Resilient Machine Learning Based Semantic-Aware MEC Networks for Sustainable Next-G Consumer Electronics
IEEE Transactions on Consumer Electronics(2023)
摘要
In this paper, we investigate a semantic-aware mobile edge computing (MEC) network for sustainable next-G consumer electronics, which leverages advanced semantic communication technology to overcome the limitations of available bandwidth and thereby improve communication efficiency. For this network, consumer electronic devices can offload the semantic information extracted from the task instead of transmitting the whole task in the conventional MEC networks, where the latency and energy consumption can be significantly reduced through proper semantic encoding, offloading, and bandwidth and computation allocation decisions. However, the non-convexity of the issue makes it difficult to obtain the optimal decision. To address this issue, a two-level optimization framework is proposed. Specifically, in the upper-level optimization, a resilient deep reinforcement learning (DRL) approach is utilized to enable adaptive offloading and semantic encoding decisions within a dynamic network. In the lower-level optimization, we design three criteria for allocating bandwidth and computation resources by carefully considering the trade-off between computational complexity and implementation efficiency. Finally, extensive simulations are conducted to validate the effectiveness of our proposed strategy. The findings in this paper can help reduce the energy consumption of consumer electronics, hence supporting the development of sustainable next-G consumer electronics.
更多查看译文
关键词
Mobile edge computing,semantic communication,resilient optimization,deep reinforcement learning
AI 理解论文
溯源树
样例
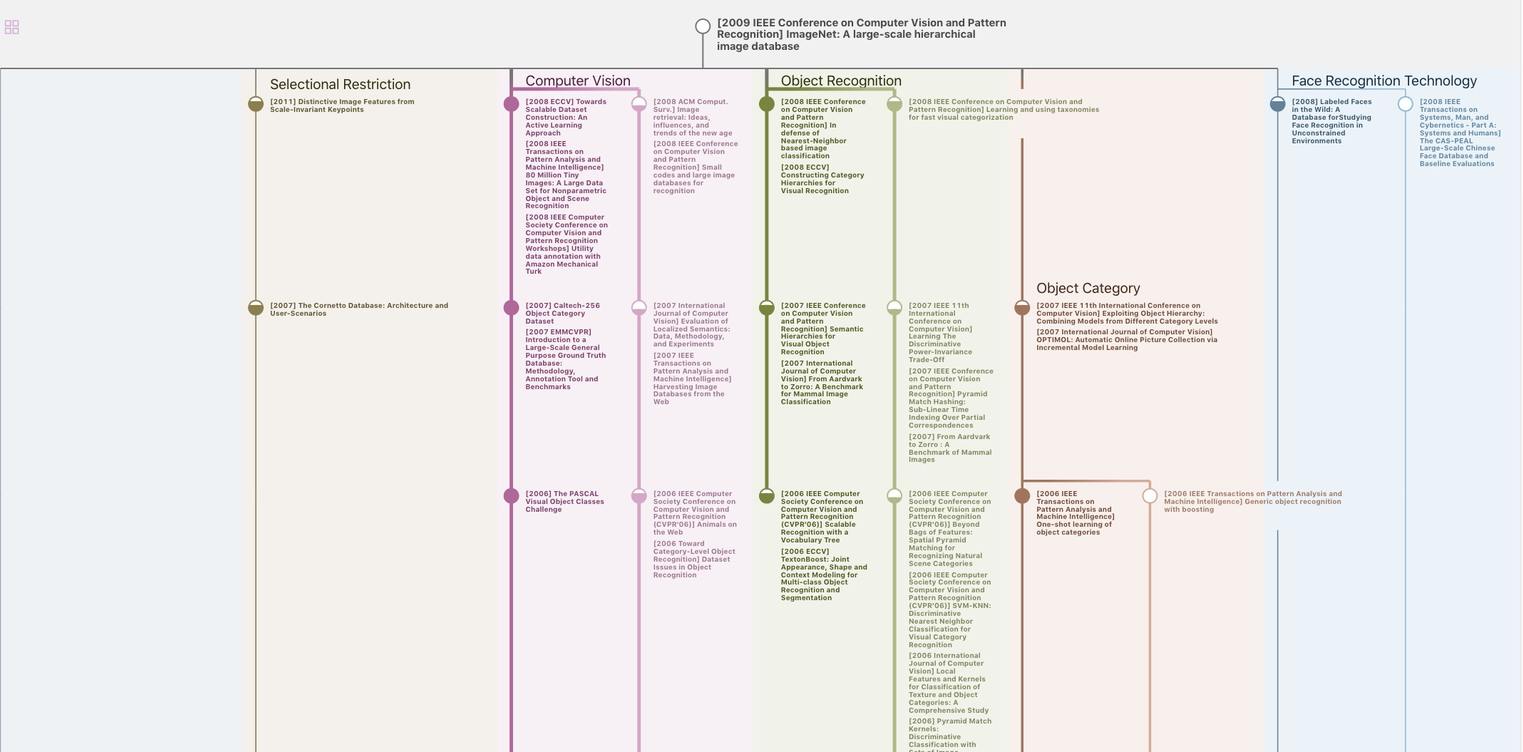
生成溯源树,研究论文发展脉络
Chat Paper
正在生成论文摘要