Social media and social impact assessment: Evolving methods in a shifting context
CURRENT SOCIOLOGY(2023)
Abstract
Among many by-products of Web 2.0 come the wide range of potential image and text datasets within social media and content sharing platforms that speak of how people live, what they do, and what they care about. These datasets are imperfect and biased in many ways, but those flaws make them complementary to data derived from conventional social science methods and thus potentially useful for triangulation in complex decision-making contexts. Yet the online environment is highly mutable, and so the datasets are less reliable than censuses or other standard data types leveraged in social impact assessment. Over the past decade, we have innovated numerous methods for deploying Instagram datasets in investigating management or development alternatives. This article synthesizes work from three Canadian decision contexts - hydroelectric dam construction or removal; dyke realignment or wetland restoration; and integrating renewable energy into vineyard landscapes - to illustrate some of the methods we have applied to social impact assessment questions using Instagram that may be transferrable to other social media platforms and contexts: thematic (manual coding, machine vision, natural language processing/sentiment analysis, statistical analysis), spatial (hotspot mapping, cultural ecosystem modeling), and visual (word clouds, saliency mapping, collage). We conclude with a set of cautions and next steps for the domain. Parmi les nombreux sous-produits du Web 2.0 figure un large eventail de donnees provenant d'images et de textes, de contenus de medias sociaux et de plateformes numeriques, qui revelent comment les gens vivent, ce qu'ils font et les questions qui les preoccupent. Ces ensembles de donnees sont imparfaits et biaises a bien des egards, mais nombre de leurs lacunes les rendent complementaires des informations collectees par les sciences sociales a l'aide de methodes conventionnelles. D'ou leur utilite potentielle pour la triangulation dans des contextes decisionnels complexes. Cet article synthetise le travail de trois etudes de cas menees au Canada pour illustrer certaines des methodes que nous avons developpees et qui pourraient etre utiles a d'autres chercheurs en EIS: thematiques (codage, apprentissage automatique, analyse semantique, association statistique), spatiales (cartographie des points chauds, modelisation du transfert des benefices) et visuelles (cartes de saillance, collage). Entre los muchos subproductos de la Web 2.0 se encuentra una amplia gama de datos de imagenes y texto, contenidos en redes sociales y plataformas digitales, que hablan de como vive, que hace y por que cuestiones se preocupa la gente. Estos conjuntos de datos son imperfectos y sesgados en muchos sentidos, pero muchos de sus defectos los hacen complementarios a la informacion recogida por las ciencias sociales con metodos convencionales. De ahi su potencial utilidad para la triangulacion en contextos complejos de toma de decisiones. Este articulo sintetiza el trabajo de tres estudios de caso llevados a cabo en Canada para ilustrar algunos de los metodos que hemos desarrollado y pueden resultar utiles para otros investigadores en EIS: tematicos (codificacion, machine learning, analisis semantico, asociacion estadistica), espaciales (mapeo de puntos criticos, modelizacion de transferencia de beneficios) y visuales (mapas de saliencia, collage).
MoreTranslated text
AI Read Science
Must-Reading Tree
Example
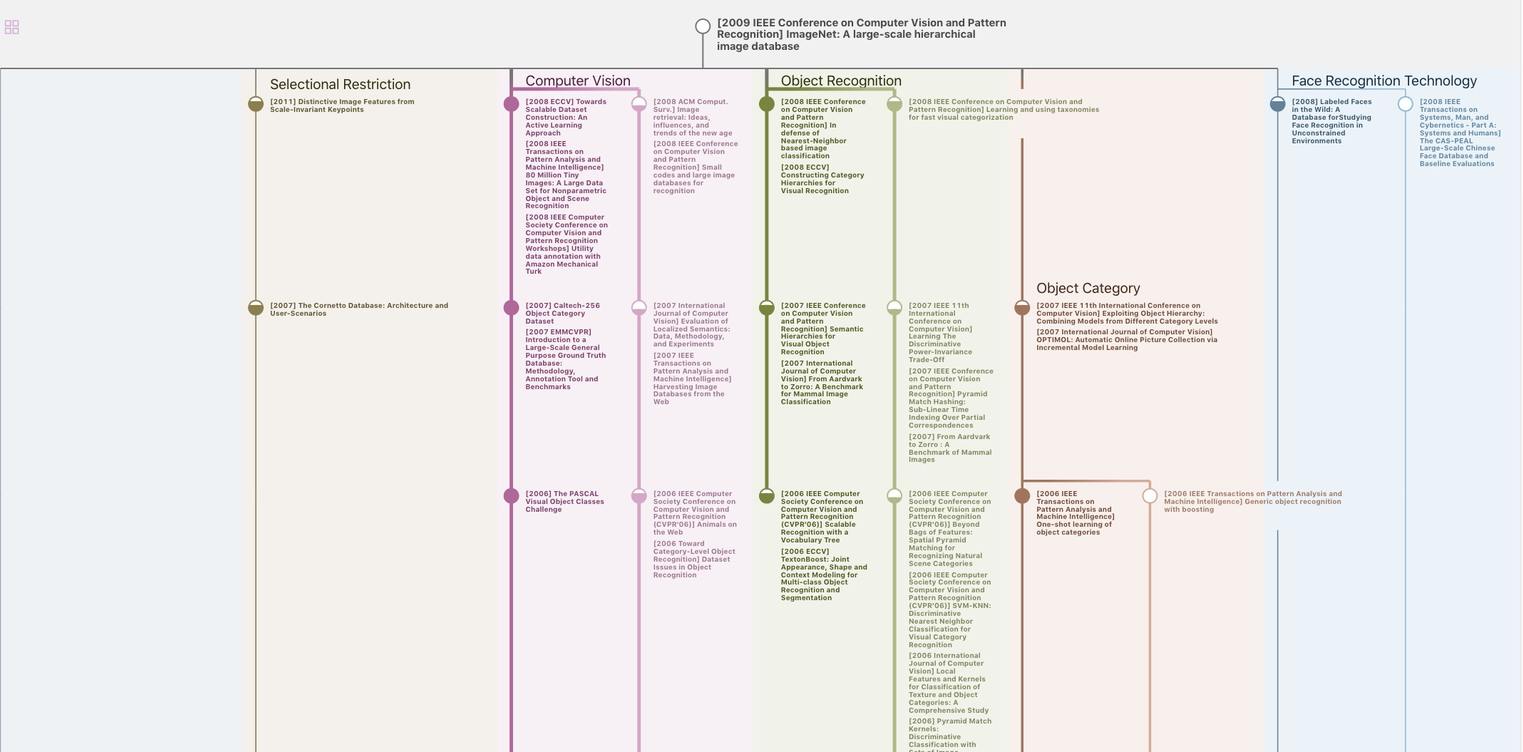
Generate MRT to find the research sequence of this paper
Chat Paper
Summary is being generated by the instructions you defined