FAMM: Facial Muscle Motions for Detecting Compressed Deepfake Videos Over Social Networks
IEEE TRANSACTIONS ON CIRCUITS AND SYSTEMS FOR VIDEO TECHNOLOGY(2023)
摘要
As a face manipulation technique, the misuse of Deepfakes poses potential threats to the state, society, and individuals. Several countermeasures have been proposed to reduce the negative effects produced by Deepfakes. Current detection methods achieve satisfactory performance in dealing with uncompressed videos. However, videos are generally compressed when spread over social networks because of limited bandwidth and storage space, which generates compression artifacts and the detection performance inevitably decreases. Hence, how to effectively identify compressed Deepfake videos over social networks becomes a significant problem in video forensics. In this paper, we propose a facial-muscle-motions-based (FAMM) framework to solve the problem of compressed Deepfake video detection. Specifically, we first locate faces from consecutive frames and extract landmarks from the face images. Then, continuous facial landmarks are utilized to construct facial muscle motion features by modeling the five sensory and face regions. Finally, we fuse the diverse forensic knowledge using Dempster-Shafer theory and provide the final detection results. Furthermore, we demonstrate the effectiveness of FAMM through analyzing mutual information, compression procedure, and facial landmarks for compressed Deepfake videos. Theoretical analyses illustrate that compression does not affect facial muscle motion feature construction and the differences in designed features exist between the real and Deepfake videos. Extensive experimental results conclude that the proposed method outperforms the state-of-the-art methods in detecting compressed Deepfake videos. More importantly, FAMM achieves comparable detection performance on compressed videos that are over real-world social networks.
更多查看译文
关键词
Multimedia forensics,compressed deepfake videos,facial muscle movements,social networks
AI 理解论文
溯源树
样例
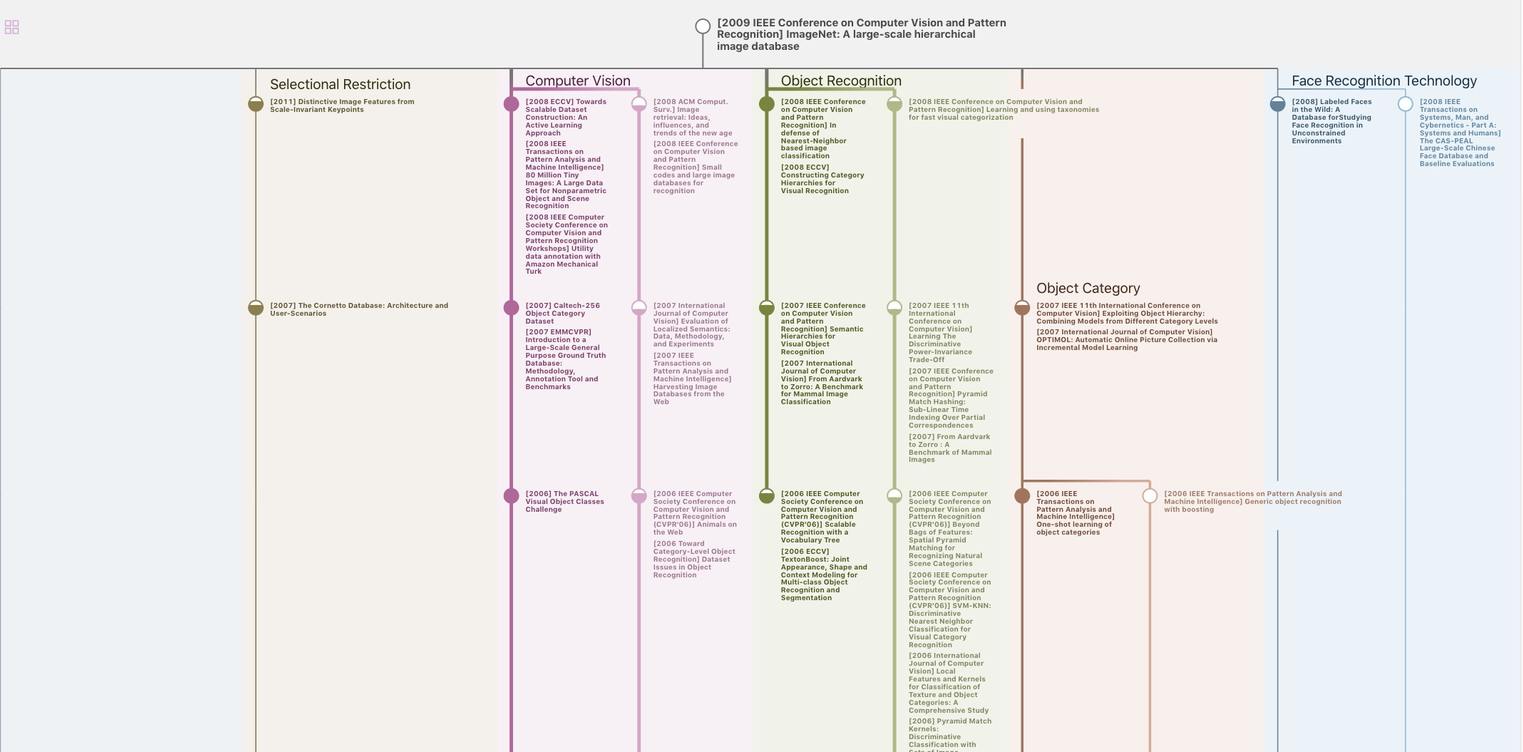
生成溯源树,研究论文发展脉络
Chat Paper
正在生成论文摘要