Sharp majorization-type cluster robust bounds for block filters and eigensolvers
SIAM JOURNAL ON MATRIX ANALYSIS AND APPLICATIONS(2023)
摘要
Convergence analysis of block iterative solvers for Hermitian eigenvalue problems and closely related research on properties of matrix-based signal filters are challenging and are attracting increased attention due to their recent applications in spectral data clustering and graph-based signal processing. We combine majorization-based techniques pioneered for investigating the Rayleigh-Ritz method in [A. V. Knyazev and M. E. Argentati, SIAM J. Matrix Anal. Appl., 31 (2010), pp. 1521-1537] with tools of classical analysis of the block power method by Rutishauser [Numer. Math., 13 (1969), pp. 4-13] to derive sharp convergence rate bounds of abstract block iterations, wherein tuples of tangents of principal angles or relative errors of Ritz values are bounded using majorization in terms of arranged partial sums and tuples of convergence factors. Our novel bounds are robust in the presence of clusters of eigenvalues, improve previous results, and are applicable to most known block iterative solvers and matrix-based filters, e.g., to block power, Chebyshev, and Lanczos methods combined with polynomial filtering. The sharpness of our bounds is fundamental, implying that the bounds cannot be improved without further assumptions.
更多查看译文
关键词
majorization,principal angles,Ritz values,subspace eigensolvers,cluster robustness,block Lanczos method
AI 理解论文
溯源树
样例
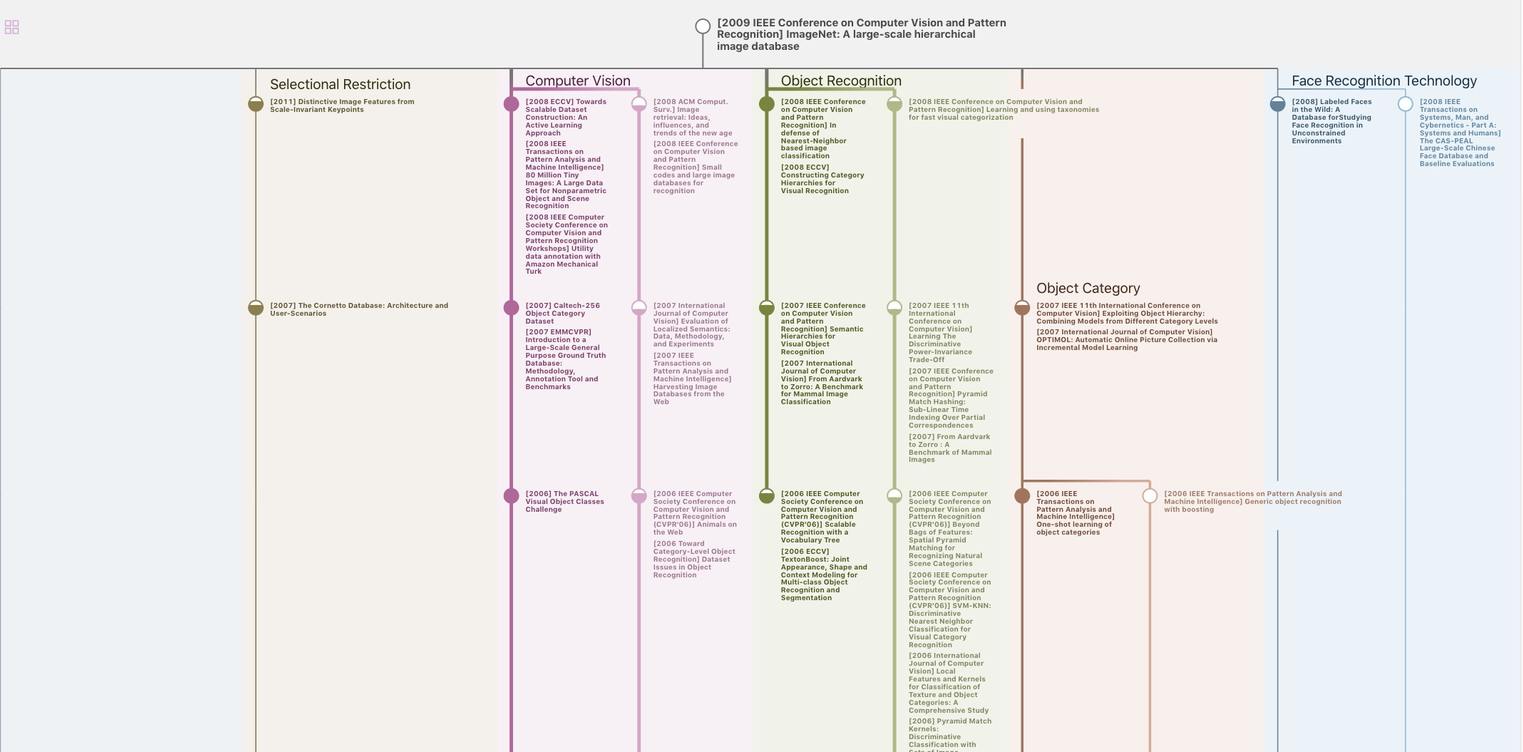
生成溯源树,研究论文发展脉络
Chat Paper
正在生成论文摘要