Machine learning classification of attention deficit hyperactivity disorder using genomic context informed genotype data and within-model ancestry adjustment
European Neuropsychopharmacology(2023)
Abstract
Classification models using genetic data in ADHD do not perform as well as expected given the high heritability of the disorder. One possibility in improving performance is better using the accumulated knowledge about the genome to help machine learning models find patterns among the disorder's heterogeneous and complex genetic architecture. In a study of 2,455 people with ADHD and 8,432 without ADHD across 9 cohorts, we collected and calculated genomic annotations related to the functional and structural qualities of each genotyped single nucleotide polymorphism. We used this genomic context alongside genotype data as input into a convolutional neural network models aiming to predict ADHD diagnosis. We implemented an adversarial ancestry task, which forces the model to unlearn features that would otherwise be helpful in predicting ancestry, to test within-model ancestry adjustment. We used additional adversarial tasks to test hypotheses on the differences between models with and without genomic context. Using the same methods in type 2 diabetes, a neural network using genotype data (AUC: 0.66) and a convolutional neural network using context informed genotype data (AUC: 0.65) both outperformed a polygenic risk score approach (AUC: 0.57). Adversarial ancestry tasks effectively eliminated the predictability of ancestry without changing model performance. Analyses are underway in ADHD and will be compared to previous results in the same dataset where a gene set polygenic risk score model had an AUC of 0.72, a traditional PRS model had an AUC of 0.62, and a PRS-CS model had an AUC of 0.66. Our current results suggest that context informed genotype models can find novel risk features. They also suggest that enriching machine learning models with annotation rich genotype data is useful in improving classification performance. Adversarial ancestry adjustment can effectively adjust for ancestry within each model and report any remaining ability of the model to use ancestry as a predictor.
MoreTranslated text
Key words
attention deficit hyperactivity disorder,deficit hyperactivity disorder,genotype,t51,within-model
AI Read Science
Must-Reading Tree
Example
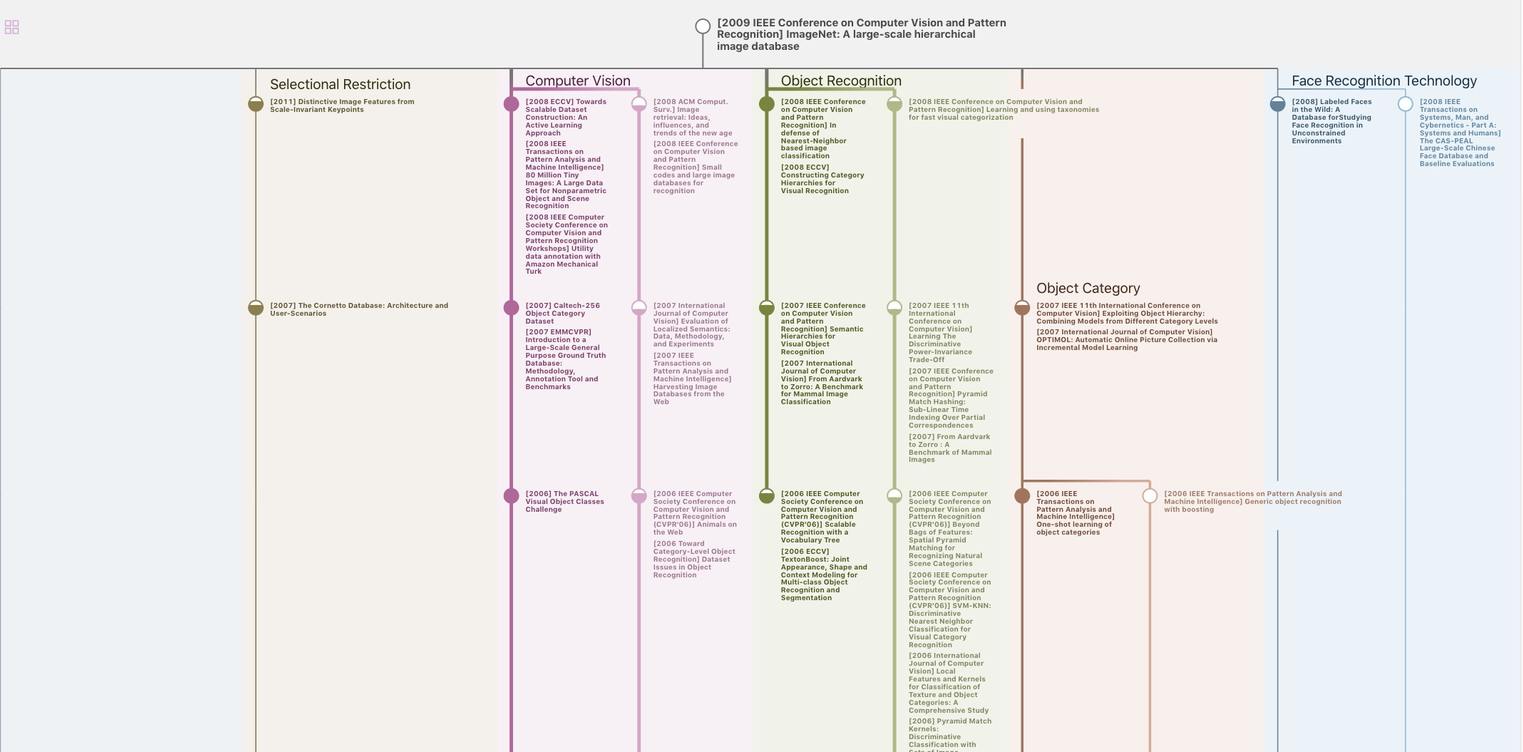
Generate MRT to find the research sequence of this paper
Chat Paper
Summary is being generated by the instructions you defined