Identification of a Relapse Signature in t(8;21) Pediatric AML
BLOOD(2023)
摘要
Pediatric acute myeloid leukemia (AML) is a malignancy of the blood in which myeloid progenitor cells are interrupted in their normal course of development and proliferate out of control. Recent studies such as the TARGET initiative, have revealed pediatric AML to be driven by relatively few molecular abnormalities when compared to adult AML. Most frequently occurring in genes directly involved in or regulating transcription. These molecular aberrations are drivers of disease, inform patient risk stratification and are transcriptomically unique from one another. Of these events, RUNX1-RUNX1T1 is the most common, evident in approximately 15% of pediatric AML cases. While RUNX1-RUNX1T1 is a positive outcome prognosticator, 20% of these patients go on to relapse with significantly poorer outcomes. Our objective was to identify a relapse signature that could be identified at the time of diagnosis so that alternative therapeutic strategies can be employed. Here we report the investigation of RNAseq data from 3,022 pediatric AML patient samples and identification of a transcriptomic relapse-signature (TRS) via differential expression analysis, supervised clustering and machine learning modelling. We performed differential gene expression analysis comparing diagnostic t(8;21) patient samples for those who relapsed (R) (n=38)vs. those who did not relapse (NR) (n=111). Analysis was performed using DESeq2 in R 4.3.0. P-values were adjusted with Benjamini-Hochberg correction to control for false discovery rate a significance threshold for padj of < 0.05 was applied. A list of 462 differentially genes was returned. Hierarchical clustering of the 462 differentially expressed genes revealed 4 patient clusters, each with a distinct transcriptomic signature ( Fig 1A). K-means (n=4) also returned similar results to the hierarchical clustering. Kaplan-Meier analysis of the 4 clusters showed a significant difference in patient outcomes both in terms of overall survival (P < 0.001) and event-free survival (P < 0.05) ( Fig 1B). In marked contrast to clusters 1-3, cluster 4 was highly enriched in patients who relapsed at a rate of 86%. Clusters 1, 2 and 3 had relapse rates of 36, 29 and 38 percent respectively. To identify the most relevant genes that could serve as a TRS, we trained a random forest (RF) model using transcript per million (TPM) transformed and length normalized counts for each of the 462 differentially expressed genes at the time of diagnosis between the R and NR cohort; the response variable was patient relapse outcome. Data was split into 70% training and 30% testing cohorts. As the small size of our cohort was a potentially limiting factor, we utilized 5-fold cross validation in the training of our model. Recursive feature selection was applied to the model and was iteratively trained using subsets of features (predictor genes) ranging from 1 to 100. Features were then recursively sorted by importance and an optimal number of 30 predictors were chosen. Application of our model to the testing dataset of 45 patients (R = 12, NR = 33) allowed us to accurately predict the outcome in 40 of 45 patients. This model accurately predicted 33 of 33 NR and 7 of 12 R patients. This model was both specific and sensitive for NR and specific to R but lacked sensitivity as we were only able to identify 58% of relapse patients. Finally, this 30-gene list was then compared to survival analysis on a by-gene basis which yielded a final panel of 16 genes which we call our TRS. These genes were; PRDM8, RARG, CSF1, PDE4B, CCL3, NAB1, WHRN, SGSM2, LPIN3, CHARLIE1B, SATB1, ZNF441, ZNF521, AL451123.1, TSPYL4 and B3GNT5. The RF model was re-trained on this smaller panel and re-tested with similar results. By applying differential expression analysis, K-means clustering and machine learning to RNAseq data from a cohort of 149 pediatric AML patients we successfully identified a relapse-signature using a panel of 16 genes evident at the time of diagnosis and prognostic of patient outcomes. Future efforts will focus on increasing the sensitivity of our model to relapse prediction and further validation on other pediatric t(8;21) cohorts.
更多查看译文
AI 理解论文
溯源树
样例
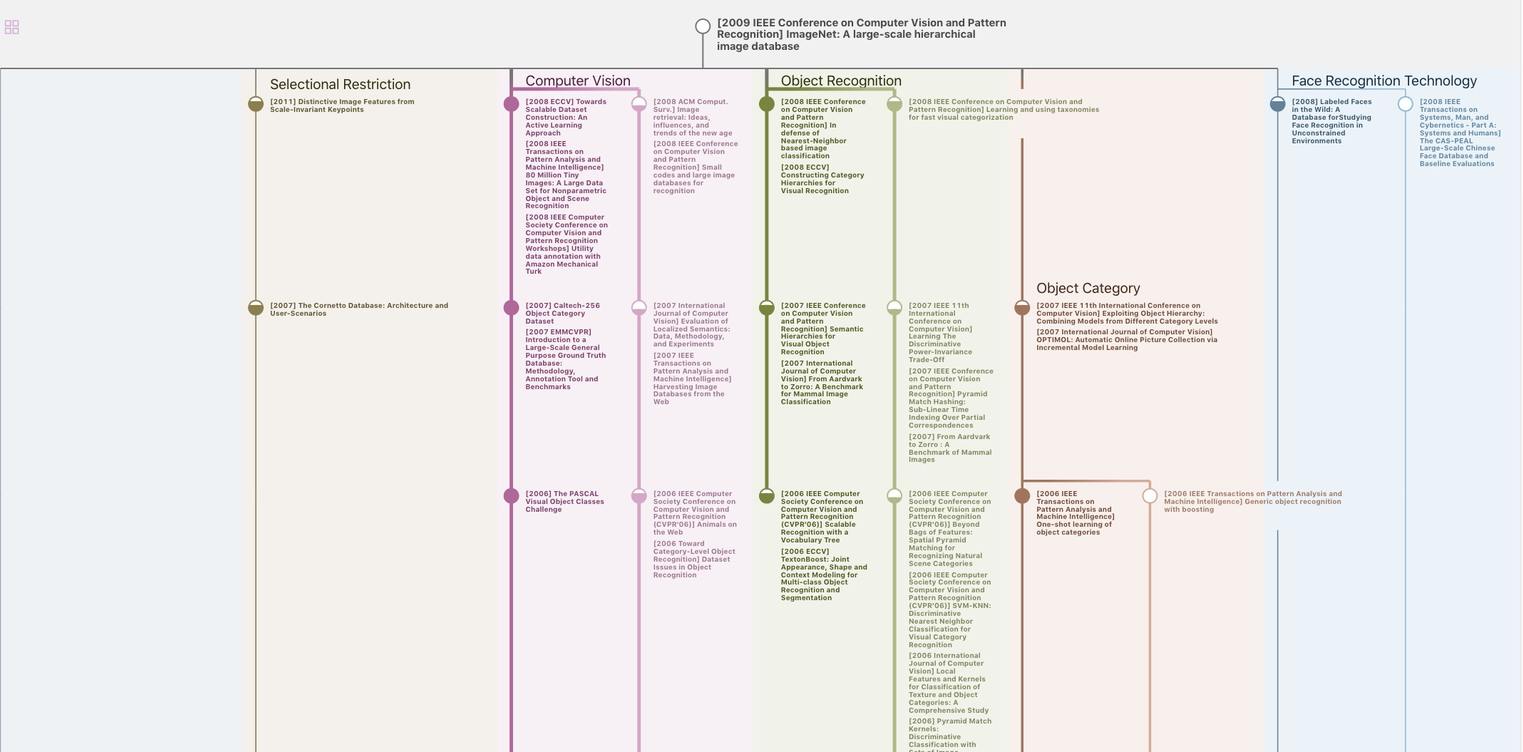
生成溯源树,研究论文发展脉络
Chat Paper
正在生成论文摘要