Revisiting Hard Negative Mining in Contrastive Learning for Visual Understanding
ELECTRONICS(2023)
Key words
contrastive learning,hard negative mining,Image–Text Retrieval,Temporal Action Localization,visual understanding
AI Read Science
Must-Reading Tree
Example
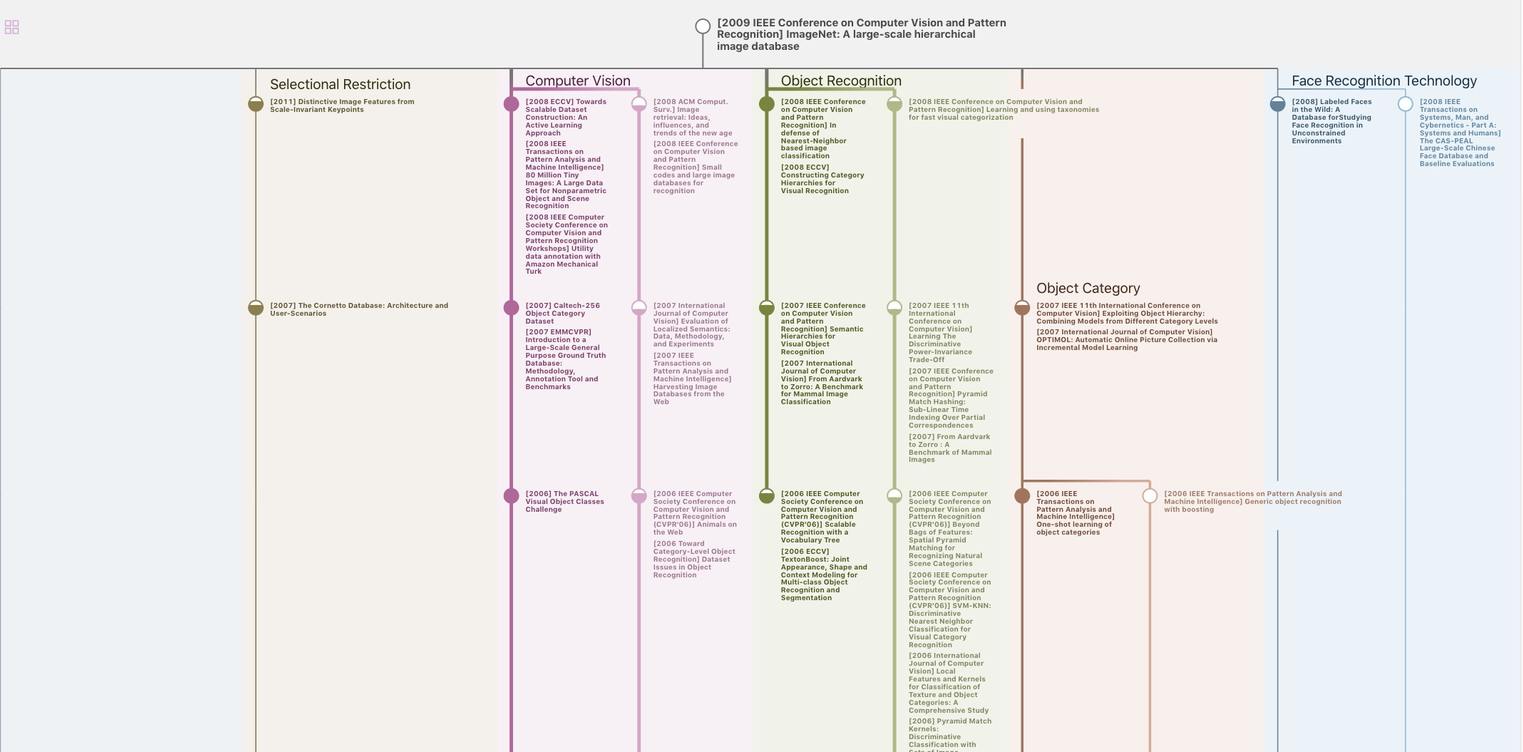
Generate MRT to find the research sequence of this paper
Chat Paper
Summary is being generated by the instructions you defined