Highly dynamic X-ray image enhancement based on generative adversarial network
JOURNAL OF INSTRUMENTATION(2023)
摘要
When using X-ray to detect industrial workpieces, the images obtained frequently have low contrast, making it difficult to detect defects. This paper proposes a GAN-based X-ray image enhancement network to address this issue. In detail, the X-ray image is concatenated with its antiphase image (as an exposure mask) as the input image, and a trainable Sobel operator is used to extract the edge features of the input image. The input image and edge features are then concatenated and fed into the U-Net generator to be enhanced. The spatial and channel attention models are used to adjust feature weights in U-Net, and a detail extraction network is designed to extract detail features from the input X-ray image. Furthermore, the extracted detail features are fused with the image by the generator after contrast stretching to produce the final enhanced image. Finally, a global-local discriminator is used to discriminate the authenticity of the image so that the contrast of the final obtained image is improved and the details are highlighted. Following experimental validation, the method proposed in this paper has a significant enhancement effect on industrial X-ray images and performs well in terms of enhancing image contrast and highlighting image details.
更多查看译文
关键词
Detection of defects, Inspection with x-rays
AI 理解论文
溯源树
样例
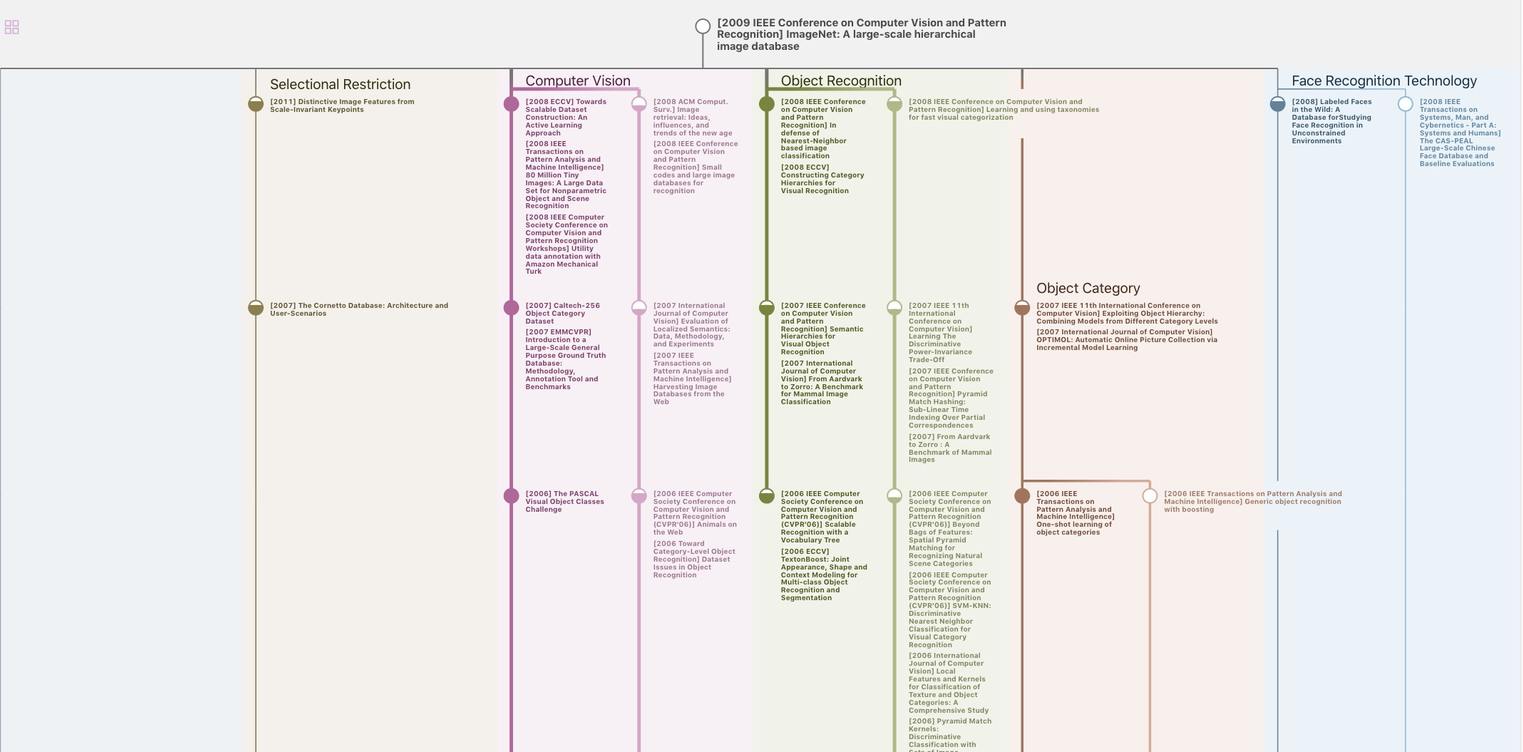
生成溯源树,研究论文发展脉络
Chat Paper
正在生成论文摘要