Robust Large-Scale Collaborative Localization Based on Semantic Submaps With Extreme Outliers
IEEE-ASME TRANSACTIONS ON MECHATRONICS(2023)
摘要
Filtering out outlier data associations between local maps can improve the robustness and accuracy of multirobot localization. When the overlap is low and the field of view difference is large, it is likely to produce outlier data associations between local maps, which will reduce the matching accuracy and even lead to the failure of collaborative localization. To remove outlier data associations between local maps and to enhance localization accuracy, this article proposes a novel outdoor large-scale robust collaborative localization algorithm in the presence of extreme outliers. The key novelty of this work is the designing of a mixture probability model and the hierarchical expectation maximization (H-EM) algorithm to identify outliers and improve localization performance. For every loop closure between submaps, the inlier probability is calculated to identify outlier loop closures by considering geometric distances, semantic consistency, and spatial consistency. Subsequently, the H-EM algorithm is utilized to exclude outlier loop closures and outlier point pairs within inlier loop closures, thereby relieving the adverse effect of outliers on localization accuracy. The proposed algorithm is validated on public datasets and compared with state-of-the-art methods, demonstrating significant improvement in localization accuracy and robustness.
更多查看译文
关键词
Collaborative localization,expectation maximization (EM),extreme outliers
AI 理解论文
溯源树
样例
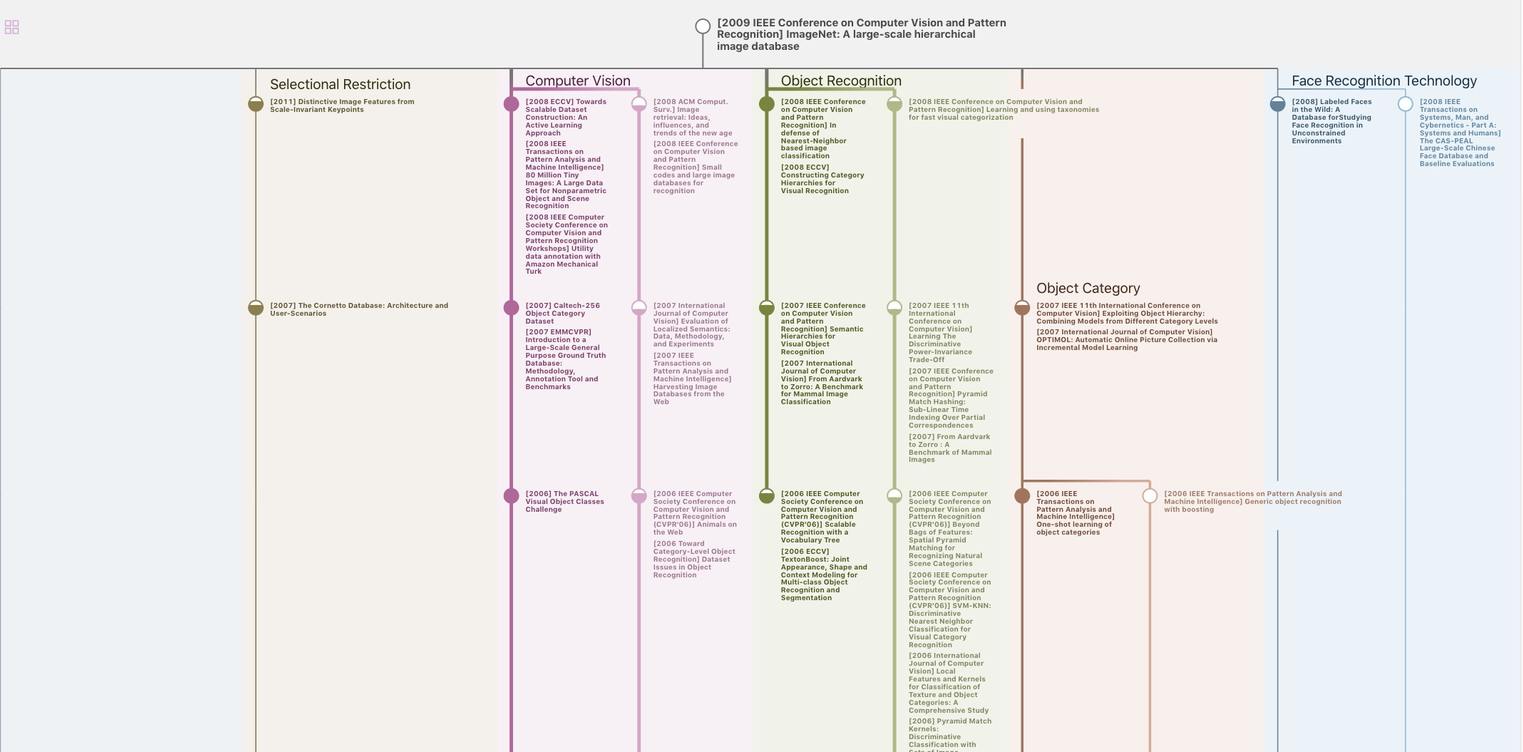
生成溯源树,研究论文发展脉络
Chat Paper
正在生成论文摘要