Parallel Guiding Sparse Auto-Encoder with Wasserstein Regularization for Efficient Classification
crossref(2023)
Abstract
In the era of big data, feature engineering has proved its efficiency and importance in dimensionality reduction and useful information extraction from original features. Feature engineering can be expressed as dimensionality reduction and is divided into two types of methods such as feature selection and feature extraction. Each method has its pros and cons. There are a lot of studies to combine these methods. Sparse autoencoder (SAE) is a representative deep feature learning method that combines feature selection with feature extraction. However, existing SAEs do not consider the feature importance during training. It causes extracting irrelevant information. In this paper, we propose a parallel guiding sparse autoencoder (PGSAE) to guide the information by two parallel guiding layers and sparsity constraints. The parallel guiding layers keep the main distribution using Wasserstein distance which is a metric of distribution difference, and it suppresses the leverage of guiding features to prevent overfitting. We perform our experiments using four datasets that have different dimensionality and number of samples. The proposed PGSAE method produces a better classification performance compared to other dimensionality reduction methods.
MoreTranslated text
AI Read Science
Must-Reading Tree
Example
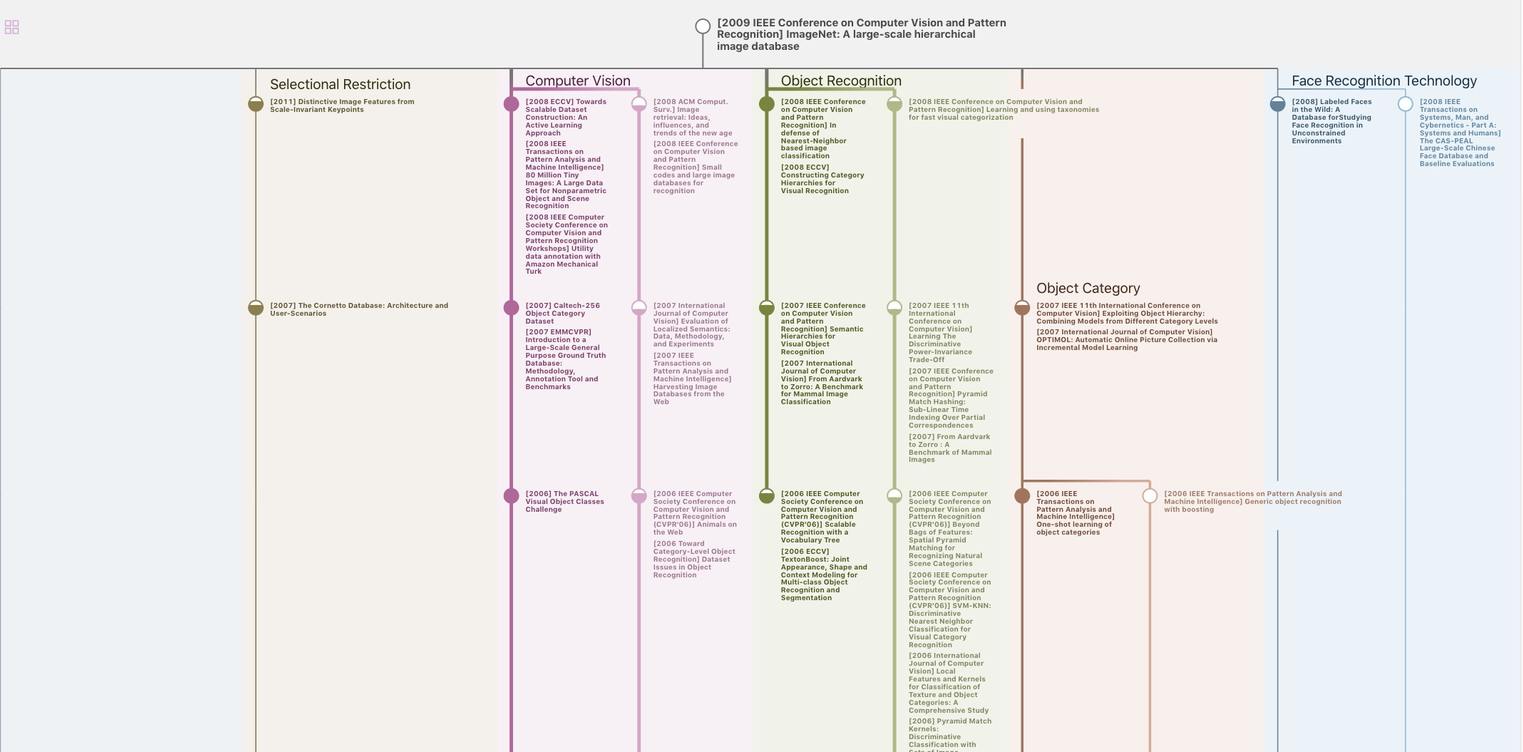
Generate MRT to find the research sequence of this paper
Chat Paper
Summary is being generated by the instructions you defined