Graph based embedding learning of trajectory data for transportation mode recognition by fusing sequence and dependency relations
INTERNATIONAL JOURNAL OF GEOGRAPHICAL INFORMATION SCIENCE(2023)
Abstract
As an important task in spatial data mining, trajectory transportation mode recognition can reflect various individual behaviors and traveling patterns in urban space. As trajectory is essentially a sequence, many scholars use the sequence inference models to mine the information in trajectory data. However, such methods often ignored the spatial correlation between trajectory points and implemented the evaluation based only on representative feature statistics selected in the trajectory data preprocessing stage, thus have difficulties in acquiring high-order traveling pattern features. In this study, we propose a novel ensemble recognition method for representing trajectory data with the graph structure based on sequence and dependency relations. This method integrates the sequence of trajectory points and the correlation between characteristic points of a travel path into a fused graph convolutional network to obtain semantic feature information at multiple levels. We validate our proposed method with experiments on the trajectory benchmark dataset from the Microsoft GeoLife project. The results demonstrated that our proposed graph network outperforms other baseline methods in the transportation mode recognition task of trajectories. This method can help to discover the movement patterns of urban residents, and further provide effective assistance for the management of cities.
MoreTranslated text
Key words
transportation mode recognition,trajectory data,graph
AI Read Science
Must-Reading Tree
Example
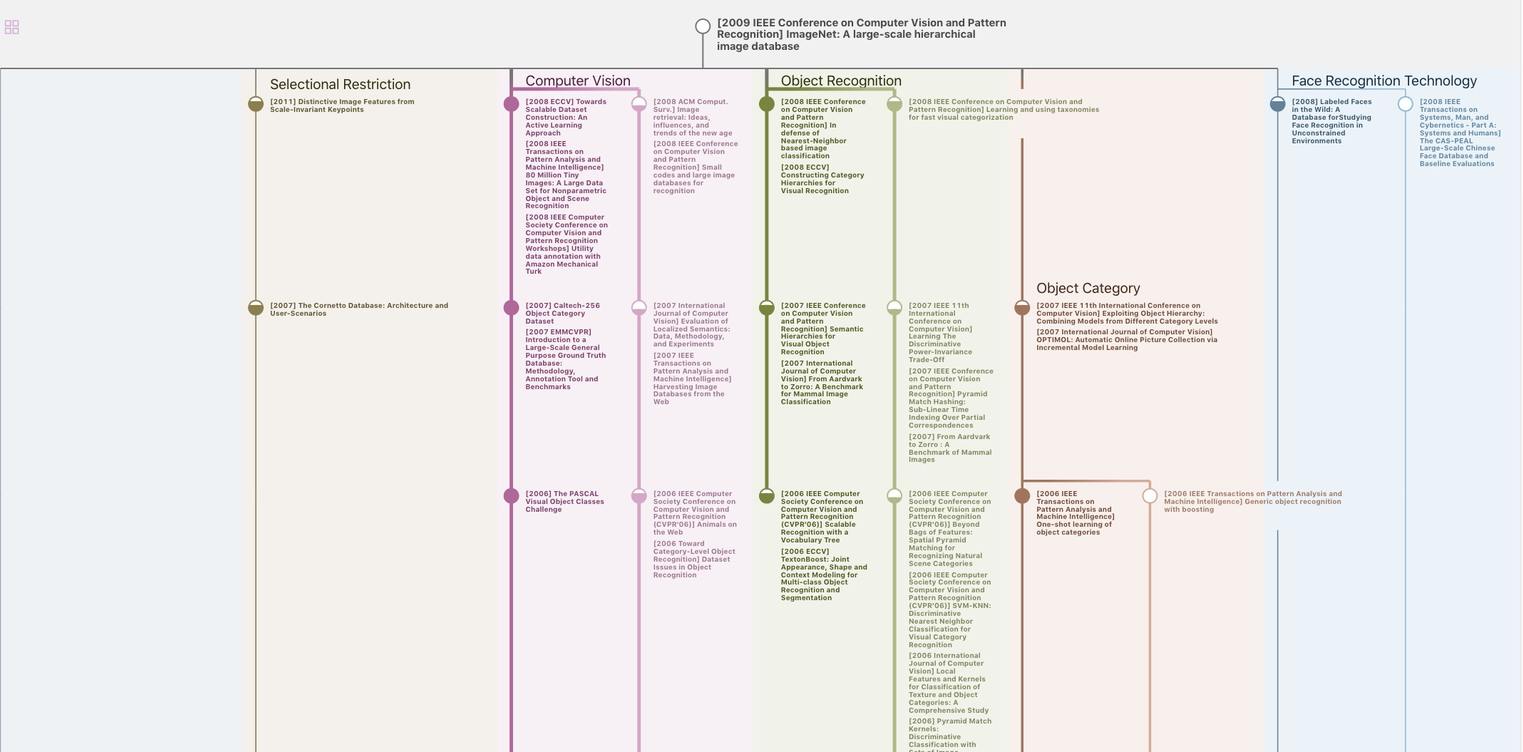
Generate MRT to find the research sequence of this paper
Chat Paper
Summary is being generated by the instructions you defined