CityTrans: Domain-Adversarial Training With Knowledge Transfer for Spatio-Temporal Prediction Across Cities
IEEE TRANSACTIONS ON KNOWLEDGE AND DATA ENGINEERING(2024)
摘要
As the spatio-temporal data of a city is not always available, insufficient data would lead to poor performance in some urban prediction tasks. Existing works utilize transfer learning to solve the data scarcity problem, but they ignore the differences in data distributions across cities, which leads to the ineffectiveness of knowledge transfer. In this paper, we propose a domain adversarial model with knowledge transfer for spatio-temporal prediction across cities, entitled CityTrans. Specifically, 1) the self-adaptive spatio-temporal knowledge (namely ST-Knowledge) is mined, to learn the latent spatial and temporal patterns among cities; 2) the domain-adversarial training strategy is introduced to enhance domain invariance; 3) a knowledge attention mechanism is proposed to extract the transferable information from the ST-Knowledge. Note that our CityTrans is an end-to-end domain adversarial spatio-temporal network without two-stage training (i.e., pre-training and fine-tuning). Finally, we conduct extensive experiments on two spatio-temporal prediction tasks: traffic (flow and speed) prediction, and air quality prediction. Experimental results demonstrate that CityTrans outperforms state-of-the-art models on all tasks by a significant margin.
更多查看译文
关键词
Urban computing,graph neural network,transfer learning,spatio-temporal prediction
AI 理解论文
溯源树
样例
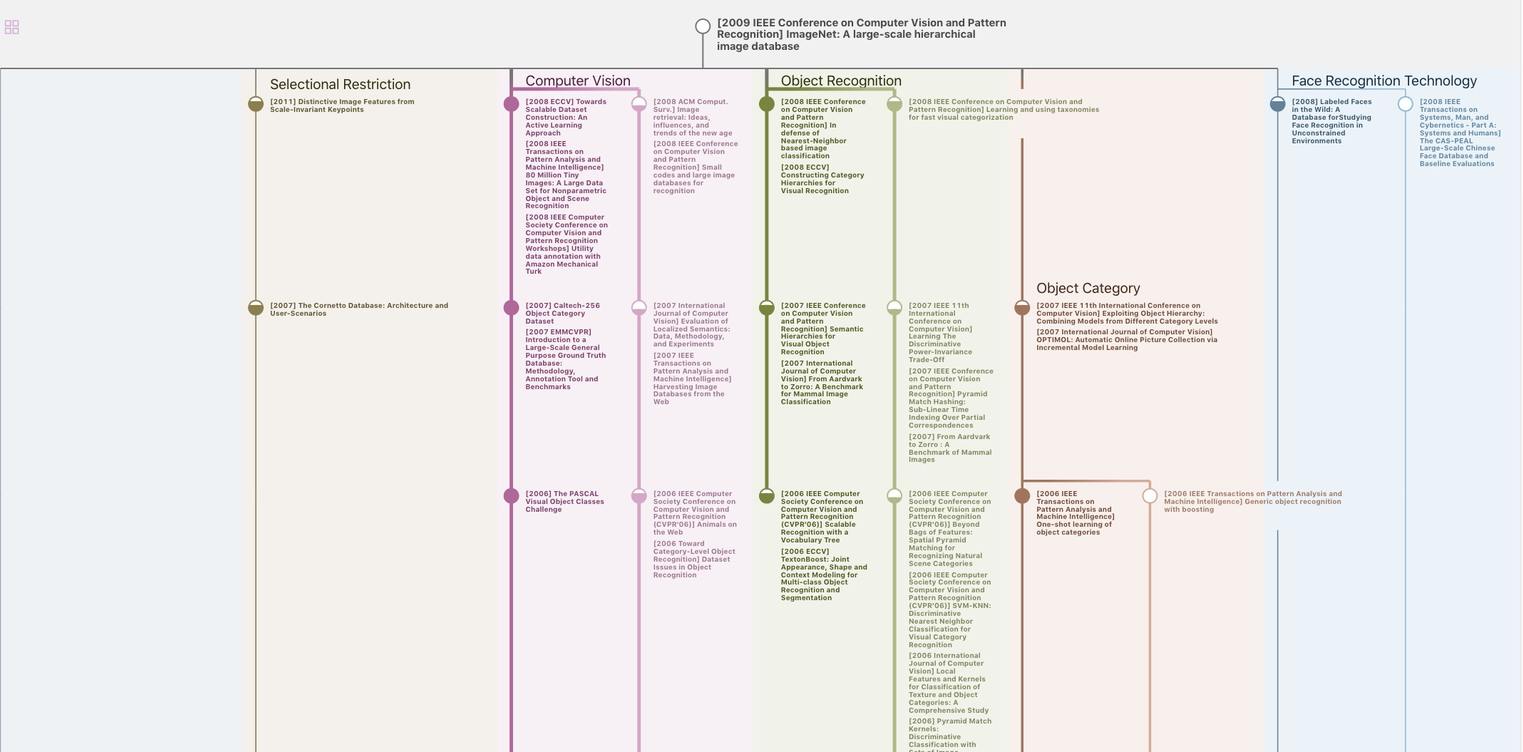
生成溯源树,研究论文发展脉络
Chat Paper
正在生成论文摘要