Metal Surface Defect Detection Based on a Transformer with Multi-Scale Mask Feature Fusion
SENSORS(2023)
摘要
In the production process of metal industrial products, the deficiencies and limitations of existing technologies and working conditions can have adverse effects on the quality of the final products, making surface defect detection particularly crucial. However, collecting a sufficient number of samples of defective products can be challenging. Therefore, treating surface defect detection as a semi-supervised problem is appropriate. In this paper, we propose a method based on a Transformer with pruned and merged multi-scale masked feature fusion. This method learns the semantic context from normal samples. We incorporate the Vision Transformer (ViT) into a generative adversarial network to jointly learn the generation in the high-dimensional image space and the inference in the latent space. We use an encoder-decoder neural network with long skip connections to capture information between shallow and deep layers. During training and testing, we design block masks of different scales to obtain rich semantic context information. Additionally, we introduce token merging (ToMe) into the ViT to improve the training speed of the model without affecting the training results. In this paper, we focus on the problems of rust, scratches, and other defects on the metal surface. We conduct various experiments on five metal industrial product datasets and the MVTec AD dataset to demonstrate the superiority of our method.
更多查看译文
关键词
defect detection,vision transformer,multi-scale feature fusion,pruning and merging
AI 理解论文
溯源树
样例
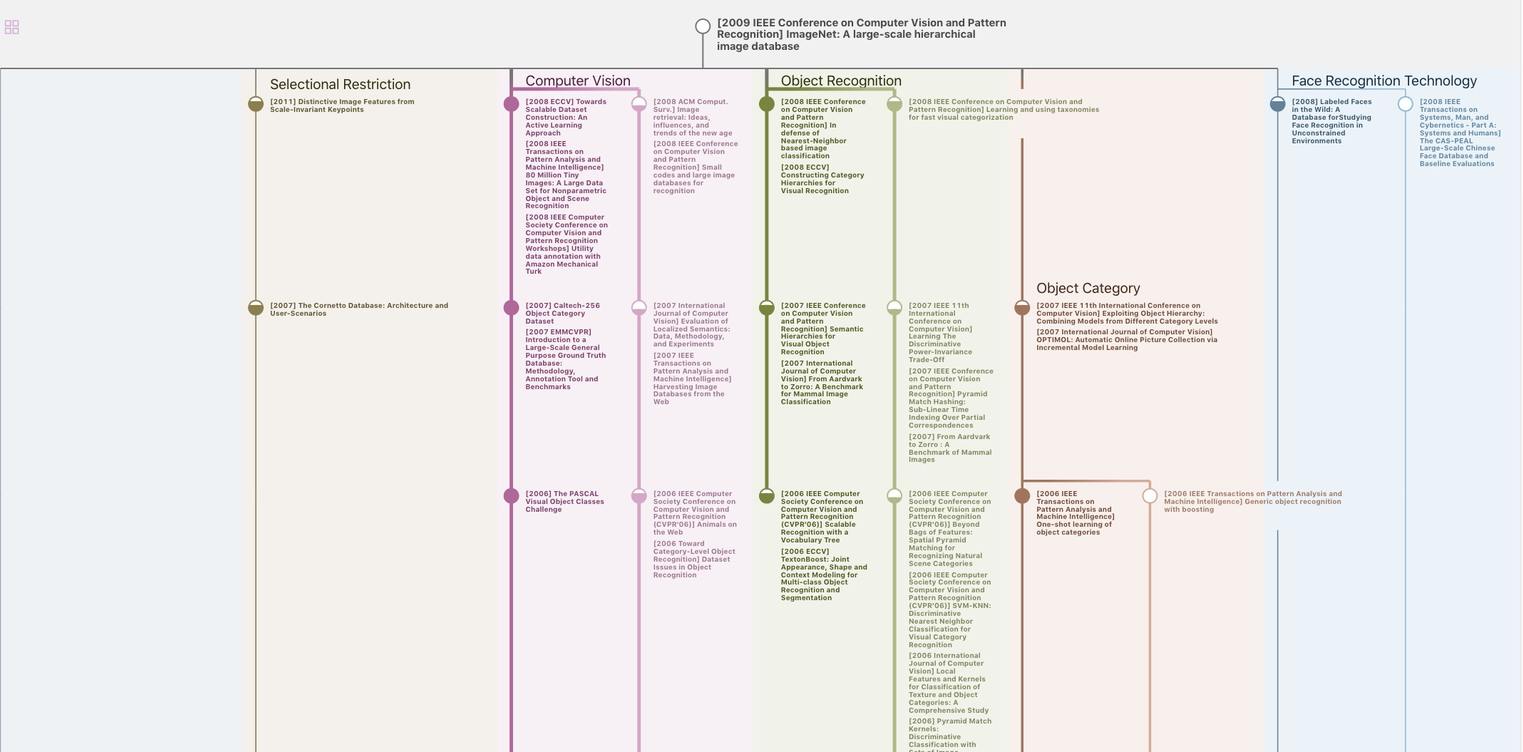
生成溯源树,研究论文发展脉络
Chat Paper
正在生成论文摘要