Continual Residual Reservoir Computing for Remaining Useful Life Prediction
IEEE TRANSACTIONS ON INDUSTRIAL INFORMATICS(2024)
摘要
In practical engineering applications, it is inevitable that production is often faced with different working conditions. Therefore, it is necessary to have a continual learning system, which can adapt to a sequence of tasks and keep learning over time. In this work, we propose a continual deep residual reservoir computing framework for the practical remaining useful life prediction task. Specifically, we propose a novel deep echo state network structure with residual blocks to effectively mitigate the performance degradation of the deep reservoir computing framework and reduce the difficulty of model training. Furthermore, the proposed framework is trained with the elastic weight consolidation method to alleviate the impact of catastrophic forgetting in continual learning systems. Extensive experiments are conducted with the FEMTO-ST bearing and high-intensity-radiated fields battery dataset. And the proposed framework is proven to be effective in multiple continual learning tasks compared with other state-of-the-art methods.
更多查看译文
关键词
Continual learning,remaining useful life (RUL) prediction,reservoir computing (RC)
AI 理解论文
溯源树
样例
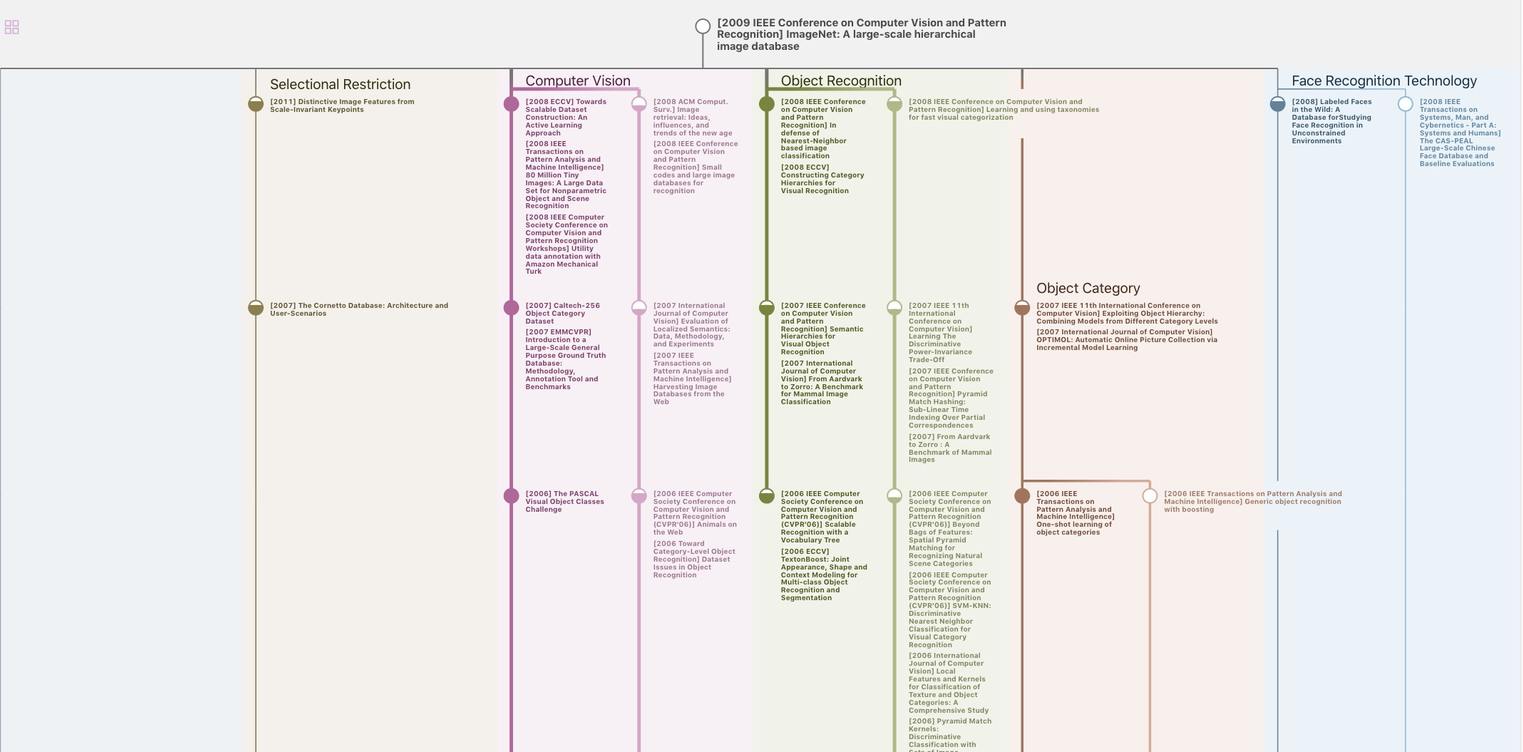
生成溯源树,研究论文发展脉络
Chat Paper
正在生成论文摘要