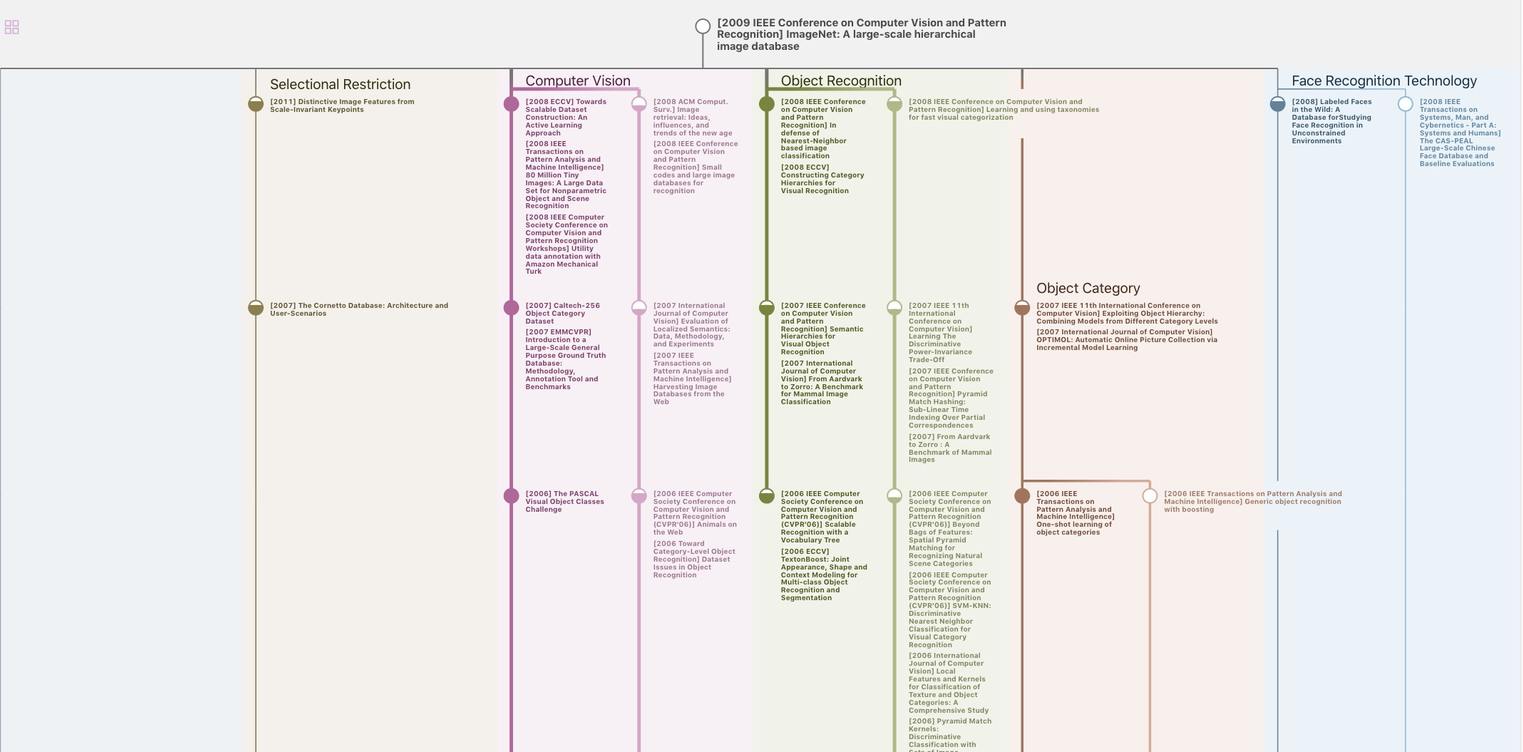
生成溯源树,研究论文发展脉络
Chat Paper
正在生成论文摘要