Improving Indoor Pedestrian Dead Reckoning for Smartphones under Magnetic Interference Using Deep Learning
SENSORS(2023)
摘要
As micro-electro-mechanical systems (MEMS) technology continues its rapid ascent, a growing array of smart devices are integrating lightweight, compact, and cost-efficient magnetometers and inertial sensors, paving the way for advanced human motion analysis. However, sensors housed within smartphones frequently grapple with the detrimental effects of magnetic interference on heading estimation, resulting in diminished accuracy. To counteract this challenge, this study introduces a method that synergistically employs convolutional neural networks (CNNs) and support vector machines (SVMs) for adept interference detection. Utilizing a CNN, we automatically extract profound features from single-step pedestrian motion data that are then channeled into an SVM for interference detection. Based on these insights, we formulate heading estimation strategies aptly suited for scenarios both devoid of and subjected to magnetic interference. Empirical assessments underscore our method's prowess, boasting an impressive interference detection accuracy of 99.38%. In indoor environments influenced by such magnetic disturbances, evaluations conducted along square and equilateral triangle trajectories revealed single-step heading absolute error averages of 2.1891 degrees and 1.5805 degrees, with positioning errors averaging 0.7565 m and 0.3856 m, respectively. These results lucidly attest to the robustness of our proposed approach in enhancing indoor pedestrian positioning accuracy in the face of magnetic interferences.
更多查看译文
关键词
magnetic interference,pedestrian dead reckoning,indoor positioning,convolutional neural network,support vector machine,unscented Kalman filter
AI 理解论文
溯源树
样例
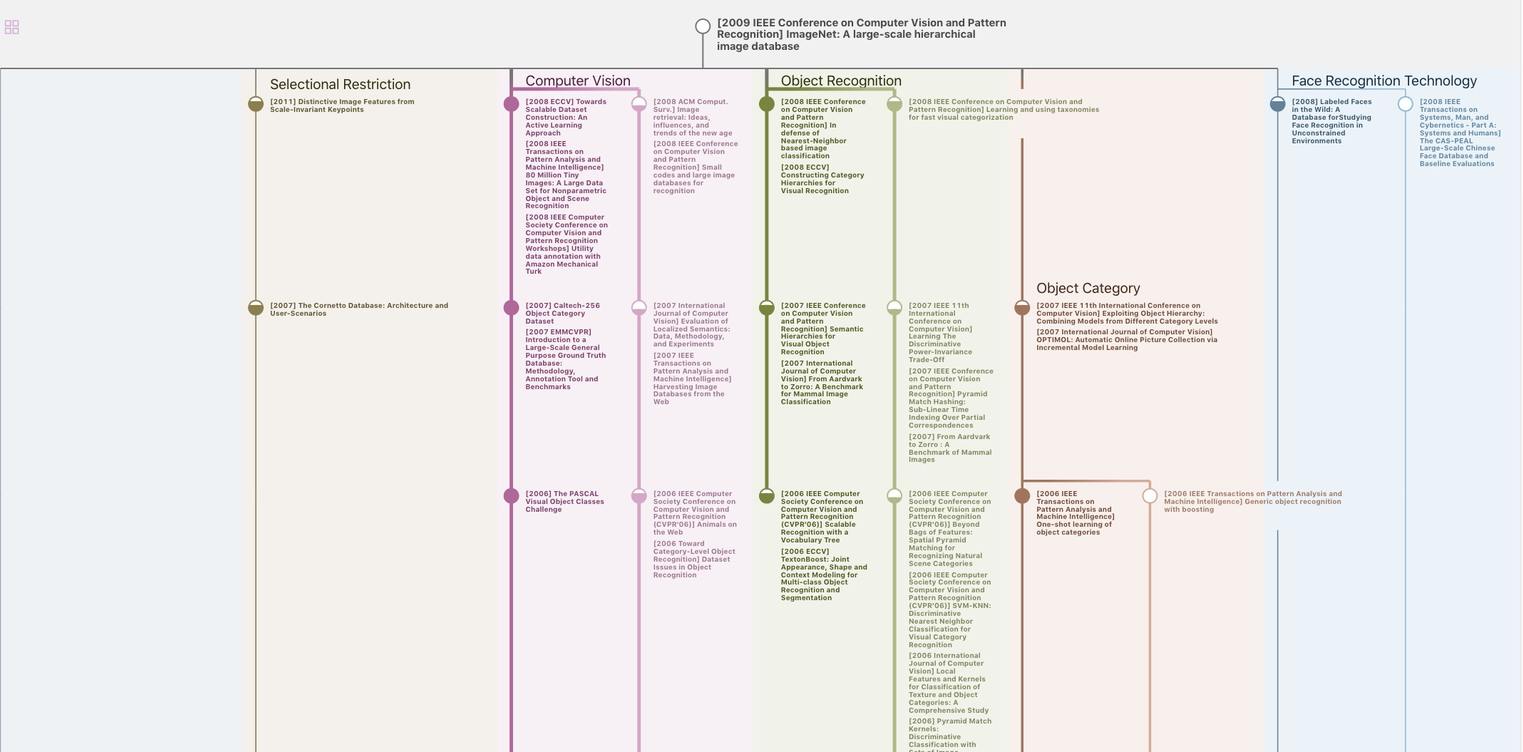
生成溯源树,研究论文发展脉络
Chat Paper
正在生成论文摘要