Camouflage Backdoor Attack against Pedestrian Detection
APPLIED SCIENCES-BASEL(2023)
摘要
Pedestrian detection models in autonomous driving systems heavily rely on deep neural networks (DNNs) to perceive their surroundings. Recent research has unveiled the vulnerability of DNNs to backdoor attacks, in which malicious actors manipulate the system by embedding specific triggers within the training data. In this paper, we propose a tailored camouflaged backdoor attack method designed for pedestrian detection in autonomous driving systems. Our approach begins with the construction of a set of trigger-embedded images. Subsequently, we employ an image scaling function to seamlessly integrate these trigger-embedded images into the original benign images, thereby creating potentially poisoned training images. Importantly, these potentially poisoned images exhibit minimal discernible differences from the original benign images and are virtually imperceptible to the human eye. We then strategically activate these concealed backdoors in specific scenarios, causing the pedestrian detection models to make incorrect judgments. Our study demonstrates that once our attack successfully embeds the backdoor into the target model, it can deceive the model into failing to detect any pedestrians marked with our trigger patterns. Extensive evaluations conducted on a publicly available pedestrian detection dataset confirm the effectiveness and stealthiness of our camouflaged backdoor attacks.
更多查看译文
关键词
autonomous driving,pedestrian detection,backdoor attacks,image scaling
AI 理解论文
溯源树
样例
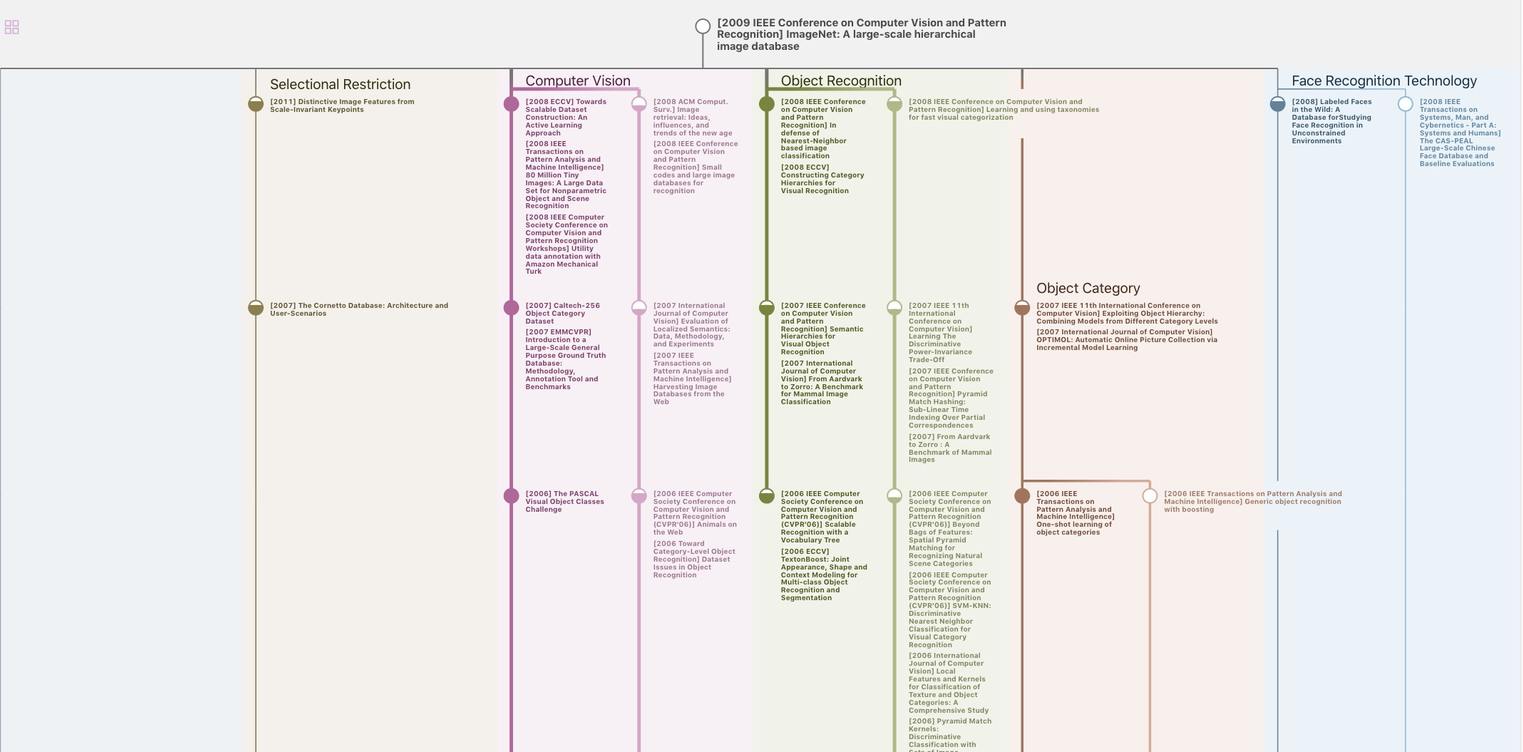
生成溯源树,研究论文发展脉络
Chat Paper
正在生成论文摘要