Automated remote sleep monitoring needs machine learning with uncertainty quantification
crossref(2023)
Abstract
Abstract Wearable electroencephalography (EEG) devices emerge as a cost-effective and ergonomic alternative to gold standard polysomnography, paving the way for better health monitoring and sleep disorder screening. Machine learning allows to automate sleep stage classification, but trust and reliability issues have hampered its adoption in clinical applications. Estimating uncertainty is a crucial factor in enhancing reliability by identifying regions of heightened and diminished confidence. In this study, we investigated the utility of an uncertainty-centered machine learning pipeline, U-PASS, on sleep staging in a challenging real-world dataset of single-channel EEG and accelerometry collected with a wearable device from elderly sleep apnea patients. We demonstrated that U-PASS effectively limited the uncertainty at training time and communicated uncertainty to clinical experts at deployment time to improve the machine learning model's reliability. This effectively increased the 5-stage sleep scoring accuracy of a state-of-the-art machine learning model from 63.9% to 71.2% on our dataset. Remarkably, the machine learning approach outperformed the human expert in interpreting these wearable data. Manual review by sleep specialists, without specific training for sleep staging on wearable EEG, proved ineffective. The diagnostic capabilities of this automated remote monitoring system were also demonstrated, establishing a strong correlation between the predicted sleep features and the most important clinical marker for sleep apnea. In essence, an automated data processing pipeline enhanced with uncertainty estimation presents a promising avenue to unlock the full potential of machine learning in revolutionizing remote patient care.
MoreTranslated text
AI Read Science
Must-Reading Tree
Example
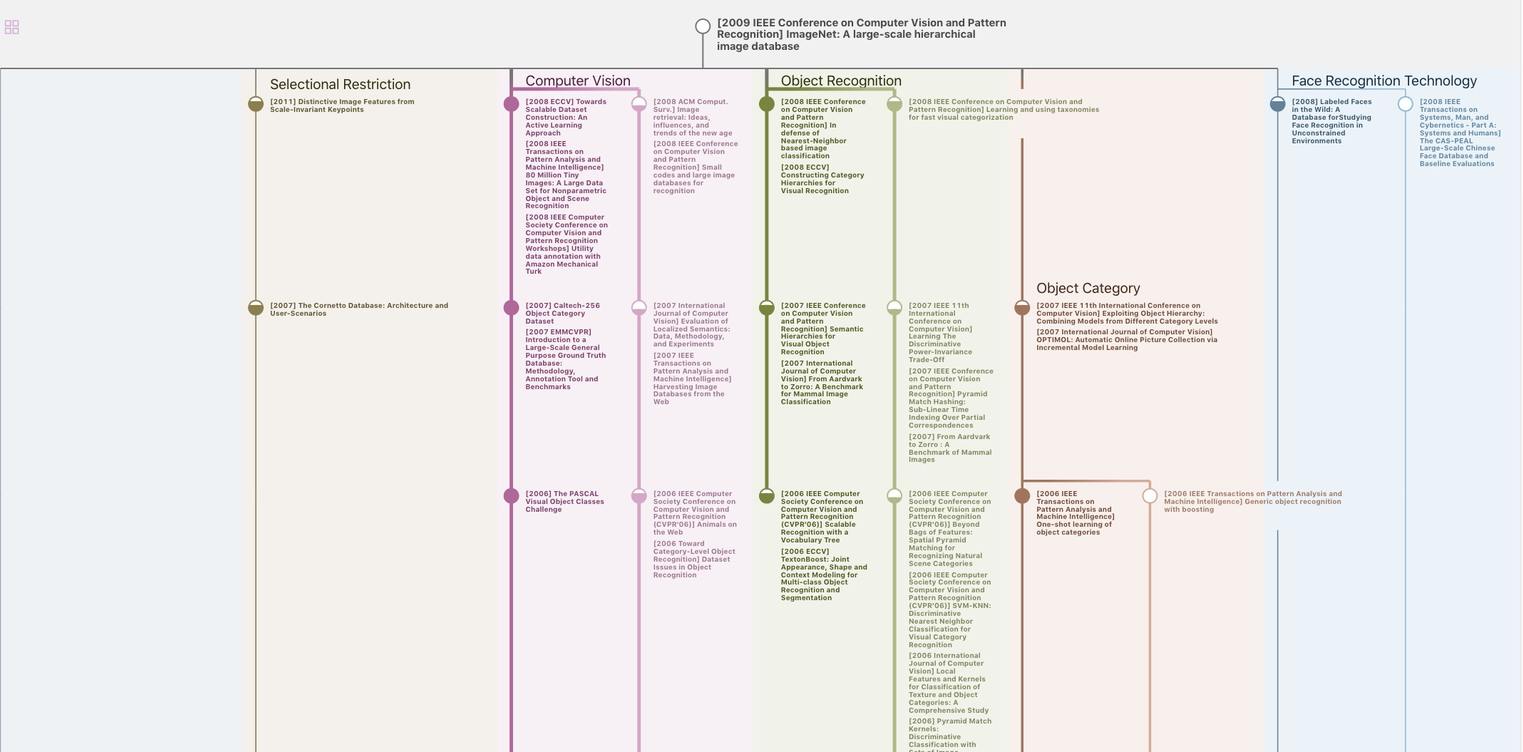
Generate MRT to find the research sequence of this paper
Chat Paper
Summary is being generated by the instructions you defined