Kibria-Lukman-Type Estimator for Regularization and Variable Selection with Application to Cancer Data
MATHEMATICS(2023)
摘要
Following the idea presented with regard to the elastic-net and Liu-LASSO estimators, we proposed a new penalized estimator based on the Kibria-Lukman estimator with L1-norms to perform both regularization and variable selection. We defined the coordinate descent algorithm for the new estimator and compared its performance with those of some existing machine learning techniques, such as the least absolute shrinkage and selection operator (LASSO), the elastic-net, Liu-LASSO, the GO estimator and the ridge estimator, through simulation studies and real-life applications in terms of test mean squared error (TMSE), coefficient mean squared error (beta MSE), false-positive (FP) coefficients and false-negative (FN) coefficients. Our results revealed that the new penalized estimator performs well for both the simulated low- and high-dimensional data in simulations. Also, the two real-life results show that the new method predicts the target variable better than the existing ones using the test RMSE metric.
更多查看译文
关键词
regularization,variable selection,elastic-net,LASSO,ridge estimator,Liu-LASSO
AI 理解论文
溯源树
样例
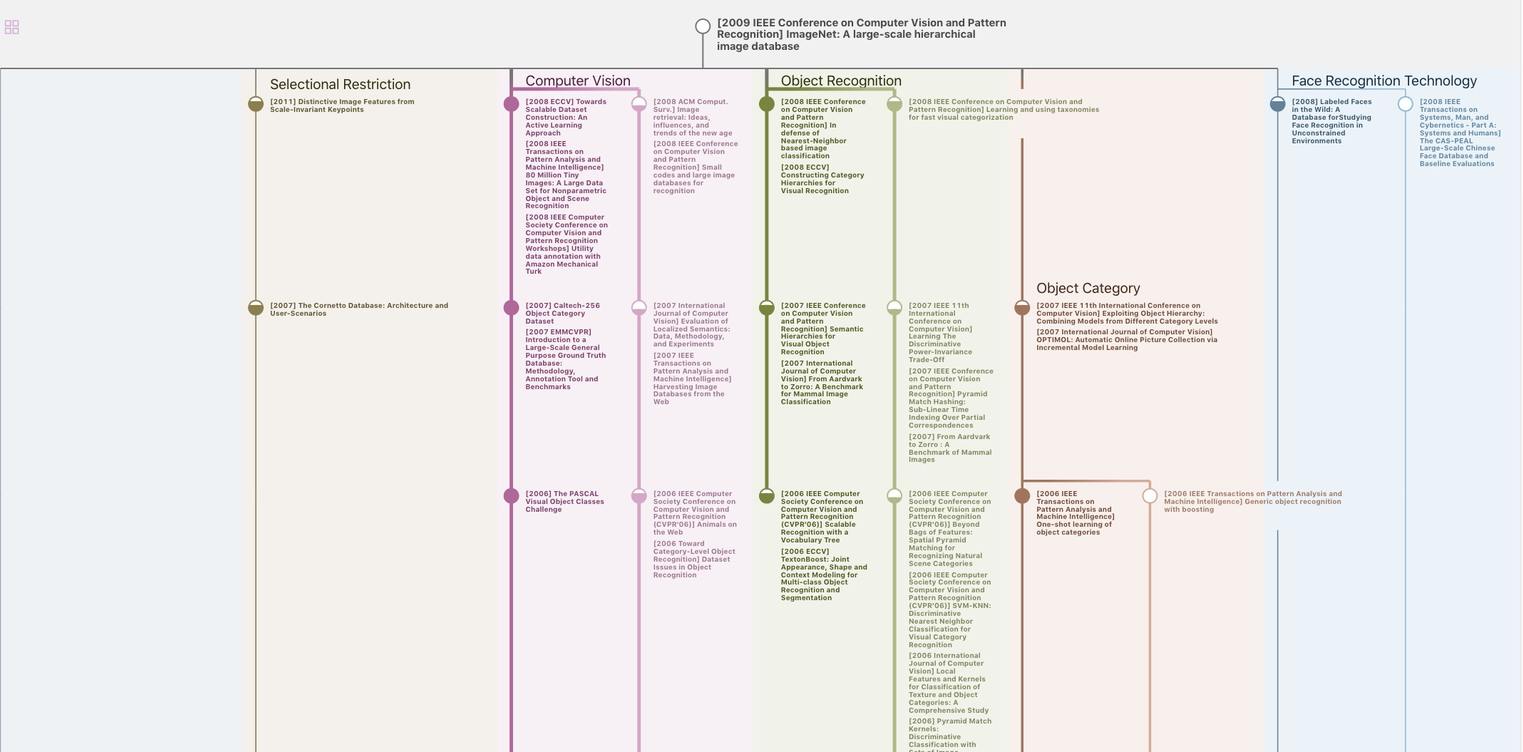
生成溯源树,研究论文发展脉络
Chat Paper
正在生成论文摘要